When Privacy and Fairness Collide: Reconciling the Tensions Between Privacy and Representation in the Age of AI
AI Ethics
March 26, 2024
Invisibility is sometimes thought of as a superpower. People often equate online privacy with selective invisibility, which sounds desirable because it potentially puts them in control of how images of their faces are used. As legitimate concerns about privacy and mass surveillance grow, the desire to be “unseen” or invisible is becoming an imperative topic of discussion. We will explore some real-world examples of being “mis-seen” in this post to bring this debate to life.
It can present a serious problem when the systems that depend on facial recognition do not recognize us or “mis-see” who we are because of challenges such as insufficient data and hidden biases. Reducing these hidden biases often means significantly increasing the diversity in training datasets — possibly including images of your face, body, and other personal data — to mitigate hidden bias.
That’s why advances in facial recognition and computer vision technologies have created a new tension between the need for privacy and the need for representative, fair, and unbiased data. In some ways, it seems like an impossible paradox – how can you be confident that you’ll be recognized accurately and fairly by facial recognition systems regardless of skin tone or gender while at the same time not feeling that your privacy is being taken away?
In a recent “SXSW Fireside Chat - Ethics in an AI World: Better to be Seen or Unseen?” (March 13, 2024), we explored whether it is better to be “unseen” (unrepresented in a system), “mis-seen” (incorrectly represented in a system), or “seen” by the system and accept some potential loss of personal privacy. We also discussed several different approaches for addressing this paradox.
The tech industry, policymakers, and the public must find ways to value both privacy and representation. This discussion considers these tensions and how AI, facial recognition, and other related technologies can be developed to preserve privacy and enhance representation. Michael Spranger, Sony AI President, spoke with Eliza Strickland, Senior Editor for IEEE Spectrum, about navigating these crosscurrents and the efforts of the Sony AI Team to raise awareness and address these issues.
A touchstone of this discussion is an article for the Harvard Journal of Law and Technology, "Being ‘Seen’ Versus ‘Mis-Seen'; Tensions Between Privacy and Fairness in Computer Vision,” by Sony’s Global Head of AI Ethics, Alice Xiang. This post summarizes key findings and suggestions in this paper. As Xiang explains in her article, at this time, many of the potential harms caused by being mis-seen have no legal remedies, but “together they amount to being treated as a second-class citizen, living in a world that cannot detect or recognize you.”
Picture This - The Paradox of Privacy and Fairness
For human-centered computer vision systems (HCCVs) to be as accurate and broadly representative as possible, large and diverse collections of images of people in different contexts are crucial. The problem is that obtaining so many pictures at scale presents serious privacy and policy risks. Without sufficiently diverse training data, these systems may become skewed and biased.
To Be or Not to Be: Being "Seen" vs. "Mis-Seen"
Addressing the challenges of privacy and fairness in the context of AI and HCCV must begin by clearly defining and distinguishing the key terms and concepts used in the discussions. While concerns about maintaining privacy from potentially invasive technologies are nothing new, novel issues and concepts are emerging.
For example, systems such as facial recognition software on your phone, payment systems, or even security monitors at your job should work just as well for you and others, regardless of skin tone or gender. Misrecognition due to inadequate representation in the training data can lead to various daily inconveniences, such as your phone’s inability to recognize who you are. More seriously, facial identification technologies used by law enforcement must be fair, representative, and accurate to preserve equal justice for all citizens. In that sense, it’s crucial to be clearly “seen.” The opposite of “seen” in this respect is not “invisible” but “mis-seen,” which is not being detected, being misrecognized as someone else, someone else being misrecognized for you, or having images/videos of you misclassified or mischaracterized. The root causes of being mis-seen stem from inherent and unintended biases created from insufficient data about different groups and models that fail to account for key real-world differences among people.
