Exposing Limitations in Fairness Evaluations: Human Pose Estimation
AI Ethics
April 17, 2023
As AI technology becomes increasingly ubiquitous, we reveal new ways in which AI model biases may harm individuals. In 2018, for example, researchers Joy Buolamwini and Timnit Gebru revealed how commercial services classify human faces with far higher error rates when individuals are darker-skinned females rather than lighter-skinned males. To anticipate and mitigate these biases, it has become crucial for AI practitioners to perform fairness evaluations on their models, thereby allowing them to detect serious problems before AI services are ever deployed. However, running a fairness evaluation is more complex than it may seem. The AI Ethics team at Sony AI has exposed some of these barriers, as demonstrated by a case study in 2D human pose estimation.
Our research on this topic was recently accepted at the AAAI 2023 Workshop on Representation Learning for Responsible Human-Centric AI (R2HCAI): “A Case Study in Fairness Evaluation: Current Limitations and Challenges for Human Pose Estimation.”
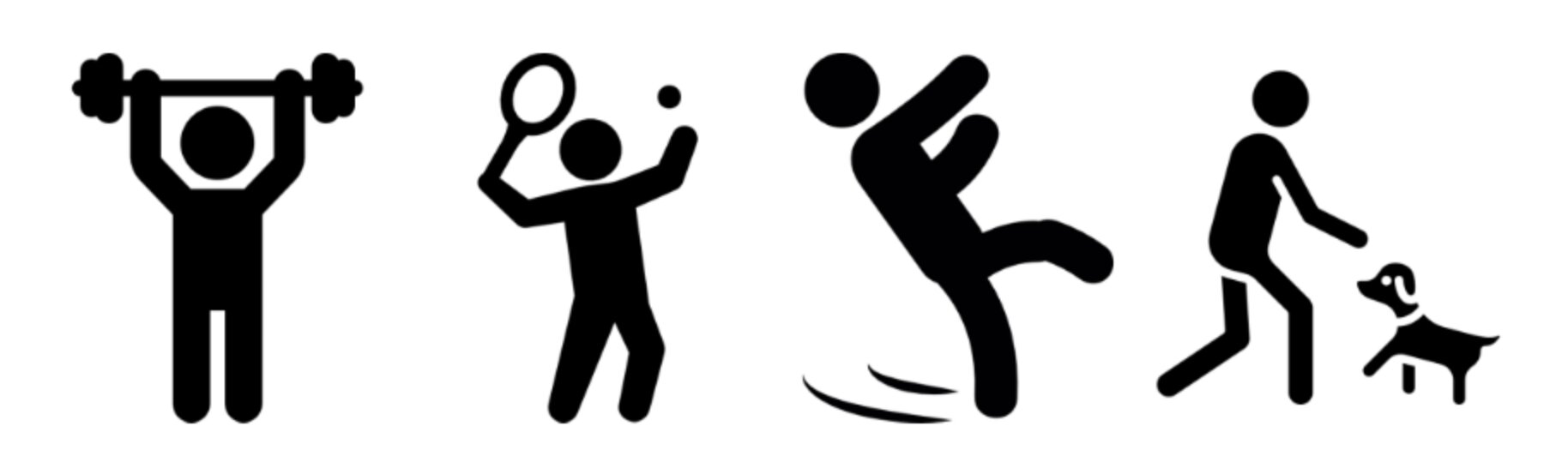
2D pose estimation guides human understanding in images, such as counting repetitions in sports, recognizing activities, and detecting falling or interactions.
We focused on 2D human pose estimation due to its utility in a wide range of human-centric visual tasks. Pose estimation - here, identifying various joint and body keypoint locations within an image or video of a person - allows us to perform tasks like action recognition, such as identifying whether a person is swinging a tennis racket or playing basketball in a YouTube video. In healthcare, pose estimation models may help determine whether patients have fallen and require immediate care. In such critical-care settings, we want to be absolutely sure that models perform well for all patients, regardless of sensitive attributes like race, age, and gender. Yet, gaining access to comprehensive, diverse datasets to fairly test pose estimation models across these attribute groups is not always easy. Why? Let’s examine some challenges below.
