Ushering in Needed Change in the Pursuit of More Diverse Datasets
AI Ethics
July 27, 2024
Sony AI, Research Scientist, Jerone Andrews’ paper, "Measure Dataset Diversity, Don't Just Claim It", has won a Best Paper Award at ICML 2024. This recognition is a testament to the groundbreaking work being done to improve the reliability and validity of machine learning datasets.
Tackling the Complexity of Dataset Diversity
One of the major challenges in machine learning research is the reproducibility crisis. The lack of clear definitions and standardization in data collection often leads to validation and replication issues. Our research advocates for a more precise approach to dataset construction, which is essential for addressing these challenges. By enhancing transparency, reliability, and reproducibility in machine learning research, we offer broader enhancements to scientific practices.
Our paper addresses the complex issue of dataset diversity, a critical characteristic often cited but seldom rigorously defined or measured. Achieving true diversity requires more than broad claims; it demands precise definitions and robust validation methods. By improving how we define and measure dataset diversity, we can develop more robust and contextually appropriate datasets. This, in turn, can lead to more reliable machine learning models trusted in critical applications.
The Need for Clear Definitions and Robust Validation
Our paper emphasizes the importance of clear definitions and robust validation methods to ensure that datasets genuinely embody the qualities they claim. The research calls for a systematic approach to defining diversity, ensuring that it is not merely a buzzword but a measurable and verifiable property. By applying principles from measurement theory, the paper provides a structured approach to conceptualizing, operationalizing, and evaluating dataset diversity.
Applying Measurement Theory to Dataset Diversity
Measurement theory, widely used in the social sciences, offers a framework for developing precise numerical representations of abstract constructs. In the context of machine learning datasets, this means defining diversity in concrete terms, identifying relevant indicators, and developing methods to measure these indicators accurately. The paper outlines a detailed process for applying measurement theory to dataset diversity, ensuring that datasets are not only diverse but also reliable and valid:
-
Conceptualization: Defining what constitutes diversity within the context of the dataset. "For dataset creators, this phase resembles the translation of abstract values, such as diversity, into tangible and concrete definitions."
-
Operationalization: Developing concrete methods to measure these dimensions. This involves the meticulous development of methodologies to empirically measure abstract concepts.
-
Evaluation: Ensuring the reliability and validity of the diversity measures. Reliability “concerns the consistency and dependability of measurement”, whereas validity centers on “determining whether the final dataset aligns with theoretical definitions”.
Key Contributions to Machine Learning Practices
The paper's contributions are significant not only for dataset creators but also for reviewers and the broader machine-learning community. Key contributions include:
- A thorough review of 135 image and text datasets, examining how diversity is defined and operationalized.
- Practical recommendations for dataset creators to provide concrete definitions of diversity and align these with clear operational measures.
- Strategies for evaluating the reliability and validity of datasets, ensuring that claimed properties such as diversity are accurately represented.
Conclusion
The recognition of "Measure Dataset Diversity, Don't Just Claim It" at ICML 2024 underscores the critical importance of diversity in machine learning datasets. By addressing the challenges of conceptualizing, operationalizing, and evaluating dataset diversity, Jerone Andrews and his collaborators have made a significant contribution to the field.
As we continue to explore the complexities of AI and machine learning, contributions like these pave the way for a more rigorous and scientifically grounded approach to developing and evaluating datasets. Congratulations to the authors Dora Zhao, Jerone Andrews, Orestis Papakyriakopoulos, and Alice Xiang for their well-deserved award.
