Mitigating Bias in AI Models: A New Approach with TAB
AI Ethics
October 4, 2024
Artificial intelligence models, especially deep neural networks (DNNs), have proven to be powerful tools in tasks like image recognition and natural language processing. However, their susceptibility to bias is a persistent challenge, particularly in tasks involving human-centric data. Bias often arises from spurious correlations, where models learn incorrect patterns due to the overrepresentation of certain data features.
Shortcut Learning and the Problem of Spurious Correlations
One well-known example of this phenomenon is the association between cows and grassy backgrounds. If a model is trained on a dataset where most images of cows are set against grassy fields, it might mistakenly learn that the presence of grass is a key feature in identifying cows. Consequently, the model may start associating grassy backgrounds with cows, even in images where no cows are present. This flawed learning process is called shortcut learning, where the model takes a "shortcut" by focusing on an irrelevant feature (the grass) instead of the actual object it is supposed to identify (the cow).
The study, Efficient Bias Mitigation Without Privileged Information, was led by an intern team, including Sony AI intern Mateo Espinosa Zarlenga. Sony AI researcher Jerone Andrews, who expanded on the research, explained that similar spurious correlations can occur in human-centric tasks: "For example, a model might detect kitchen utensils in an image and wrongly infer the presence of a woman, even if no woman is actually in the image." This highlights how models can focus on irrelevant details instead of accurately identifying people or objects.
The problem of shortcut learning extends to many domains beyond image recognition. For instance, in the Waterbirds dataset used in this Targeted Augmentations for Bias Mitigation (TAB) research, the model initially struggled to correctly classify birds when the background (water or land) didn’t align with the typical environment of that bird species.
As Andrews explained, "In the Waterbirds dataset, ducks are typically shown on water, and land birds are shown on land. But when these backgrounds are flipped—like a waterbird shown on land—the model often misclassifies the bird because it relies too heavily on the background context rather than the bird itself."
In essence, shortcut learning arises when AI models focus on features that frequently co-occur with the desired output, instead of learning the actual underlying relationship between the object and its true identifying characteristics. TAB addresses this problem by identifying these spurious correlations through the model’s training history and rebalancing the dataset to present the model with more challenging examples, such as cows not on grass or birds in atypical environments, allowing the model to generalize better.
How TAB Works
Sony AI researchers have developed a new method called Targeted Augmentations for Bias Mitigation (TAB), let’s talk about how it works. Unlike traditional bias mitigation techniques that rely on group labels or extensive hyperparameter tuning, TAB offers a hyperparameter-free framework that can be easily integrated into existing training workflows.
"TAB uses the training history of a model to identify bias-aligned and bias-conflicting samples, then augments the dataset by oversampling the underrepresented or bias-conflicting group. This approach allows us to retrain the model in a more balanced way, improving fairness without sacrificing overall accuracy," Andrews explains.
At the heart of TAB is the use of training history to monitor how well the model learns different types of data over time. As the model progresses through its training, TAB keeps track of which samples it finds easier (bias-aligned) and which are more challenging (bias-conflicting). Andrews explains, "Bias-aligned samples are those that match the model's existing learned shortcuts, while bias-conflicting samples are those that contradict these learned associations, like a waterbird shown on land or a land bird shown on water."
Once these two groups are identified, TAB rebalances the dataset by oversampling the minority group, effectively increasing the number of challenging examples. This forces the model to learn from a more diverse and balanced dataset, ultimately improving its ability to generalize across different types of data. "Because TAB doesn't require any additional group labels or complex hyperparameter tuning, it offers a much simpler and more efficient way to mitigate bias in AI models," Andrews adds.
The Broader Implications of TAB
While TAB was initially designed to address bias in tasks like image classification, its applications extend beyond human-centric tasks. "We often think of bias mitigation in the context of fairness to humans, but TAB can be applied to any task where spurious correlations affect model performance," says Andrews. "For example, in pedestrian detection, a model might learn to associate hats with pedestrians if most of the training data includes pedestrians wearing hats. TAB helps correct these kinds of biases by ensuring the model doesn't over-rely on any one feature."
The development of TAB is part of a broader push within Sony AI to create tools that make AI systems fairer and more reliable. Andrews notes that the ultimate goal is for TAB to be widely adopted by researchers and companies. "It's important that we don't just think about fairness in abstract terms, but also develop practical tools that can be used in real-world AI applications. TAB is one such tool, and we hope it will have a positive impact on the AI community."
Ethical Considerations
While TAB shows promise in mitigating bias, it is not without ethical concerns. Training the model twice, as required by TAB, increases the carbon footprint associated with AI training, an issue that cannot be ignored. "Although TAB doubles the training time, this impact is offset by its efficiency in avoiding complex model selection procedures," explains Andrews. Additionally, the method's ability to identify bias-conflicting samples could potentially be misused by bad actors to further increase bias in a model. "It's a risk that comes with any bias mitigation method, not just TAB," Andrews says, emphasizing the importance of responsible AI development.
Conclusion
Targeted Augmentations for Bias Mitigation (TAB) represents a significant step forward in addressing the issue of bias in AI models. By leveraging training history to create a balanced dataset, TAB offers a simple, efficient, and effective solution to a long-standing problem in AI research. As Andrews notes, "TAB is about making AI systems more reliable and fair, without the need for complex setups or additional data. It's a tool that can help us move towards a future where AI models are not only powerful but also equitable."