Challenge #1: Lack of Demographic AnnotationsFirst, we’ll take a look at the most commonly utilized datasets for pose estimation and assess whether or not they are viable candidates for performing fairness evaluations. As a measure of popularity, we’ll examine the Google Scholar citation counts for each dataset’s publication, as shown in the plot below. By far, the Common Objects in Context (COCO) dataset (with applications specific to “pose”) is the most commonly utilized dataset in the pose estimation space, followed by MPII Human Pose.
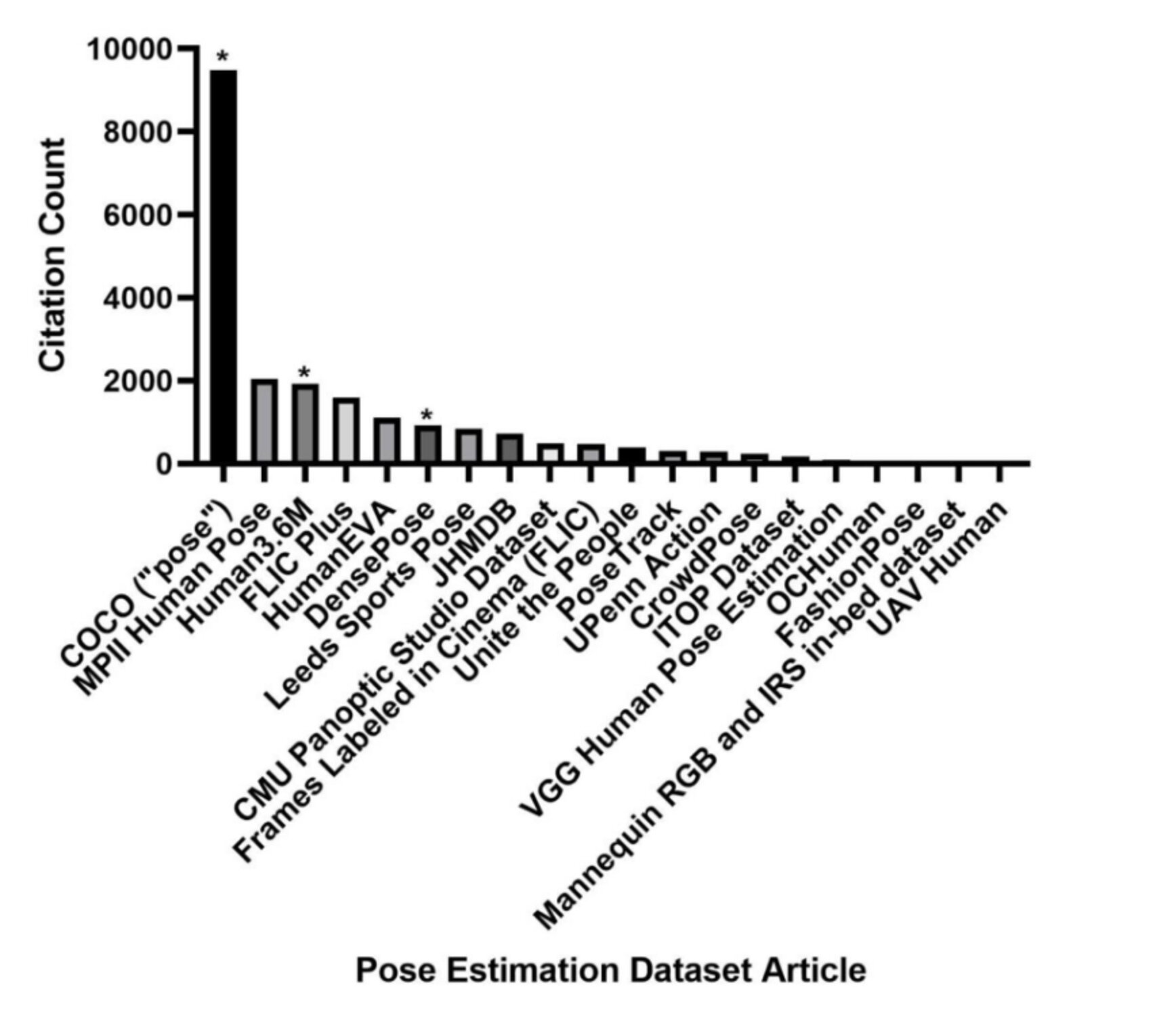
While COCO is the most commonly utilized dataset for pose estimate, it has not been designed with demographic annotation collection from the start.
