Exploring the Challenges of Fair Dataset Curation: Insights from NeurIPS 2024
AI Ethics
December 4, 2024
Sony AI’s paper accepted at NeurIPS 2024, "A Taxonomy of Challenges to Curating Fair Datasets," highlights the pivotal steps toward achieving fairness in machine learning and is a continuation of our team’s deep explorations into these issues. This research also marks an evolution of the work from empirical inquiry into theory, taking a Human-Computer Interaction (HCI) approach to the issues.
As ML systems become increasingly embedded in fields such as healthcare, hiring, and fraud detection, to name only a few, the importance of fair dataset curation is clear: Rather than viewing fairness as an "add-on," this research champions a holistic approach to curating equitable datasets.
The study comes at a crucial time, as the field grapples with the best ways to balance fairness criteria, especially in systems where traditional metrics like accuracy don’t tell the whole story. With no universal standard for ML fairness, the conversation has turned to whether regulatory frameworks, such as the European Union's AI legislation, or industry-led standards similar to cybersecurity frameworks, will provide the most effective solutions. With insights from real-world applications, this paper not only addresses common challenges but also suggests practical, adaptable solutions, pushing for systemic changes that can foster a fairer future in AI’s real-world settings.
Real-World Applications and Consequences
Before delving into our research, it’s important to discuss how unfair datasets can have serious consequences where machine learning increasingly guides critical decisions. As the paper discusses, biased data can inadvertently "perpetuate dominant cultural beliefs" and lead to disparities. Tools used by other industries, when trained on historical data reflecting past judicial biases, often reinforce existing inequalities. For example, "spurious correlations with demographic attributes are ubiquitous" in some datasets, making it challenging to avoid biased decisions and compounding the risk of mislabeling certain groups as high-risk.
The paper’s research explores how curators grapple with fairness challenges at each stage of the process and suggests that "building fair datasets requires systemic change rather than solely relying on individual contributions." These systemic changes are crucial to preventing unintended harms and fostering AI systems that are fair, inclusive, and capable of benefiting diverse communities.
The Ideal Approach: Addressing Practical Realities of Fair Dataset Curation
Despite the increasing focus on fairness in ML, the practicalities of curating fair datasets are often overlooked. Our researchers aimed to fill this gap by moving beyond theoretical guidelines to understand how fair dataset curation unfolds in real-world settings. They conducted in-depth interviews with curators working with vision-, language-, and multi-modal datasets, exploring the nuanced challenges that emerge throughout the process.
Fair dataset curation extends beyond simply gathering diverse data; it encompasses three crucial dimensions—composition, process, and release—that guide curators in embedding fairness at every stage. As the researchers emphasize, "Fairness is not only a property of the final artifact—the dataset—but also a constant consideration curators must account for throughout the curation process." This approach ensures that fairness is treated as an integral part of dataset creation, not just an afterthought.
- Composition involves ensuring that datasets represent various perspectives and demographics, capturing varied experiences across cultural, geographic, and social contexts. Achieving this representation and particularly "balancing fairness with utility" is often challenging for curators when diverse and nuanced data is limited by availability.
- Process addresses the creation of datasets, focusing on ethical labor practices for annotators and transparency in data gathering. The study’s participants emphasized that "[R]esearchers [need] to make sure that annotators represent everyone because [if] not, you’re just gonna have a skewed pool of annotations as well." This dimension asks whether the data collection process respects contributors’ rights and ensures fair compensation, aiming to avoid exploitative practices that can compromise data quality and integrity.
- Release centers on transparency and accessibility once datasets are shared. It ensures datasets are openly available, with clear documentation on how the data was curated. Such transparency helps researchers and practitioners understand the context and limitations of the dataset, preventing misuse or unintended bias in future applications. One participant underscored that achieving this transparency is essential to prevent "fairness issues with data release [that] are linked to concerns about composition."
By addressing these three dimensions, this study highlights persistent, practical challenges in real-world dataset curation. Tackling these challenges is crucial as curators navigate trade-offs and strive to meet fairness goals in meaningful and actionable ways.
The Challenges
Defining Fairness Across Contexts
Fairness has many interpretations depending on the domain, task, and cultural context. For example, a study participant highlighted, "For a [South Asian] country, race does not manifest like it manifests for America." This diversity in definitions required curators to make trade-offs between different fairness dimensions, with one researcher remarking, "You can’t have complete diversity with respect to, say, races, geographies, times of the day, and other domains. Everything is not possible."
Data Availability and Annotation Limitations
It is difficult to collect diverse data because of how hard it can be to access information from underrepresented regions or recruit annotators with the requisite expertise. The study noted that biases can arise when "crowdsourced annotators regularly embed gender biases into datasets." This issue is compounded by systemic challenges, such as laws limiting access to demographic details, which obstruct efforts to create datasets that accurately reflect various communities.
The Inherent Bias of Taxonomies
Standard categorization methods could unintentionally perpetuate bias. For example, binary gender classifications or taxonomies based on broad geographic regions may fail to capture the true diversity of experiences. Conclusion
The findings from this study provide a detailed taxonomy that maps the challenges of fair dataset curation to different phases of the dataset lifecycle. This taxonomy can guide future research and practices in curating fair datasets by highlighting the obstacles at the individual, disciplinary, organizational, regulatory, and socio-political levels.
