Training the World’s Fastest Gran Turismo Racer
Insights into building an AI agent that can achieve superhuman lap times
Gaming
GT Sophy
June 14, 2022
GT SOPHY TECHNICAL SERIES
Starting in 2020, the research and engineering team at Sony AI set out to do something that had never been done before: create an AI agent that could beat the best drivers in the world at the PlayStation® 4 game Gran Turismo™ Sport, the real driving simulator developed by Polyphony Digital. In 2021, we succeeded with Gran Turismo Sophy (GT Sophy). This series explores the technical accomplishments that made GT Sophy possible, pointing the way to future AI technologies capable of making continuous real-time decisions and embodying subjective concepts like sportsmanship.
Incredible speed and near-perfect control are two elements any driver needs to win a racing event. When we built AI agent GT Sophy, we needed to train it to master those skills within the world of the PlayStation game Gran Turismo, just as a human driver would. And we had to ensure that GT Sophy could apply those capabilities while racing different cars on various tracks. Achieving that blend of speed and control was one of the first steps in creating an agent that could beat the world’s best Gran Turismo drivers. This post offers an inside look at the pivotal steps for developing an agent capable of record-breaking lap times.
How does GT Sophy see the world?In training an agent to drive fast in Gran Turismo, it is important to understand which attributes of the game are relevant to achieving this goal. From interactions with the game developers at Polyphony Digital and a former top Gran Turismo racer, as well as from internal team tests, we captured a set of relevant features, including vehicle velocity, acceleration, orientation, load on the tires, and whether the vehicle is off the track.
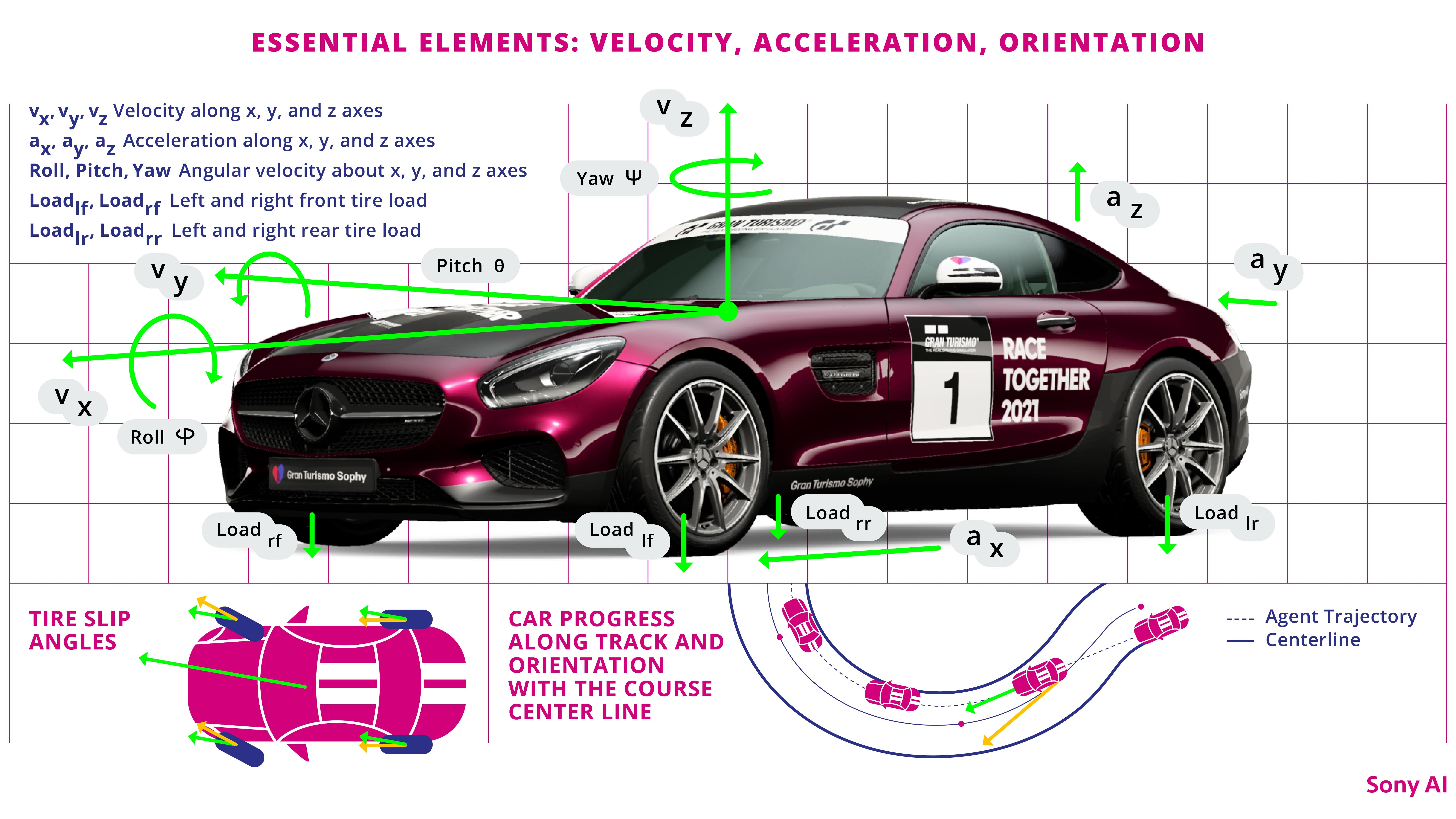
The relevant features also included the shape of the track, specifically the sections immediately in front of the car.
