Revolutionizing Hypothesis Generation
How do great scientific discoveries come about? What is the recipe for revolutionary research?
Life at Sony AI
July 9, 2021
In part, scientific research is led by the hypothesis – the supposition or proposal that forms the basis for further investigation. Traditionally, such hypotheses are formed by the researcher and their team, taking into account vast amounts of previous scientific research. However, this task is becoming more difficult, as the volume of data and research has grown exponentially.
A recent paper from our CEO, Dr. Hiroaki Kitano, touched on similar topics where he said, “Understanding, reformulating, and accelerating the process of scientific discovery is critical in solving problems we are facing...”
My research into how we might automate hypothesis generation could solve such issues and lead to giant new discoveries.
Project Background
The idea for this project was planted in 2019, during my internship at Sony CSL under the supervision of Michael Spranger (now, COO of Sony AI), and in collaboration with Sucheendra K. Palaniappan of the Systems Biology Institute, Japan. In the years since, with the help of my Ph.D. supervisor, Professor Xiangliang Zhang, this idea has been watered and nurtured to produce several works that can aid in the rigorous and often time-consuming process of scientific research.
As we set out in this project, our aim was to automate the process of discovering implicit relationships between scientific terms in scholarly literature, and identify the hypotheses that resulted from this.
Introduction to Biomedical Hypotheses Generation
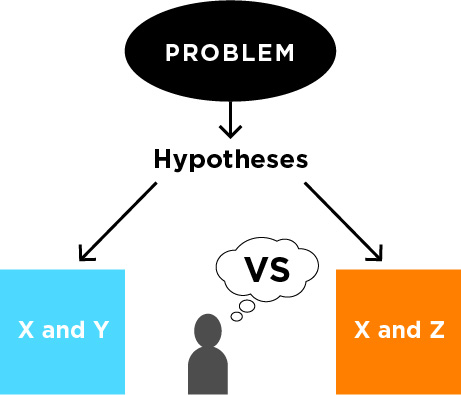
The Oxford English Dictionary defines a hypothesis as “a supposition or proposed explanation made on the basis of limited evidence as a starting point for further investigation.” While applicable to several problem domains, the act of hypotheses construction is essential for scientific discoveries. The road to scientific discoveries has traditionally involved scientific expertise and ideas coalescing to form hypotheses, which are then checked for validity.
Hypothesis generation systems are often domain specific. For instance, in the gastronomy domain, a hypothesis could be: will ingredient X improve the taste of recipe Z, or will ingredient Y be a better choice?
In our project, we focused on the biomedical domain, with the intention to discover meaningful implicit connections between scientific terms, including but not limited to diseases, chemicals, drugs, and genes extracted from databases of biomedical articles.
In this domain, the manual process of hypothesis generation often involves the tedious process of reading through articles in the domain of interest. Of course, the more articles read, the more knowledge and expertise is obtained to make knowledgeable hypotheses. However, with the advancement of technology, the number of publications is growing exponentially. This fast growth has made the manual process of hypothesis generation even harder.
Hence, computer systems like ours have been introduced to augment the efforts of researchers and enhance the research experience.
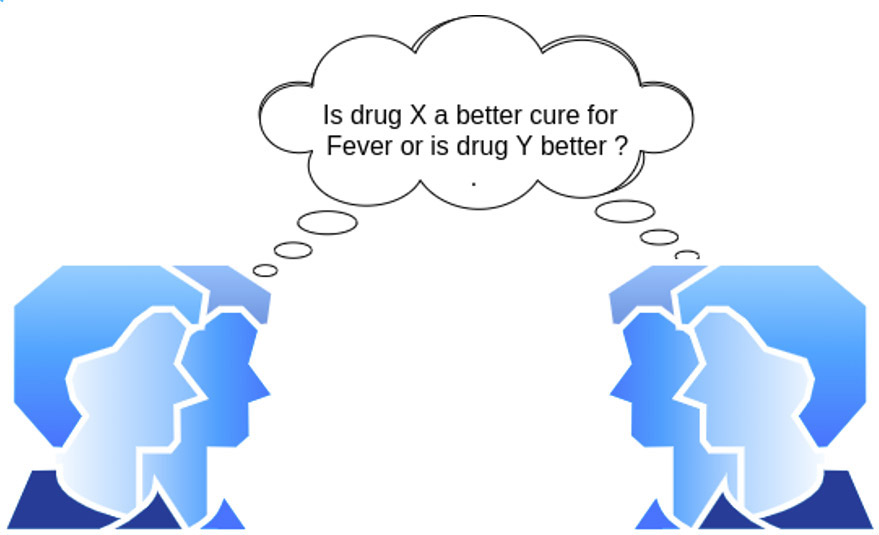
Approach to the Project
The goal of my initial project was to try to understand and extract knowledge from biomedical text. In this context, the knowledge to be extracted is often contained in biomedical publication texts. Sometimes information can be explicitly given in a text; however, it is often hidden implicitly in the text. Therefore, extracting this biomedical knowledge requires the study and understanding of the text.