Are any of these datasets useful for fairness evaluations based on sensitive attributes like age, race, gender, disability status, and so on? Our verdict, after careful analysis, is no. Out of all the datasets considered, only the Human3.6M dataset had gender data associated with the original dataset beyond the standard pose estimation keypoint annotations. Beyond this, external researchers supplemented the COCO dataset (and therefore the DensePose dataset, which inherits from COCO) with perceived gender and rough skin tone annotations. Such perceived labels are typically unreliable; in this case, the annotations are not available for commercial use, further limiting the potential to perform fairness evaluations using them. Thus, a healthcare team wishing to test its fall-detection system for bias according to race, gender, age, disability, and other sensitive attributes would have no means of doing so due to the lack of data.
So, what is the alternative when the data is lacking? Often, AI practitioners and users may choose to skip fairness evaluations entirely or end up collecting their own data manually - which is usually too expensive and time-consuming to enable the collection of a sizable dataset. In the next section, we’ll see an example of how AI practitioners may encounter problems in attempting to create their own demographic annotations.
Challenge #2: Imbalanced Demographic LabelsSecond, given the widespread use of COCO, we explore its potential as a fairness evaluation dataset. To achieve this, we collect demographic annotations related to gender, age, and skin tone, similar to the model card for MoveNet. Our annotations cover 657 images of the COCO 2017 validation set for 2D human pose estimation. We make them publicly available for research and commercial purposes.
What is the distribution of the demographic labels? We observe imbalances where (i) male individuals are approximately twice as common as female individuals; (ii) older individuals are less frequently represented; and (iii) lighter-skinned individuals are present over ten times as frequently as darker-skinned individuals. When looking at intersectional groups, darker-skinned females appear to be present within only 17 of the 657 valid images (only 2.6%!), whereas lighter-skinned males comprise 394 (60.0%). Such demographic imbalances limit the applicability of COCO for fairness evaluation purposes and question the validity of fairness evaluations given the low sample number.
How should the demographic labels be annotated? We observe significant differences between manually-produced annotation results and the automated attribute prediction results. Automated and manual annotations differed by more than 30% for age and gender and up to 70% for skin tone. Estimated errors from purely automatic attribute prediction could invalidate the results of any fairness evaluation performed with such annotations. Due to these risks, we encourage fairness evaluators to carefully assess annotation quality.
Challenge #3: Fairness Evaluation Results (with Limited Samples) Third, we evaluate several 2D human pose estimation models using the proposed COCO-KD dataset, namely OpenPose, MoveNet, and PoseNet. We report overall performance as well as performance broken down by demographic groups and their intersectional subgroups.
What is the performance of COCO-KD? Bottom-up approaches such as OpenPose and MoveNet perform better than top-down approaches such as PoseNet. Out of all three models, OpenPose outperforms both the other models in terms of mean average precision (mAP) and mean average recall (mAR) of object keypoint similarity (Table 1). On evaluation based on demographic annotations, it is observed that performance discrepancies exist for all the evaluated models regardless of the demographic label (gender, age, skin tone) (Table 2-4). For instance, OpenPose and MoveNet perform better for male subjects, whereas PoseNet performs better for females. Even in the case of intersectional analysis, males with lighter skin tones yield a higher score regardless of the model. Still, in the case of PoseNet, females with lighter skin tones outperform their male counterparts.