Left: The magenta dots represent the agent’s view of the track. When GT Sophy nears the hairpin turn, it slows down—and its view decreases. Right: GT Sophy navigates the stretch of track depicted on the map.
The agent sees the course ahead as a series of discrete points, up to six seconds from its current position. The distance ahead is calculated based on the speed of the car. The faster the agent, the farther it can see; the slower it is, the shorter the range of points it takes into account. When the car approaches a hairpin turn, you can see the points collapse as the vehicle slows down and the view ahead decreases. The agent uses these points to orient itself with respect to the left, center, and right track lines and find the best path through a sequence of curves. This is similar to how experienced drivers memorize the track layout through numerous hours of practice and learn to optimize the trajectory of the car.
When we combine features that help the agent localize itself on the track with car-related information (tire load, tire slip), the agent is able to execute precise maneuvers to optimally navigate a variety of turns. An example of the utility of these features is apparent in the latter half of the Dragon Trail Seaside track. The car drifts through a series of left and right turns, using as much of the curb as possible while maintaining control with a minimal loss of speed.
We partnered with Sony Interactive Entertainment and gained access to more than a thousand PlayStation consoles in the cloud.
What does GT Sophy prioritize?Once the agent is able to perceive the world around it, it needs to understand the elements of driving fast and the actions that lead to it. It would be difficult to engineer the precise braking and steering points, as well as the driving lines to follow, in a complex dynamical domain such as Gran Turismo. Instead, we define a basic set of objectives and use a Reinforcement Learning (RL) algorithm so the agent can automatically learn a behavior that optimizes the lap time. In RL terminology, we refer to this as the agent’s reward function. Driving well in Gran Turismo has multiple objectives, such as maximizing speed and adhering to the racing rules, which include avoiding barrier contact and staying on the track. These are defined in a way where the agent can reason about the tradeoffs between them and avoid exploiting any potential loopholes, for example cutting corners to gain time. The objective function consists of four components:
Progress: The agent is rewarded as it covers more distance in its decision cycle, 0.1s in this case (when operating at 10Hz). The distance covered is measured along the track centerline. The more distance the agent covers, the higher the reward.
Barrier contact penalty: The agent is penalized for every timestep it spends in contact with a fixed barrier. The penalty depends on the speed of the car to discourage it from making contact at high speeds.
Off-track penalty: The agent faces a penalty for every timestep it spends with more than two tires off the tarmac or curbs. This penalty also depends on the speed of the car to discourage it from going off the track at high speeds.