Latest Blog
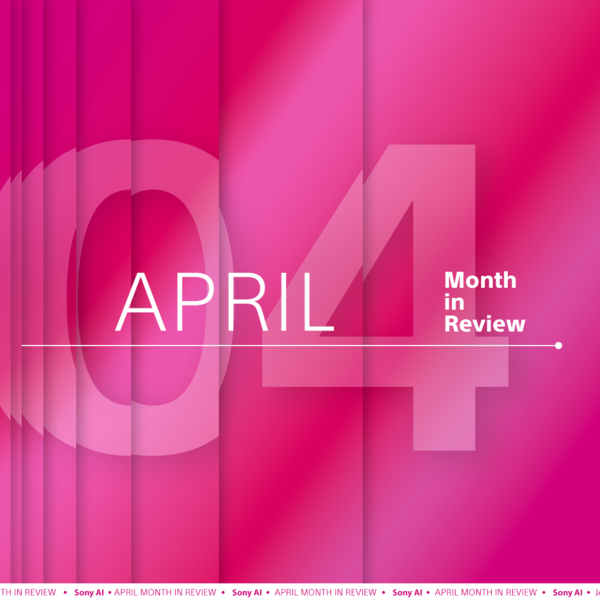
May 1, 2025 | Sony AI
Advancing AI: Highlights from April
April marked a standout moment for Sony AI as we brought bold ideas to the world stage at ICLR 2025. This year’s conference spotlighted our work across generative modeling, multimo…
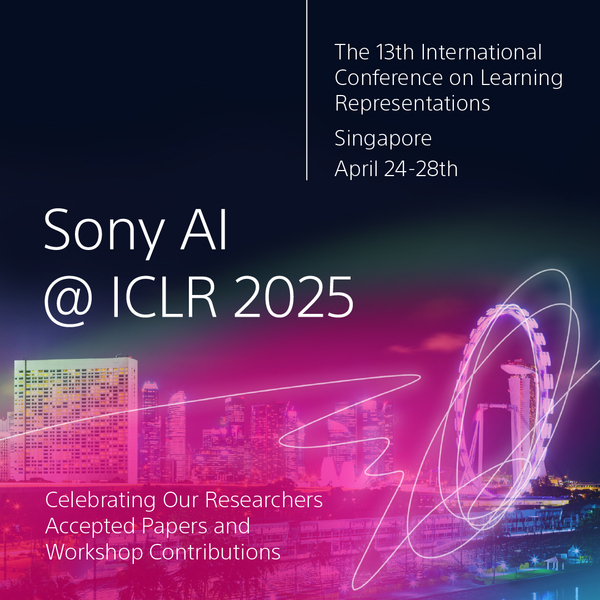
April 23, 2025 | Events, Sony AI
Sony AI at ICLR 2025: Refining Diffusion Models, Reinforcement Learning, and AI …
This April, Sony AI will present a wide-ranging portfolio of research at the International Conference on Learning Representations (ICLR 2025). From progressing text-to-sound and vi…
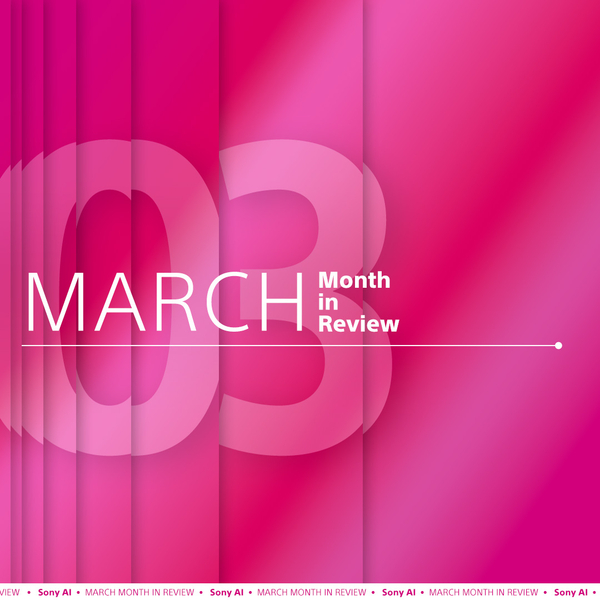
April 1, 2025 | Sony AI
Advancing AI: Highlights from March
March was a month of notable advancements, global discussions, and strategic collaborations, reinforcing our commitment to responsible AI innovation. From pioneering multi-modal AI…