Behind the Research: How Sony AI Researchers are Pioneering AI Models for Scientific Discovery
Scientific Discovery
September 14, 2024
The pace of scientific research is accelerating, with an exponential increase in published research articles each year. For instance, in 1980, approximately 500,000 scientific articles were published, while by 2020, that number had risen to over 2.5 million. Navigating this vast and ever-growing body of knowledge is a daunting task for researchers. As Isaac Asimov wisely stated, "Science gathers knowledge faster than society gathers wisdom."
Researchers at Sony AI, including Research Scientist, Uchenna Akujuobi, are building upon the existing research literature to develop innovative models that support the challenges that scientific discovery faces.
Akujuobi explains: “Scientific Discovery is one of our flagship projects, and our goal is to augment knowledge and creativity in complex scientific research with AI. We aim to create tools that support researchers to accelerate discoveries, for the benefit of humanity.”
One of the pressing challenges for researchers lies in how to keep up with their fields and, in order to do so, how to efficiently extract meaningful insights from the aforementioned ocean of data and knowledge. The purpose of AI models, like those developed by Sony AI, is not to occupy the human role within the scientific process, but to free researchers from some of the more tedious parts of their work. As Akujuobi put it, "We’re not trying to replace researchers—but to assist them in producing higher quality research in less time."
In this blog, we will explore two key contributions to scientific discovery from the Sony AI team THiGER, a temporal graph-based method for hypothesis generation, and SINERA/SINERAS, models that enhance Named Entity Recognition (NER).
Leveraging Temporal Graphs for Hypothesis Generation
Our first piece of research introduces THiGER, a novel approach using temporal graphs to predict as-of-yet-undiscovered relationships between entities in a research domain. The key differentiator to previous methods is THiGER’s greatly improved ability to capture the temporal evolution of connections between scientific concepts.
As Akujuobi explained, "Understanding the temporally evolving interactions between domain entities can provide valuable information for predicting the future development of entity relationships."
This temporal understanding is akin to a detective piecing together clues over time to solve a mystery. THiGER, much like a modern-day Sherlock Holmes, identifies potential future links between scientific concepts by analyzing how these relationships evolve. The project, which began with an idea from Sony AI COO Michael Spranger in collaboration with the Tokyo-based System Biology Institute, was born out of the need to develop systems that could help researchers manage the overwhelming volume of academic papers being published. "The number of publications is growing faster than a human researcher can keep up with," remarks Akujuobi.
THiGER’s hierarchical transformer-based architecture is designed to overcome the limitations of previous methods by modeling temporal relationships hierarchically. This allows the model to prioritize attention to the most informative regions of temporal evolution, improving the quality of predictions.
When asked about the challenges faced in ensuring that THiGER could generalize across different types of biomedical datasets, particularly those with sparse temporal data, Akujuobi explained, "There are two main ways we addressed that challenge. One is through the architecture of the model itself, and the other is through how we feed the data into the model, ensuring it’s not just randomized."
Akujuobi explained that the transformer model's architecture enables it to focus on the most relevant input, unlike recurrent models that may forget earlier information. By feeding data in gradually increasing temporal windows, starting with a 10-year period, the model's attention mechanism effectively captures the temporal evolution of the data. This approach ensures comprehensive training even with past information.
A key innovation in these models is the use of curriculum learning, where the models are trained incrementally, starting with simpler concepts and gradually tackling more complex ones.
Priyadarshini Kumari, Akujuobi’s joint first author on the article, developed a training strategy employing active curriculum learning by gradually introducing more difficult examples, imitating the way humans learn—from simple to complex concepts.
This approach allows the model to be trained on a diverse set of informative data with varying complexities, helping it generalize better across different datasets.
Another critical aspect of THiGER’s design is the evaluation metrics used to assess its performance. As Akujuobi noted, "The choice of evaluation metrics is crucial. When predicting positive versus negative connections, there is often an imbalance—many more negative samples than positive ones.
Using metrics like ‘accuracy’ could give a false sense of performance. For example, if a model predicts everything as negative, it might appear to perform well due to the high number of negatives. But that’s misleading. Metrics like F1 score and Average Precision give a more balanced view by focusing on both positive and negative predictions, providing a more accurate measure of the model's performance."
These metrics ensure that THiGER’s predictions are reliable and meaningful, which is essential for making valid scientific hypotheses.
