New Dataset Labeling Breakthrough Strips Social Constructs in Image Recognition
AI Ethics
June 29, 2023
New Dataset Labeling Breakthrough Strips Social Constructs in Image Recognition
The outputs of AI as we know them today are created through deeply collaborative processes between humans and machines. The reality is that you cannot take the human out of the process of creating AI models. This means the social constructs that rule how we interact with the world around us are inherently woven into data used to create AI models. Since the data that makes up an AI model is a byproduct of these human interactions and our inherent social structures and ideas, it is difficult to create a data set that is free from the often brittle constructs and biases that populate how we think, judge and process information.
To change the way data is labeled requires rethinking how the human mind categorizes data. A View From Somewhere (AVFS), new research from Sony AI, created and tested a new way of labeling images, in particular faces. It explores a new method for uncovering human diversity without the problematic semantic labels commonly used, such as race, age, gender, etc. Stripping away the social constructs created by semantic labels allowed judgements that are more closely aligned to raw human perception using similarity and context.
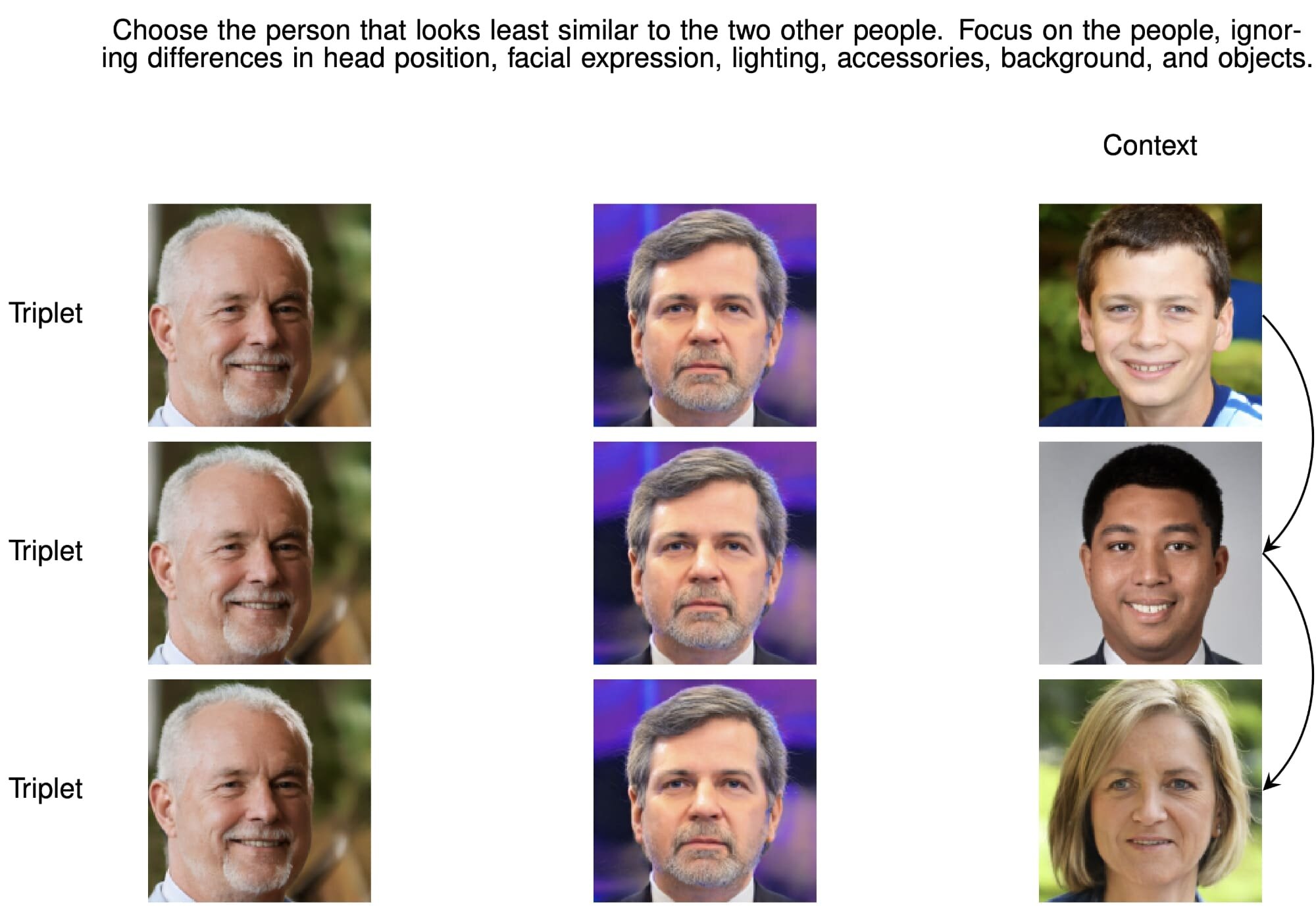
Annotators were tasked with choosing the person that looks least similar to the two other people. Context makes salient context-related properties and the extent to which faces being compared share these properties. By exchanging a face in a triplet (i.e., altering the context), each judgment implicitly encodes the face attributes relevant to pairwise similarity. All face images in the figure were replaced by synthetic StyleGAN3 (Karras et al., 2021) images for privacy reasons; annotators were shown real images.