We posed a question: Can this knowledge be extracted automatically from biomedical texts? If so, what kind of information can be extracted?
After several discussions, we came up with an idea to extract information, to infer the relationship between keywords – including diseases, drugs, and others.
For instance, should there be a relationship between a given drug and a given disease, or the relationship between several diseases? The first step was to identify which terms might be relevant (with both positive and negative connotations). Further steps would include differentiating the different types of extracted relationships and providing an explainable model that does not just infer a hypothesis but also provides a way to understand how the hypothesis is made.
What we did
Over the course of several months, we proposed two methods to generate hypotheses by learning from the relationship evolution of the terms. These methods are discussed in detail in the paper.
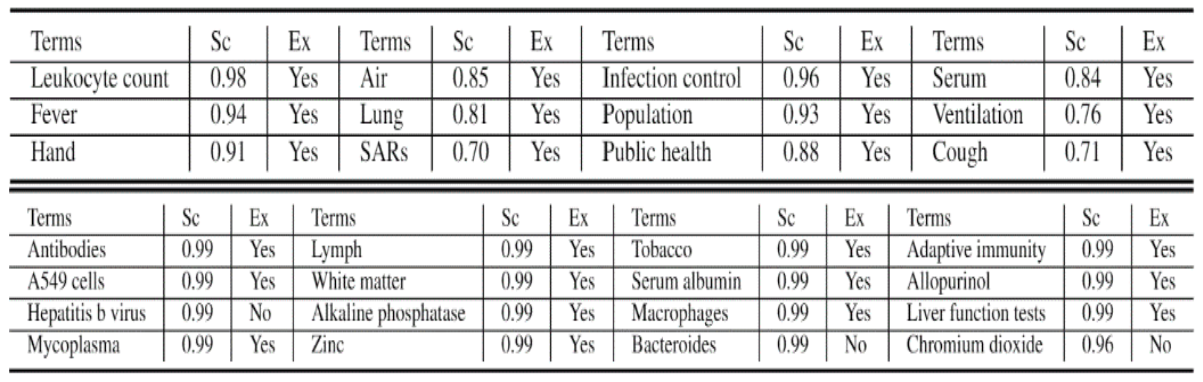
A use case of our proposed method can be seen in the application of hypothesis generation on COVID-19. This investigation tries to answer the question, ‘Do the top-ranked terms predicted to be related to COVID-19 have an actual connection in the observed data?’
In other words, are they meaningful discoveries? The top predicted terms predicted to be connected with covid-19 are shown in Table 1.
In Table 1 (top), we notice that the top terms are truly relevant to ‘covid-19’, and we do observe their connection in the evaluation data. For instance, Cough, Fever, SARS, Hand (washing of hands) were known to be relevant to covid-19.
In Table 1 (bottom), we did find there exist discussions between ‘covid-19’ and some top ranked terms, for example, Fortune Business Insight presents how covid-19 affected the market of Chromium oxide and hepb talks about caring for people living with Hepatitis B virus during the covid-19 spread.
Applications in Biomedicine and Beyond
In this project, we focused on automatic biomedical hypothesis generation, which involves predicting the existence of a connection between two biomedical terms. The results obtained from this research show that computers can be exploited in the automatic information retrieval from text for hypothesis generation.
One of the many directions this research could take in the future is to predict the relationship strength between drugs and diseases. This has implications for the biomedical field, but also for different domains like gastronomy. For instance in ingredient pairing, to predict the ingredients that go well together.
For more information about this project please see https://proceedings.neurips.cc/paper/2020/file/310614fca8fb8e5491295336298c340f-Paper.pdf
and https://ieeexplore.ieee.org/document/9170911.
Sony AI is always looking to develop partnerships with academic institutions and food companies to develop knowledge in AI and gastronomy. And we are always looking to work with talented researchers like Uchenna! Please get in touch if you’d like to discuss more.
Uchenna Akujuobi
Research Scientist
Uchenna Akujuobi is currently a research scientist with the SonyAI gastronomy team based in Tokyo. His research interests include network embedding, graph mining, information retrieval,text mining, and deep neural networks. Born in Nigeria, he obtained his BS degree in Saint Petersburg Electrotechnical University and his MS and PhD degree in the MINE Laboratory at the King Abdullah University of Science and Technology. He is motivated by the use of AI to augment human abilities for more creativity and improved performance. He believes in pushing the boundary between human possibilities and impossibilities one AI step at a time.