Without the off-track penalty, an agent might learn to cut corners, as depicted here on the Circuit de la Sarthe track (which is modeled on the famous route used in the 24 Hours of Le Mans):
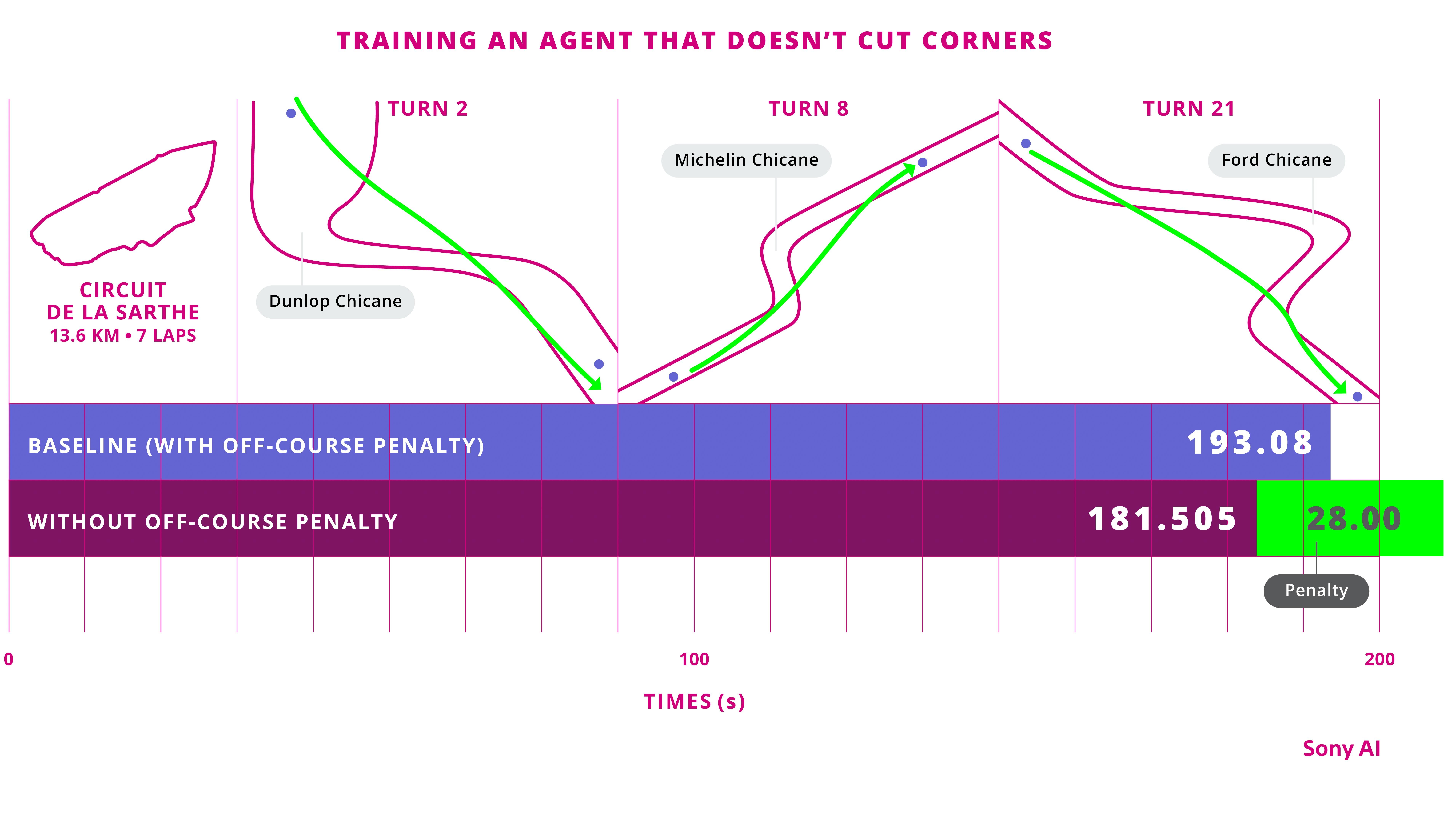
The chart shows the difference in learned lap times without the off-track penalty, using the Red Bull X2019 Competition car. The vehicle goes faster by ignoring the track limits, but doing so would cost it an in-game penalty of 28 seconds.
These reward signals play a significant role in shaping the learned behavior. While these should be sufficient for the agent to learn from, it is often helpful to provide additional signals to speed up the learning process. In that regard, we include:
Tire slip penalty: The agent is penalized for every timestep its tires begin to slip, skid, or slide. The penalty takes into account all four tires and is computed based on the tire slip angle and tire slip ratio.
Using these components, the agent is tasked with learning how to maximize progress and minimize the penalties based on how it perceives the world around it.
In a time trial, GT Sophy had the opportunity to race some of the best Gran Turismo drivers in the world.
How did we train GT Sophy?The agent can control the car in Gran Turismo using two continuous actions, one for throttling or braking and another for steering. GT Sophy has to explore the world and learn the effects of these actions in different situations.