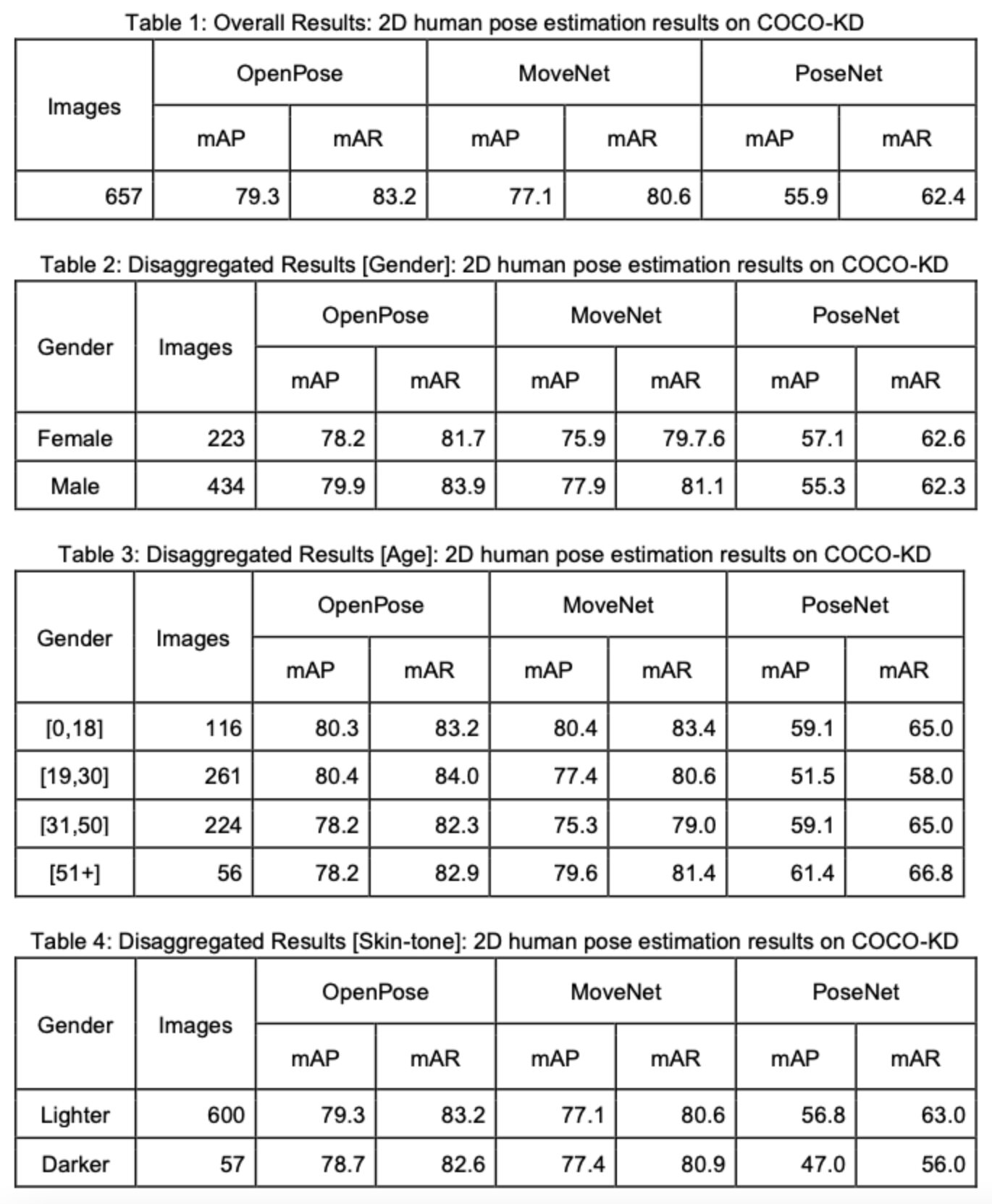
Can the fairness results be influenced? Yes! The results can be sensitive to the samples selected for evaluation, especially when dealing with imbalanced sets. We evaluated by subsampling data in each category across attributes to be the same size of the group as the smallest size. For demographic labels of gender, age and skin, we subsample to obtain 223, 56 and 57 images for each group, respectively. We perform the experiment 100 times and report the mean and standard deviation(Figure 3). Similar trends are observed, as reported in Table 1-2, for gender and age group; however, the bias direction appears very sensitive to the sample set when considering skin tone. The direction in OpenPose and MoveNet can show discrimination with a large margin based on the subset of images. These results question the robustness of fairness evaluations. When dealing with imbalanced data, it is suggested to subsample data and perform fairness evaluations on multiple splits to report the presence of model biases.
