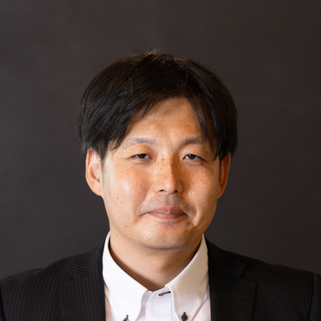
Takashi
Shibuya
Publications
In this work, we build a simple but strong baseline for sounding video generation. Given base diffusion models for audio and video, we integrate them with additional modules into a single model and train it to make the model jointly generate audio and video. To enhance align…
This work addresses the lack of multimodal generative models capable of producing high-quality videos with spatially aligned audio. While recent advancements in generative models have been successful in video generation, they often overlook the spatial alignment between audi…
In text-to-motion generation, controllability as well as generation quality and speed has become increasingly critical. The controllability challenges include generating a motion of a length that matches the given textual description and editing the generated motions accordi…
Diffusion models are prone to exactly reproduce images from the training data. This exact reproduction of the training data is concerning as it can lead to copyright infringement and/or leakage of privacy-sensitive information. In this paper, we present a novel way to unders…
We propose to synthesize high-quality and synchronized audio, given video and optional text conditions, using a novel multimodal joint training framework MMAudio. In contrast to single-modality training conditioned on (limited) video data only, MMAudio is jointly trained wit…
In this work, we build a simple but strong baseline for sounding video generation. Given base diffusion models for audio and video, we integrate them with additional modules into a single model and train it to make the model jointly generate audio and video. To enhance align…
Sound content is an indispensable element for multimedia works such as video games, music, and films. Recent high-quality diffusion-based sound generation models can serve as valuable tools for the creators. However, despite producing high-quality sounds, these models often …
Controllable generation through Stable Diffusion (SD) fine-tuning aims to improve fidelity, safety, and alignment with human guidance. Existing reinforcement learning from human feedback methods usually rely on predefined heuristic reward functions or pretrained reward model…
In this study, we aim to construct an audio-video generative model with minimal computational cost by leveraging pre-trained single-modal generative models for audio and video. To achieve this, we propose a novel method that guides single-modal models to cooperatively genera…
Sound content creation, essential for multimedia works such as video games and films, often involves extensive trial-and-error, enabling creators to semantically reflect their artistic ideas and inspirations, which evolve throughout the creation process, into the sound. Rece…
Generating novel views from a single image remains a challenging task due to the complexity of 3D scenes and the limited diversity in the existing multi-view datasets to train a model on. Recent research combining large-scale text-to-image (T2I) models with monocular depth e…
Contrastive cross-modal models such as CLIP and CLAP aid various vision-language (VL) and audio-language (AL) tasks. However, there has been limited investigation of and improvement in their language encoder, which is the central component of encoding natural language descri…
Recent advances in generative models that iteratively synthesize audio clips sparked great success to text-to-audio synthesis (TTA), but with the cost of slow synthesis speed and heavy computation. Although there have been attempts to accelerate the iterative procedure, high…
Generative adversarial network (GAN)-based vocoders have been intensively studied because they can synthesize high-fidelity audio waveforms faster than real-time. However, it has been reported that most GANs fail to obtain the optimal projection for discriminating between re…
Vector quantization (VQ) is a technique to deterministically learn features with discrete codebook representations. It is commonly performed with a variational autoencoding model, VQ-VAE, which can be further extended to hierarchical structures for making high-fidelity recon…
Diffusion-based speech enhancement (SE) has been investigated recently, but its decoding is very time-consuming. One solution is to initialize the decoding process with the enhanced feature estimated by a predictive SE system. However, this two-stage method ignores the compl…
Sound event localization and detection (SELD) systems estimate direction-of-arrival (DOA) and temporal activation for sets of target classes. Neural network (NN)-based SELD systems have performed well in various sets of target classes, but they only output the DOA and tempor…
Generative adversarial networks (GANs) learn a target probability distribution by optimizing a generator and a discriminator with minimax objectives. This paper addresses the question of whether such optimization actually provides the generator with gradients that make its d…
Generative adversarial network (GAN)-based vocoders have been intensively studied because they can synthesize high-fidelity audio waveforms faster than real-time. However, it has been reported that most GANs fail to obtain the optimal projection for discriminating between re…
Diffusion-based speech enhancement (SE) has been investigated recently, but its decoding is very time-consuming. One solution is to initialize the decoding process with the enhanced feature estimated by a predictive SE system. However, this two-stage method ignores the compl…
Sound event localization and detection (SELD) systems estimate direction-of-arrival (DOA) and temporal activation for sets of target classes. Neural network (NN)-based SELD systems have performed well in various sets of target classes, but they only output the DOA and tempor…
Audio classification and restoration are among major downstream tasks in audio signal processing. However, restoration derives less of a benefit from pretrained models compared to the overwhelming success of pretrained models in classification tasks. Due to such unbalanced b…
Although deep neural network (DNN)-based speech enhancement (SE) methods outperform the previous non-DNN-based ones, they often degrade the perceptual quality of generated outputs. To tackle this problem, we introduce a DNN-based generative refiner, Diffiner, aiming to impro…
One noted issue of vector-quantized variational autoencoder (VQ-VAE) is that the learned discrete representation uses only a fraction of the full capacity of the codebook, also known as codebook collapse. We hypothesize that the training scheme of VQ-VAE, which involves some…
Blog
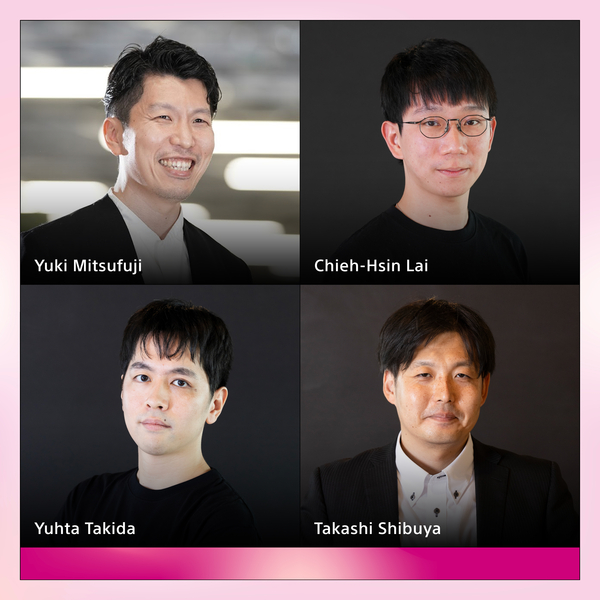
May 10, 2024 | Events | Sony AI
Revolutionizing Creativity with CTM and SAN: Sony AI's Groundbreaking Advances in Generative AI for Creators
In the dynamic world of generative AI, the quest for more efficient, versatile, and high-quality models continues to push forward without any reduction in intensity. At the forefront of this technological evolution are Sony AI's r…
In the dynamic world of generative AI, the quest for more efficient, versatile, and high-quality models continues to push forward …
JOIN US
Shape the Future of AI with Sony AI
We want to hear from those of you who have a strong desire
to shape the future of AI.