Latest Blog
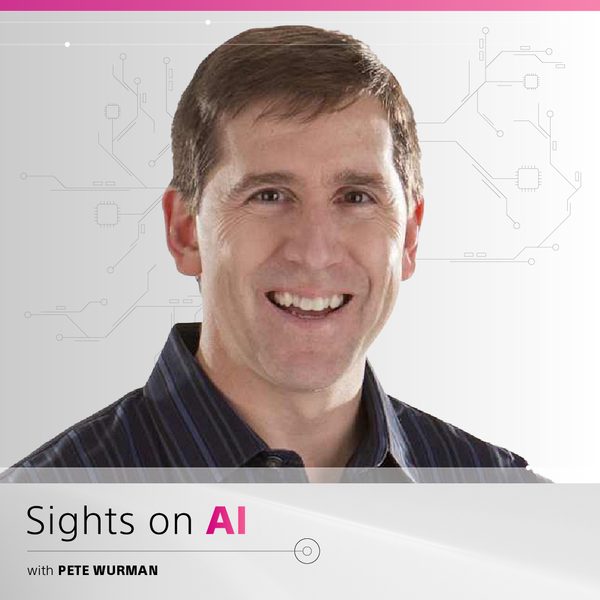
February 5, 2025 | Sony AI
Sights on AI: Pete Wurman Discusses Career in AI and How the Technology is Evolv…
The Sony AI team is a diverse group of individuals working to accomplish one common goal: accelerate the fundamental research and development of AI and enhance human imagination an…
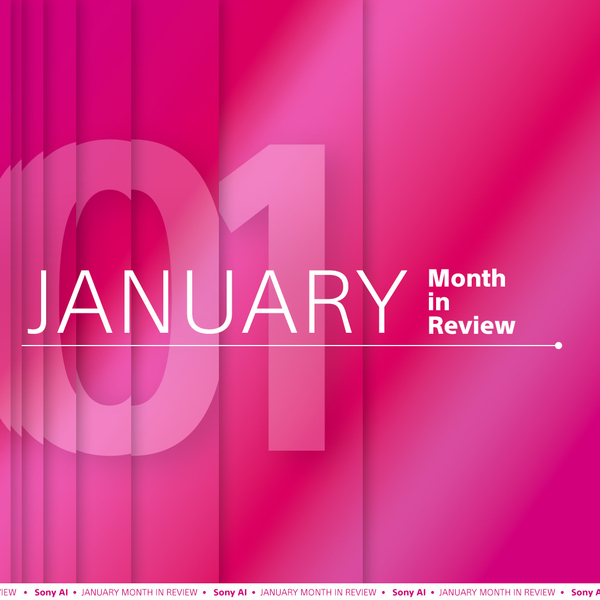
January 31, 2025 | Sony AI
Advancing AI: Highlights from January
At Sony AI, every month is an opportunity to innovate, connect, and share our progress with you. Welcome to Advancing AI, our brand-new monthly round-up series where we highlight t…
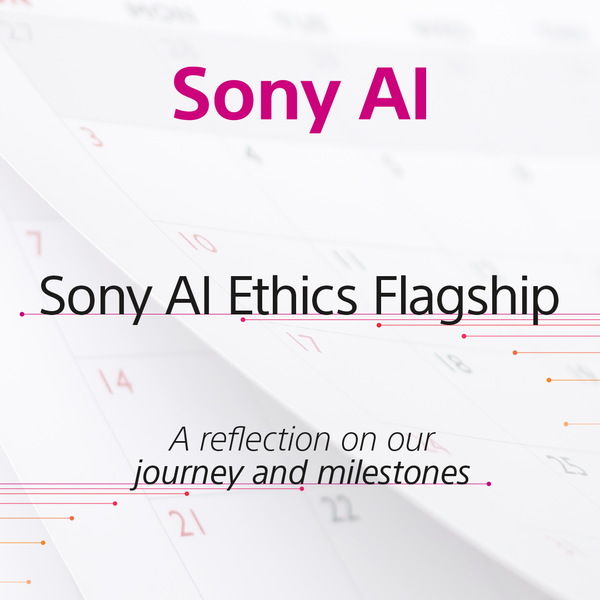
January 14, 2025 | AI Ethics
Sony AI Ethics Flagship: Reflecting on Our Progress and Purpose
Since Sony AI’s inception, ethics has been at the heart of everything we do. The establishment of our AI Ethics Research Flagship at the beginning of our journey laid the foundatio…