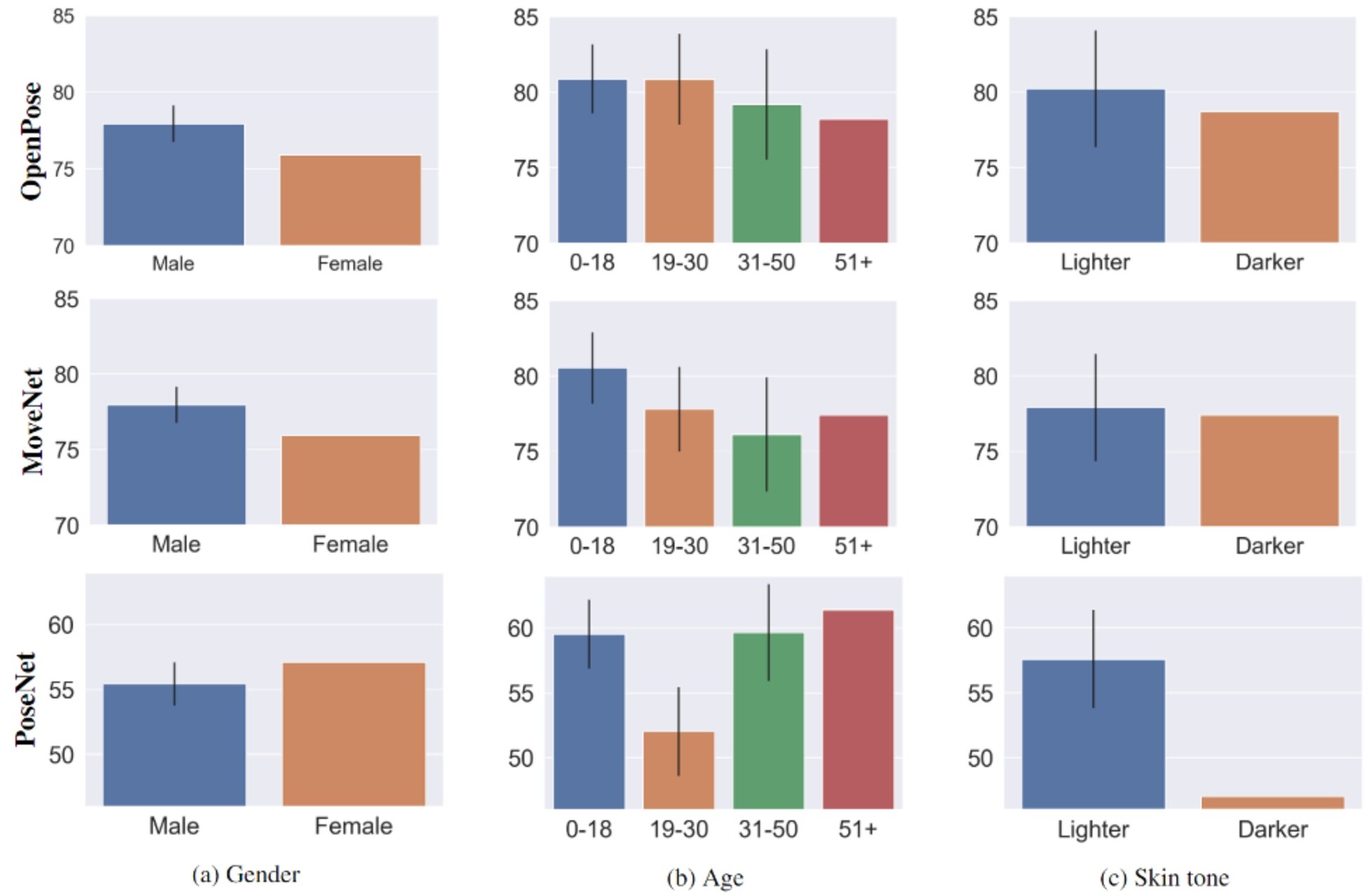
Adjusting sample size for sub-groups on COCO-KD. We subsample sub-groups with a higher number of images (resulting in 223 images for both gender values, 56 images for every age group, and 57 images for both skin tone values). We report the average and standard deviation of the mAP score over 100 different subsamples.
Recommendations towards operationalization of fairness evaluations: Our case study on human pose estimation examined the challenges in operationalizing fairness evaluations in human-centric computer vision tasks. Based on our assessment of some commonly used 2D pose estimation models on our semi-manually annotated dataset, we identified potential model biases and provided recommendations for the operationalization of fairness evaluations as follows:
(i) Data collection should potentially include demographic labels for human-centric datasets to enable fairness evaluations
(ii) Automatic Annotations are not reliable, and it is therefore recommended to rely on self-reported or manual annotations or manual quality checks to ensure the validity of the demographic labels.
(iii) We recommend that data collection efforts are concentrated on building demographically balanced datasets.