By removing these sensitive categories, the new approach opened up the potential for more inclusion. Label taxonomies often do not permit multi-group membership, resulting in the erasure, for example, of multi-ethnic or gender non-conforming individuals.
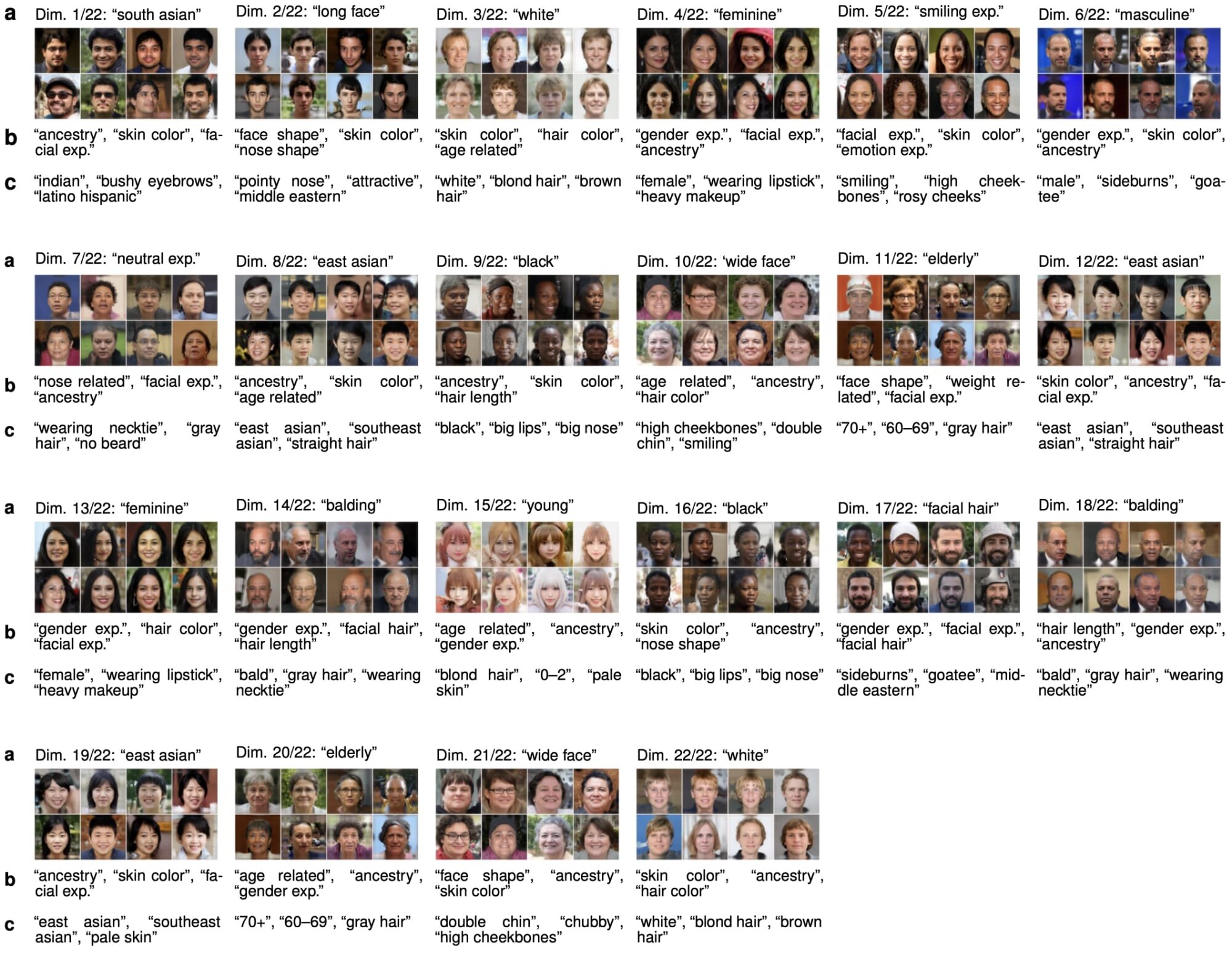
The interpretability of AVFS model dimensions. (a) Our interpretation of the AVFS model dimensions, derived from (b), along with the highest scoring face images along each AVFS dimension. (b) Highest frequency human-generated topic labels for each AVFS model dimension, where the topic labels were obtained from a set of annotators that were allowed to inspect each AVFS dimension. (c) CelebA and FairFace model-generated labels for the face images, which exhibit a clear correspondence with the human-generated topics. Significantly, unlike CelebA and FairFace models, the AVFS dimensions, which largely explain the variation in human judgments, are learned without training on semantic labels. All face images in the figure were replaced by synthetic StyleGAN3 (Karras et al., 2021) images for privacy reasons; annotators were shown real images.
Alice Xiang, Global Head of AI Ethics, Sony Group Corporation, and Lead Research Scientist, Sony AI, and Jerone Andrews, Research Scientist at Sony AI, authored AVFS. The dataset includes 638,180 face similarity judgments over 4,921 faces. Each judgment corresponds to the odd-one-out (i.e., least similar) face in a triplet of faces and is accompanied by both the identifier and demographic attributes of the annotator who made the judgment.
It is the largest dataset of its kind and the first computer vision dataset with additional metadata associated with each judgment: a unique annotator identifier and annotator demographic info (age, gender identity, nationality, and ancestry.) Creating a data set that produces less harm to the image subject and potentially