Training to drive in Gran Turismo takes place in real time, as the game speed cannot be modified. Under this condition, we would need data from multiple instantiations of the game, running in parallel, to be able to provide GT Sophy the experiences relevant to high-speed driving in a reasonable amount of time.
To facilitate training GT Sophy on a large scale, we partnered with Sony Interactive Entertainment and gained access to more than a thousand PlayStation consoles (PS4s) in the cloud. To train a time trial agent, we used 10 PS4s, each running the game with 20 cars in a race evenly spaced around the track.
We train GT Sophy using a novel reinforcement learning algorithm called Quantile Regression Soft Actor-Critic that we developed at Sony AI. The algorithm helps to better model the distribution of possible outcomes in a complex domain like Gran Turismo. (Technical details on the method are available in the Nature article.)
Once an experiment begins, the agent learns to drive through trial and error. Understandably, it struggles at the start. With an hour of training, the agent is able to complete laps, albeit slowly and carefully. This progression continues, and after eight hours of training, the agent is completing laps at times similar to the default AI built into the game. After a day, the agent is near the top 10 percent quantile of the best human players. It takes another eight to 10 days of training to shave off the last few seconds and milliseconds and reach consistent superhuman performance without any racing penalties.
GT Sophy makes rapid progress in training. It struggles at the start, but after a single day of training, the agent is near the top 10 percent of the best human players.
GT Sophy makes rapid progress in training. It struggles at the start, but after a single day of training, the agent is near the top 10 percent of the best human players.
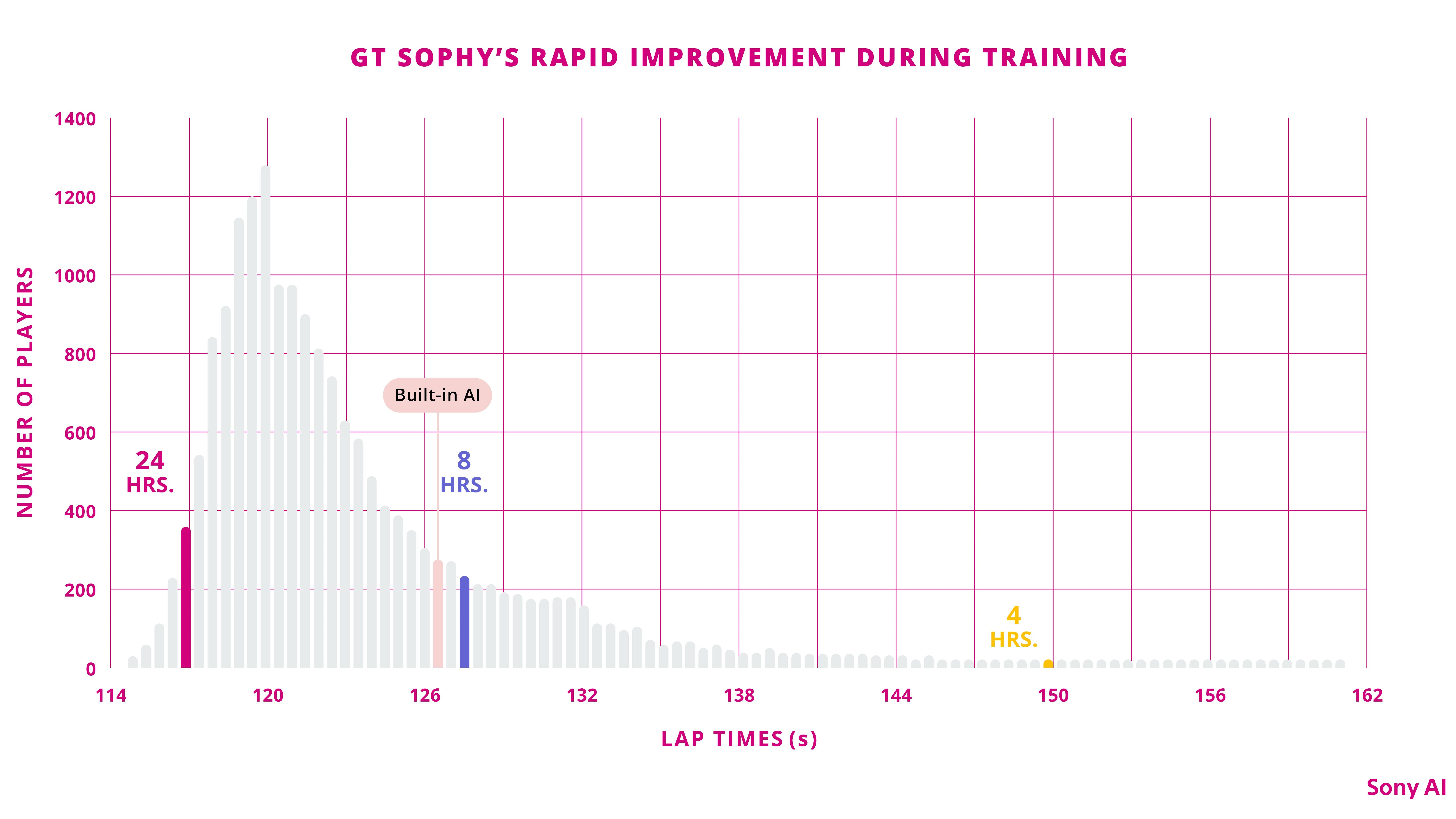
After 24 hours of training, GT Sophy can beat all but a few hundred of the best Gran Turismo racers on the Lago Maggiore GP course.