(iv) For fairness evaluations on imbalanced demographic data, we recommend reporting results on multiple data splits to confirm the presence of bias
Latest Blog
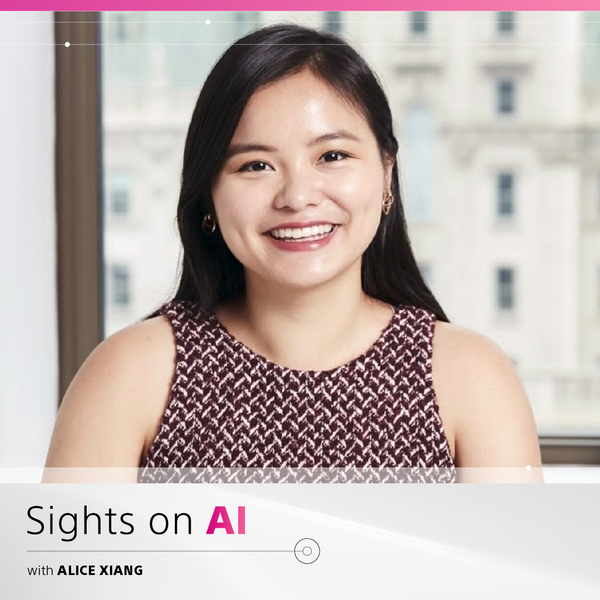
December 13, 2024 | Sony AI
Sights on AI: Alice Xiang Discusses the Ever-Changing Nature of AI Ethics and So…
The Sony AI team is a diverse group of individuals working to accomplish one common goal: accelerate the fundamental research and development of AI and enhance human imagination an…
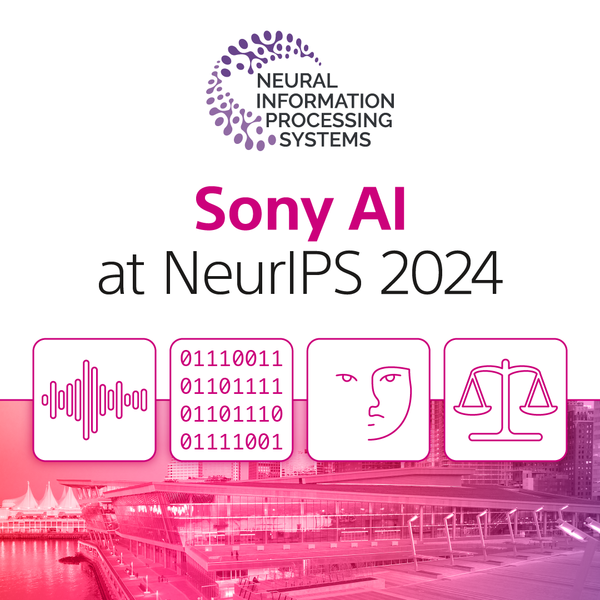
December 13, 2024 | Events
From Data Fairness to 3D Image Generation: Sony AI at NeurIPS 2024
The 38th Annual Conference on Neural Information Processing Systems is fast approaching. NeurIPS 2024, the largest AI conference in the world, is taking place this year at the Vanc…
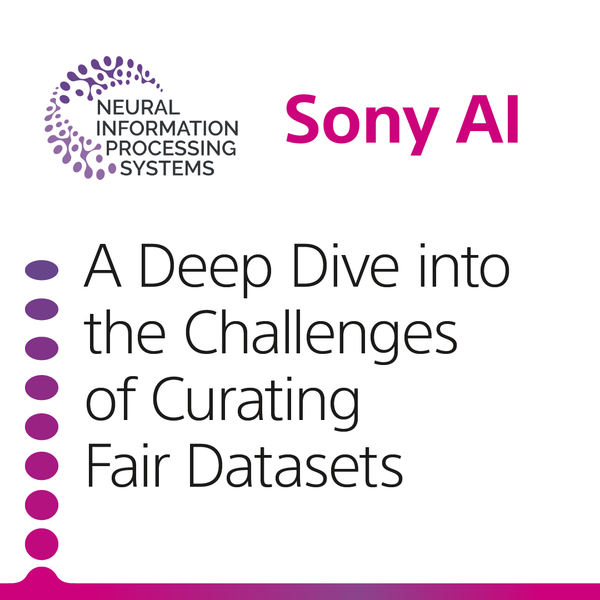
December 4, 2024 | AI Ethics
Exploring the Challenges of Fair Dataset Curation: Insights from NeurIPS 2024
Sony AI’s paper accepted at NeurIPS 2024, "A Taxonomy of Challenges to Curating Fair Datasets," highlights the pivotal steps toward achieving fairness in machine learning and is a …