uncovers bias in the annotators labeling the data. To our knowledge, AVFS represents the first dataset, where each annotation is associated with the annotator who created it and their demographics, permitting the additional examination of annotator bias and its impact on AI model creation.
The research was accepted and presented at the 2023 International Conference on Learning Representations. AVFS represents a significant milestone for Sony AI’s work towards ethical human datasets. Data and code are publicly available under a Creative Commons license (CC-BY-NC-SA), permitting noncommercial use cases at https://github.com/SonyAI/a_view_from_somewhere.
We asked Alice and Jerone to share the genesis of this research, its real-world applications, and how it could benefit researchers or practitioners.
What factors motivated the development of the dataset?
Alice Xiang:
When I led the research lab at Partnership on AI, we conducted an interview study of algorithmic fairness practitioners that found a significant challenge faced by these practitioners is a need for more sensitive attribute data to check for bias. There are many privacy and other ethical concerns with collecting such data. In computer vision, there has been a lot of emphasis on the importance of diverse training and evaluation tests to address potential biases.
To check for diversity, though, you need sensitive attribute data—you can’t say if a dataset is gender-balanced if you have no idea what people’s gender identities are. While in certain contexts, the solution then is to find a way to collect sensitive attribute data, this is not always possible or desirable, especially given that labels for gender and racial constructs are highly contestable. Given this context, Sony AI, we wanted to explore alternatives for measuring diversity in large image datasets without knowing the sensitive attributes of the individuals.