Looking ahead, Akujuobi discussed the next steps for THiGER: "We’re expanding the model’s capabilities to not just predict connections but to understand the nature of those connections. For example, not just predicting that paracetamol is connected to headache relief, but also determining whether it cures or exacerbates the condition."
This forward-thinking approach highlights Sony AI’s commitment to enhancing the practical utility of AI in scientific research.
In the context of food computing, THiGER has already shown promise in predicting links between dietary habits and health outcomes, potentially leading to new dietary recommendations and food products that promote better health. This application is particularly relevant given the increasing prevalence of diet-related health conditions, such as obesity and type-2 diabetes.
For instance, the paper discusses a case study where THiGER was used to predict connections between oils like flaxseed and health conditions such as root caries and gingivitis. Flaxseed oil, known for its antibacterial properties and rich omega-3 content, was found to potentially inhibit the bacteria responsible for these conditions. Additionally, the model successfully hypothesized connections between other food-related entities, like benzoxazinoid and gingelly oil, and health outcomes such as senile osteoporosis linked to soybean oil, offering insights that could influence dietary recommendations.
These findings suggest that THiGER's capabilities go beyond traditional analysis, offering a novel approach to understanding the complex relationships between diet and health, which is particularly important as we face increasing rates of diet-related conditions.
Enhancing Named Entity Recognition in Food Computing
The second research focus is on enhancing Named Entity Recognition (NER) in the food domain through the development of SINERA. These models are designed to accurately identify and classify food-related entities, such as ingredients and nutrients, within large datasets. The robustness of these enhanced NER models enables them to handle the variety and complexity of food-related terms found in real-world data, enhancing intelligent recipe recommendations and health analysis.
"We had to manually review thousands of recipes to identify patterns," Akujuobi explained. "Even though these variations exist, we found some consistent patterns which helped us design the model to be more robust to different writing styles".
In addition to variability, the models had to manage different levels of granularity, such as distinguishing between "apple" as a specific ingredient and "fruit" as a broader category. Akujuobi explained that while it might sound difficult, the model learns these distinctions naturally with the right data. Proper labeling helps the model understand hierarchical relationships between broader and specific categories, making it more effective in diverse datasets.
Looking to the future, SINERA and SINERAS are just initial steps towards a larger goal. The researchers are thinking about systems that can provide practical advice grounded in science, for instance supporting personalized nutrition and health optimization.
There’s Synergy Between Hypothesis Generation and Food Computing!
The true power of these innovations lies in their potential integration. "Whatever is built with one can be applied to the other and vice versa," Akujuobi highlights. By combining the predictive capabilities of THiGER with the precise entity recognition of SINERA/SINERAS, researchers could gain comprehensive insights into the relationships between food components and health outcomes.
For example, SINERA and SINERAS can scan and extract detailed ingredient information from vast recipes and nutritional guide databases. This rich dataset can then be analyzed by THiGER to predict new health benefits from certain ingredient combinations. This synergy accelerates scientific discovery and opens up new potential possibilities for practical applications, such as personalized nutrition plans and the development of health-boosting food products.
Future Directions
The potential for further integration of temporal graph-based hypothesis generation and advanced NER models is vast, with implications not only for food computing but for scientific research and healthcare more broadly. The work presented in these studies is just the beginning. As Akujuobi noted, "This paper is really a first step... Eventually, we want to highlight how AI can be used for this kind of work more generally." Future efforts will likely involve expanding these models to other domains, further improving their robustness, and exploring new applications that can benefit from AI-driven insights.
Conclusion
The combined research from Sony AI demonstrates the potential of AI in accelerating scientific discovery and the possibilities of translating these findings into practical applications. By leveraging THiGER’s ability to predict future connections and SINERA/SINERAS’s precision in identifying food-related entities, researchers can unlock new insights into the health benefits of food components. These advancements are just the beginning of a new era where AI tools work hand-in-hand with researchers, not only accelerating the pace of discovery but also ensuring that these discoveries have the potential to make a tangible impact on society.