To highlight the level of consistency: after completing 200 laps, the agent in a Porsche 911 car on the Lago Maggiore GP track had a best lap time of 114.071s, a mean lap time of 114.208s, and a standard deviation of 0.061s. The best time ever logged by a human driver was 114.181 by Valerio Gallo in August 2021.
Pushing GT Sophy to the limitsWith the training procedure set up, it was time to test GT Sophy against humans. We had the opportunity to benchmark the agent against some of the best Gran Turismo drivers in the world—Emily Jones, Valerio Gallo, and Igor Fraga—in a time trial challenge. Each driver raced against a “ghost” version of GT Sophy; in other words, a representation of the AI-controlled car appeared on the track as a speed reference but had no impact on the race (the human drivers could actually drive right through it). In that challenge, the humans were given the chance to drive multiple laps, in a 30-minute period, to beat the AI lap time.
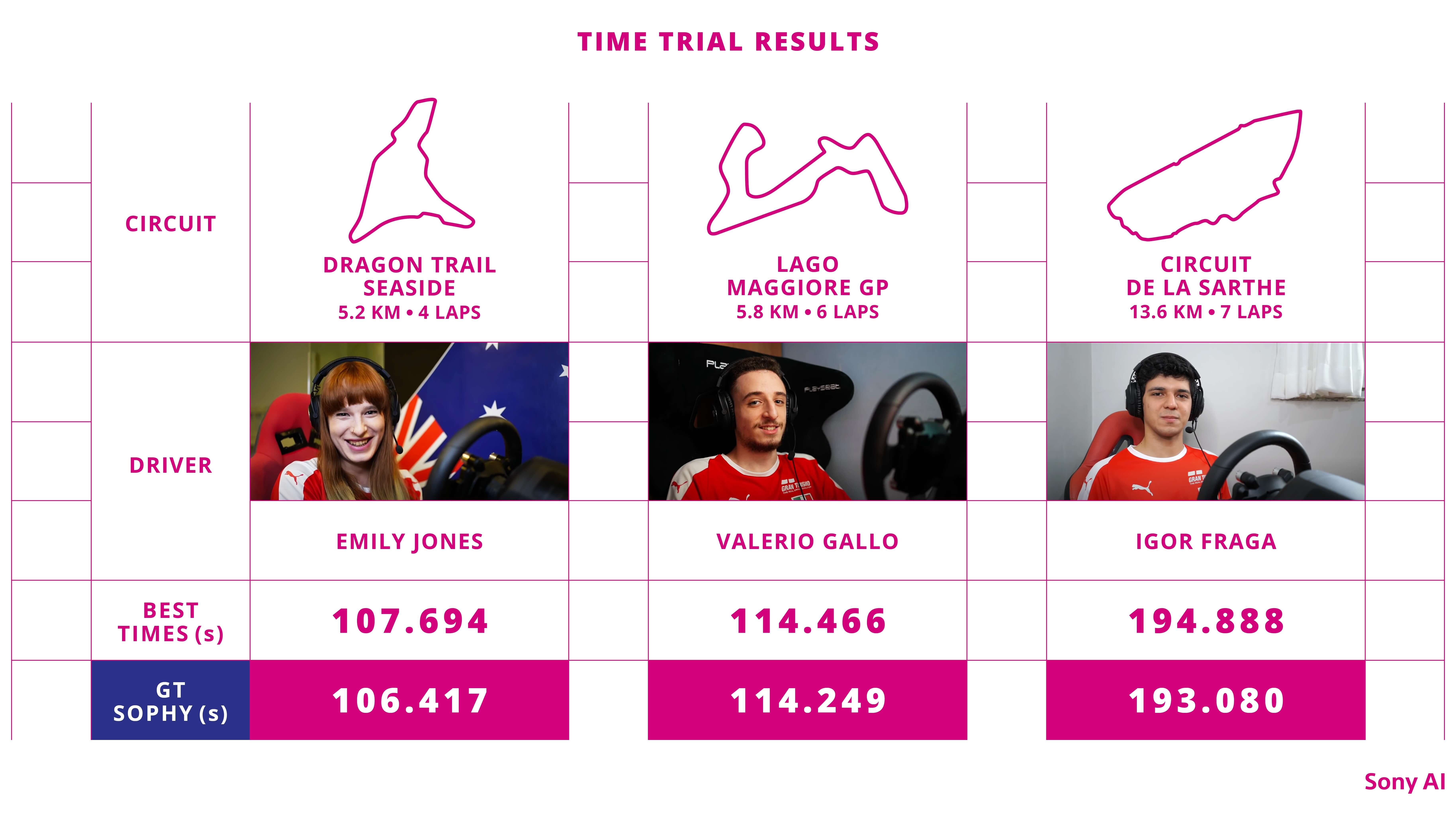
In time trials, GT Sophy outraced three of the world’s fastest Gran Turismo drivers.
GT Sophy won by more than a second on the Dragon Trail Seaside and Circuit de la Sarthe tracks and managed to beat Valerio Gallo on Lago Maggiore GP, despite his slight lead on GT Sophy for the first half of the lap on his 26th attempt.
The results were compelling and showed us that we were able to train the fastest agent to race three specific cars on three Gran Turismo tracks. The question we then asked ourselves: would our approach work for another track-car pair, or have we optimized training and overfit to these preselected combinations?