Were there any challenges you faced and overcame during the research process?
Jerone Andrews:
One challenge was related to the use of semantic labels. We wanted to avoid relying on problematic semantic labels to capture human diversity, which can introduce biases. This is especially true when inferred by human annotators, and may not accurately reflect the continuous nature of human phenotypic diversity (e.g., skin tone is often reduced to “light” vs. “dark”). We used a novel approach of face similarity judgments to overcome this challenge to generate a continuous, low-dimensional embedding space aligned with human perception. This approach allowed for a more nuanced representation of human diversity without relying on explicit semantic labels. Moreover, our approach permitted us to study bias in our collected dataset, which was the result of the sociocultural backgrounds of our annotators. This is important because computer vision researchers rarely acknowledge the impact an annotator’s social identity has on data.
Can you describe real-world applications for this dataset and how it could benefit researchers or practitioners?
Jerone Andrews:
Our dataset has several use cases that can benefit researchers and practitioners. First, it can be used for learning a continuous, low-dimensional embedding space aligned with human perception. This enables the accurate prediction of face similarity and provides a human-interpretable decomposition of the dimensions used in the human decision-making process. Thus, providing insights into how different face attributes contribute to face similarity, which can inform the development of more interpretable and explainable models.
Second, the dataset implicitly and continually captures face attributes, allowing the learning of a more nuanced representation of human phenotypic diversity compared to datasets with categorically labeled face attributes. This enables researchers to explore and measure the subtle variations of faces, particularly the similarity between faces that belong to the same demographic group.
Third, the dataset facilitates the comparison of attribute disparities across different datasets. By examining the variations in attribute distributions, researchers can identify biases and disparities in existing image datasets, leading to more equitable and inclusive data practices. This is particularly relevant in ethics and fairness, where mitigating biases in AI systems is crucial.
Fourth, the dataset provides insight into annotator decision-making and the influence of their sociocultural backgrounds, underscoring the importance of diverse annotator groups to mitigate bias in AI systems.
More generally, the dataset can be used for tasks related to human perception, human decision-making, metric learning, active learning, and disentangled representation learning. It provides a valuable resource for exploring these research areas and advancing our understanding of human-centric face image analysis.
How might this dataset enable interdisciplinary collaborations or cross-disciplinary research projects?
Jerone Andrews:
Our dataset facilitates interdisciplinary collaborations and cross-disciplinary research projects by offering an alternative approach to evaluating human-centric image dataset diversity and addressing the limitations of demographic attribute labels. It allows researchers from computer vision, psychology, and sociology to collaborate.
Our dataset gives means to learning a face embedding space aligned with human perception, enabling accurate face similarity prediction and providing a human-interpretable decomposition of the dimensions used in human decision-making. These dimensions relate to gender, ethnicity, age, face morphology, and hair morphology, allowing for the analysis of continuous attributes and comparison of datasets and attribute disparities.
Including demographic attributes of annotators also underscores the influence of sociocultural backgrounds, emphasizing the need for diverse annotator groups to mitigate bias. Thus, researchers from various disciplines can utilize this dataset to investigate face perception, diversity, biases, and social constructs, fostering interdisciplinary collaborations and advancing cross-disciplinary research projects.
What broader implications or societal impacts could result from using this dataset in research or applications?
Alice Xiang:
We hope the dataset and the techniques we develop in this paper can enable practitioners to measure and compare the diversity of datasets in contexts where collecting sensitive attribute data might not be possible or desirable. In doing so, we hope practitioners will be more conscious of the potential biases their models might learn and take active measures to prevent them.