Read both papers for more details:
Latest Blog
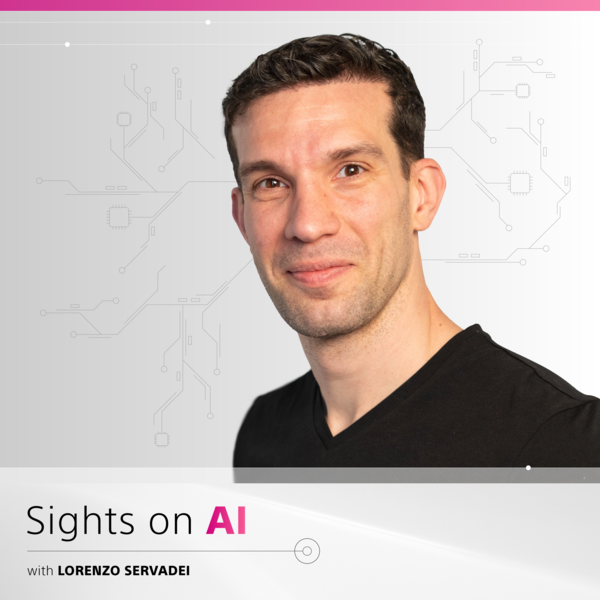
September 13, 2024 | Sony AI
Sights on AI: Lorenzo Servadei Discusses Impact of AI on Chip Design and Semicon…
The Sony AI team is a diverse group of individuals working to accomplish one common goal: accelerate the fundamental research and development of AI and enhance human imagination an…
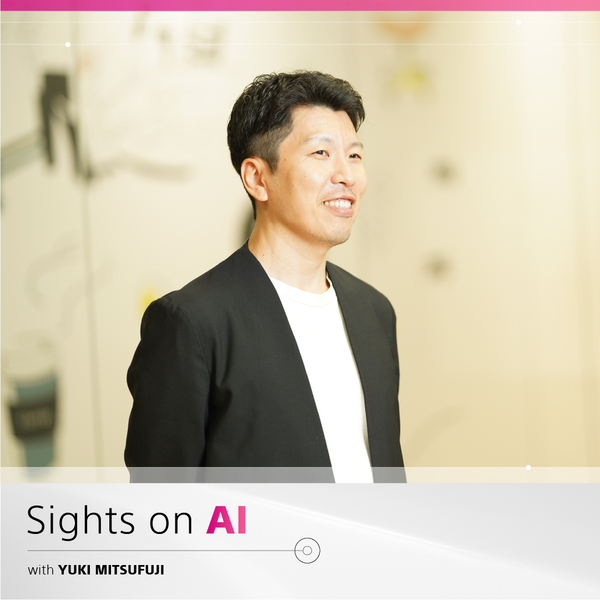
August 14, 2024 | Sony AI
Sights on AI: Yuki Mitsufuji Shares Inspiration for AI Research into Music and S…
The Sony AI team is a diverse group of individuals working to accomplish one common goal: accelerate the fundamental research and development of AI and enhance human imagination an…
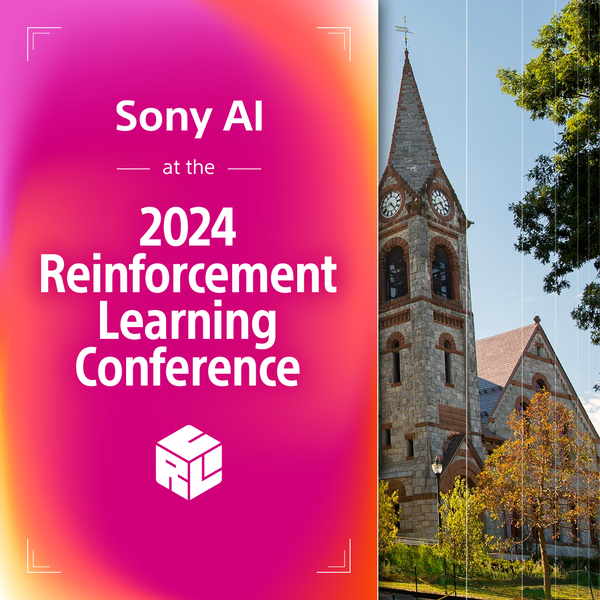
August 10, 2024 | Game AI
Sony AI at the Reinforcement Learning Conference 2024
Sony AI will be participating in the Reinforcement Learning (RL) Conference in Amherst, Massachusetts, from August 9 to 12, 2024 where we will be joining some of the brightest mind…