Read more about our pivotal work in AI ethics and bias mitigation:
- Ushering in Needed Change in the Pursuit of More Diverse Datasets – Sony AI
- When Privacy and Fairness Collide: Reconciling the Tensions Between Privacy and Representation in the Age of AI – Sony AI
- Being 'Seen' vs. 'Mis-Seen': Tensions Between Privacy and Fairness in Computer Vision – Sony AI
- Beyond Skin Tone: A Multidimensional Measure of Apparent Skin Color – Sony AI
Latest Blog
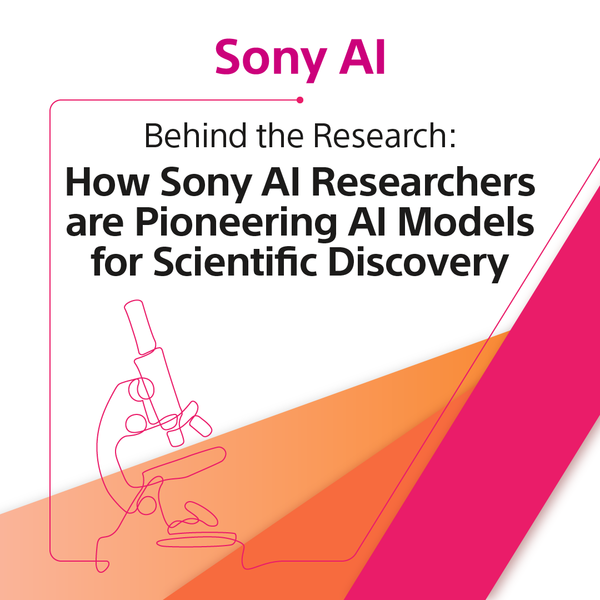
September 14, 2024 | Scientific Discovery
Behind the Research: How Sony AI Researchers are Pioneering AI Models for Scient…
The pace of scientific research is accelerating, with an exponential increase in published research articles each year. For instance, in 1980, approximately 500,000 scientific arti…
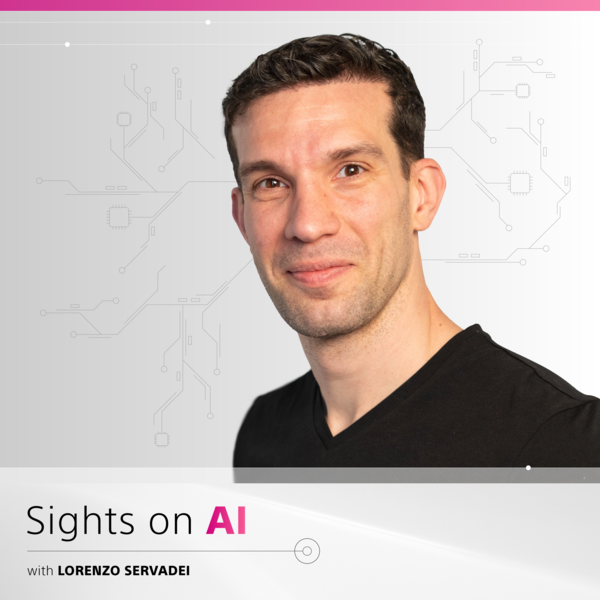
September 13, 2024 | Sony AI
Sights on AI: Lorenzo Servadei Discusses Impact of AI on Chip Design and Semicon…
The Sony AI team is a diverse group of individuals working to accomplish one common goal: accelerate the fundamental research and development of AI and enhance human imagination an…
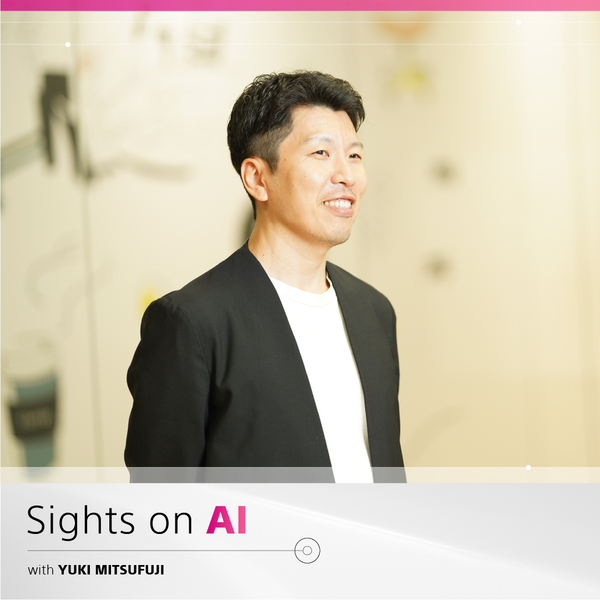
August 14, 2024 | Sony AI
Sights on AI: Yuki Mitsufuji Shares Inspiration for AI Research into Music and S…
The Sony AI team is a diverse group of individuals working to accomplish one common goal: accelerate the fundamental research and development of AI and enhance human imagination an…