Latest Blog
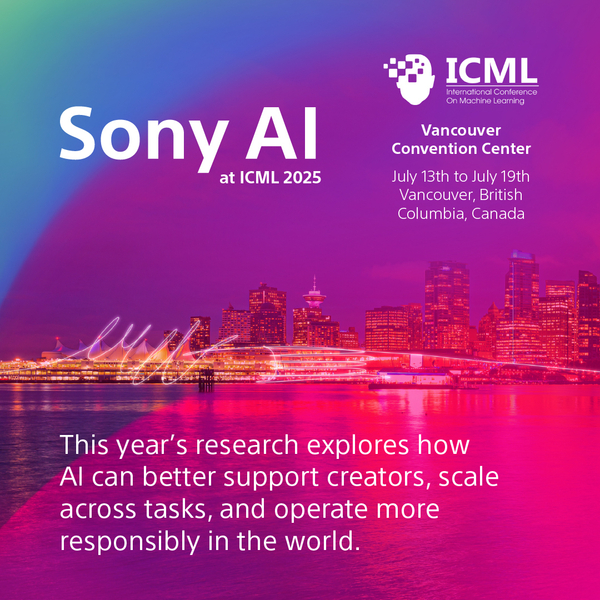
July 14, 2025 | Events, Sony AI
Sony AI at ICML: Sharing New Approaches to Reinforcement Learning, Generative Mo…
From powering creative tools to improving decision-making in robotics and reinforcement learning, machine learning continues to redefine how intelligent systems learn, adapt, and s…
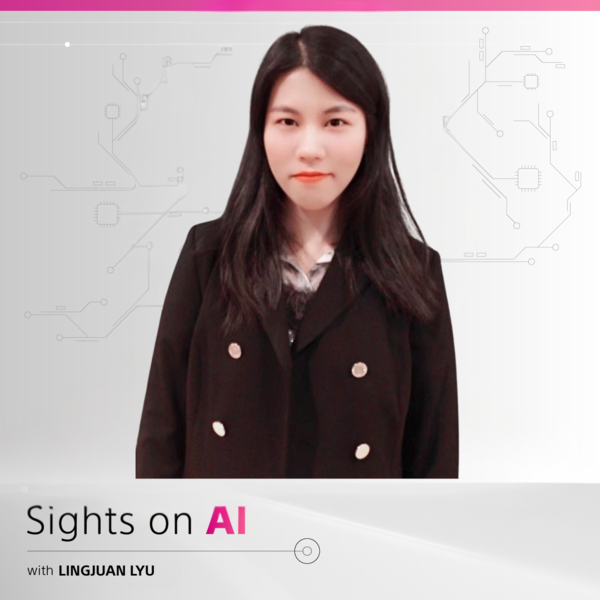
July 8, 2025 | Sony AI
Sights on AI: Lingjuan Lyu Discusses Her Career in Privacy-Preserving AI and Sta…
The Sony AI team is a diverse group of individuals working to accomplish one common goal: accelerate the fundamental research and development of AI and enhance human imagination an…
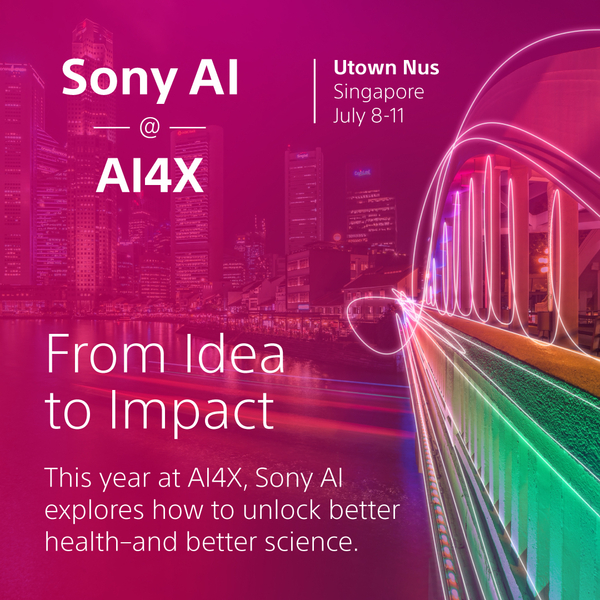
July 7, 2025 | Scientific Discovery, Events
Scientific Discovery: How Sony AI Is Building Tools to Empower Scientists and Pe…
At this year’s AI4X 2025, Sony AI is presenting two research projects that show how intelligent systems can augment knowledge and creativity in complex scientific research. These t…