To answer this question, we attempted to tackle the Lewis Hamilton Time Trial Challenge DLC available in Gran Turismo. The challenges involve pitting players against seven-time F1 world champion racing driver Lewis Hamilton, who is also a Gran Turismo ambassador. Hamilton provided the game developers with his best laps on 10 tracks with two cars (Mercedes-AMG GT3 and the Sauber Mercedes C9). We chose the Nürburgring Nordschleife and Sauber Mercedes C9 track-car pair. The track is one of the longest in the game at 20.8km (12.9 miles). It has more than 150 corners, 300m (1,000 feet) of elevation change in a lap, and is considered to be one of the hardest in the world.
Hamilton’s reported lap time in the game is 5:40.622. The best time logged by a Gran Turismo racer, Igor Fraga, is 5:26.682. GT Sophy, trained with the approach described earlier, demonstrated a lap time of 5:22.975, nearly four seconds faster than the best human time and 17 seconds faster than Hamilton. (The full lap can be viewed here.) The result showed that our training approach, including the choice of input features, reward functions, and data generated from the PS4s, can be used on other challenging tracks in the game to achieve superhuman performance.
In one time trial challenge, GT Sophy beat the best time ever logged by a human driver. It also raced faster than seven-time Formula 1 world champion driver Lewis Hamilton, who is a Gran Turismo ambassador.
Building the fastest Gran Turismo racer in the world has been a long and fruitful journey. We drew on previous work and pushed the limits that extra mile. We believe this work will enhance Gran Turismo racers’ experience by providing a new target for them to aim at, challenging them to improve their skills and learn new and innovative ways to race. Looking ahead, there are several open challenges related to speed and control. How can we optimize tire wear and fuel consumption in time trials? What is the best driving strategy for different weather conditions? The team at Sony AI is continuing research efforts along various threads as we push the boundaries of high-speed Gran Turismo racing.