The study also underscores the need for flexible and context-specific approaches to data curation. The authors suggest that "...systemic changes across organizational, disciplinary, and regulatory levels" are necessary to enable fairer practices. The insights gained from the interviews offer practical recommendations, such as adopting flexible taxonomies and supporting fair labor practices for annotators, that could significantly improve the fairness of datasets used in ML applications.
Fair dataset curation is a complex and evolving issue that requires continuous effort across various dimensions of the dataset lifecycle. By focusing on the practical realities faced by dataset curators, this study provides a roadmap for addressing fairness more comprehensively. With ongoing efforts to implement these recommendations, we may see a future where fairness moves beyond an aspiration or "add-on" to instead become a fundamental aspect of ML dataset curation and utilization.
Latest Blog
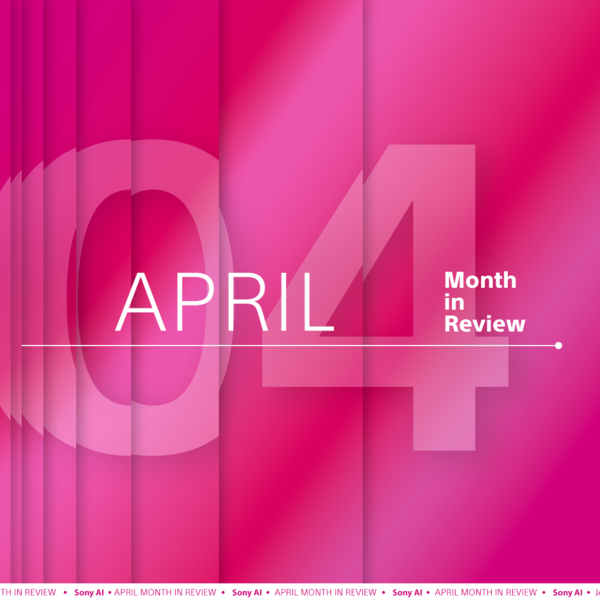
May 1, 2025 | Sony AI
Advancing AI: Highlights from April
April marked a standout moment for Sony AI as we brought bold ideas to the world stage at ICLR 2025. This year’s conference spotlighted our work across generative modeling, multimo…
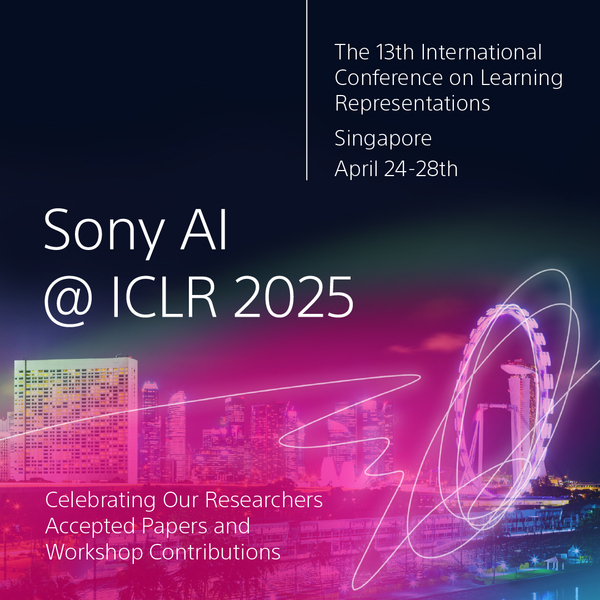
April 23, 2025 | Events, Sony AI
Sony AI at ICLR 2025: Refining Diffusion Models, Reinforcement Learning, and AI …
This April, Sony AI will present a wide-ranging portfolio of research at the International Conference on Learning Representations (ICLR 2025). From progressing text-to-sound and vi…
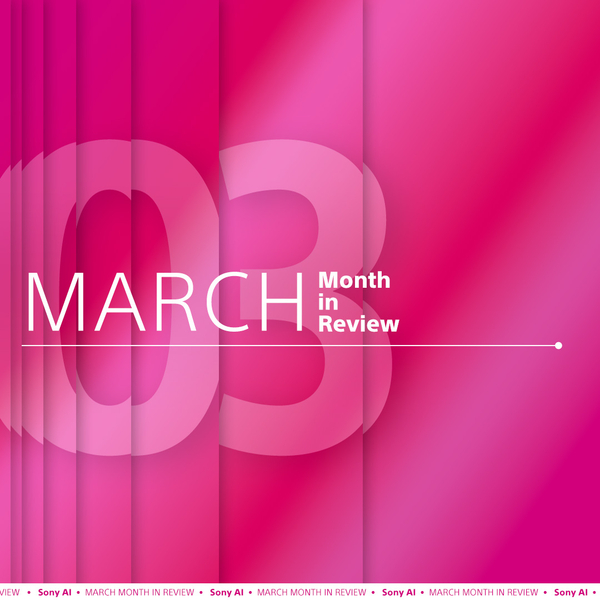
April 1, 2025 | Sony AI
Advancing AI: Highlights from March
March was a month of notable advancements, global discussions, and strategic collaborations, reinforcing our commitment to responsible AI innovation. From pioneering multi-modal AI…