Latest Blog
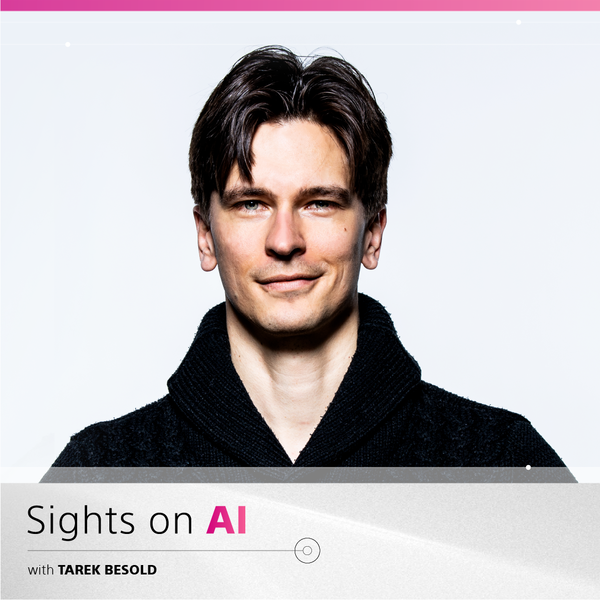
June 18, 2024 | Sony AI
Sights on AI: Tarek Besold Offers Perspective on AI for Scientific Discovery, Ba…
The Sony AI team is a diverse group of individuals working to accomplish one common goal: accelerate the fundamental research and development of AI and enhance human imagination an…
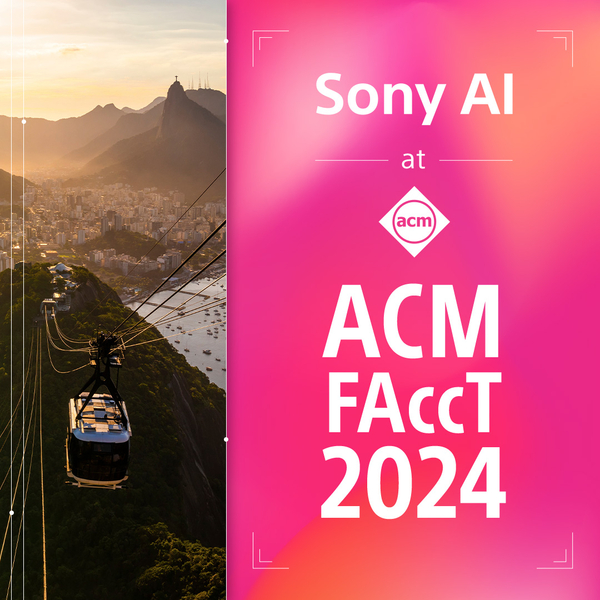
June 4, 2024 | Events , Sony AI
Not My Voice! A Framework for Identifying the Ethical and Safety Harms of Speech…
In recent years, the rise of AI-driven speech generation has led to both remarkable advancements and significant ethical concerns. Speech generation can be a driver for accessibili…
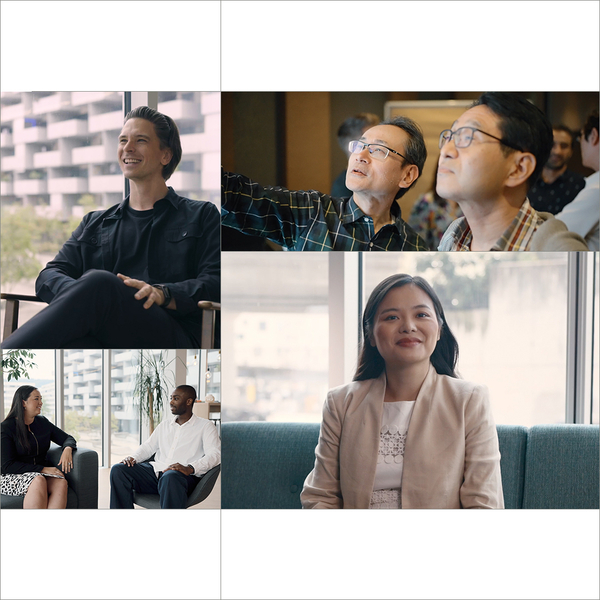
May 22, 2024 | Sony AI
In Their Own Words: Sony AI’s Researchers Explain What Grand Challenges They’re …
This year marks four years since the inception of Sony AI. In light of this milestone, we have found ourselves reflecting on our journey and sharpening our founding commitment to c…