These distinctions are not theoretical and can have serious, real-world repercussions today. Consider the societal and individual impacts of being mis-seen by AI because of inadequate representative data about skin tones. Further, consider this issue in the context of law enforcement, where misidentification can have serious consequences now. Take the case of Robert Williams, who was wrongfully arrested due to a faulty facial recognition match. In 2020, Detroit police arrested Mr. Williams for allegedly stealing thousands of dollars worth of watches. Using a facial recognition service, the police matched grainy surveillance recordings of the crime to his driver’s license. In fact, he was driving home from work at that time and not near the crime scene. Williams later said of the technology that led to his false arrest, “It’s dangerous when it works and even more dangerous when it doesn’t work.” He noted that in his situation, “...the Detroit police were supposed to treat face recognition matches as an investigative lead and not as the only proof they need to charge someone with a crime.”
This story not only points out the ways surveillance technologies can go wrong, with potentially harmful, real-world consequences, but it also highlights the fact that the way the technology is intended to work is not necessarily how it will be used. Does all this mean you need to give up on the idea of personal privacy to avoid being miss-seen? No.
There are possible solutions to this complicated challenge.
How to Be Seen on Your Terms
So far, you might think this is an insurmountable challenge, but there are solutions. In her paper, Xiang presents a highly detailed discussion of these issues and offers some potential solutions. While Xiang notes that these ideas are not perfect or complete, they offer the necessary scaffolding for a workable framework.
Solution - Targeted exemptions or “carve-outs” for some facial recognition and related technologies that would foster fairness while preserving privacy as much as possible.
This may seem impossible, but a nuanced approach can make a difference. For example, larger and more diverse sets of facial data might be used to help teach the systems to make them fairer and more accurate. Still, those images could not be later used in the deployment of these systems, which is where there can be a more significant potential for privacy harm to someone directly interacting with the system.
Solution - Increasing participatory design can help improve fairness.
That is, engaging with stakeholders who use or are affected by facial recognition technology to design and deploy systems that strike a better balance. Among the key challenges is a lack of incentive for communities to contribute their personal information to AI training sets. There may be good reasons to do so, including helping with the fairness issues discussed here. Additionally, it may be a way to help preserve the history of a family or community. There are understandable reasons why many people would be wary of sharing their data. The incentives may not outweigh the concerns. Even so, these participatory design efforts can help feed into and inform other ways to reconcile personal privacy and fairness.
Solution - Building trust with third-party collection is another complementary approach to enhancing trust and fairness for AI data training sets.
Rather than having private companies with unclear or debatable motivations for collecting and using your data, there could be more potentially trusted and transparent third-party entities. These entities could either be governmental or not governmental and do not have the same financial responsibilities and operate on different priorities and constraints. While this approach might be able to help, it can’t resolve the problems by itself. These entities will take time to create and will still need to confront some of the fundamental challenges described here.
Solution - Advances in technology can play a role in addressing the challenges laid out in this article.
Privacy-preserving technologies and synthetic image generation may be helpful. Privacy-preserving tech includes image pixelization and blurring, but that approach doesn't guarantee that the image cannot be reverse-engineered back to its original state. Further, the process of face blurring itself requires steps that require even more biometric data, creating another privacy paradox loop. Although privacy-preserving technologies have significant limitations, it still can be part of the mix if used thoughtfully. Another approach that has some promise is synthetic image generation, which can create images of people who are not real or of people who are real and put them in different contexts. This approach could be used as part of AI training sets. That way, at least in theory, it could provide a more robust and representative set of examples without using as many images as possible of real people, which in turn would presumably help improve privacy overall. As you might expect, this approach is not a panacea. It has many potential drawbacks, including the potential for introducing more bias into the data pipeline and the fact that artificial images are artificial and, therefore, will not necessarily capture the real world as fully or accurately as needed.
The right against being “mis-seen” is the last solution considered in the Harvard Law Review paper. Currently, there are no legal protections for being “mis-seen” in many of the ways described here unless it is recognized and covered by the laws as harmful.Instances of inconvenience, indignation, or embarrassment likely have no legal protection. As instances of being mis-seen pile up for a person or group of people, it can have a significant, cumulative negative impact at a personal and societal level.
One way to look at legal protections against being mis-seen is degraded product performance. If a product does not perform as well for some people, perhaps because of inherent skin tone biases, that might be a product liability issue. Intuitively, that makes sense, but there are many limitations to the existing product liability doctrine, making it difficult, if not impossible, to apply. The full scope of these limitations is beyond the scope of this post but is addressed in detail in the research. The bottom line, however, is that legal protections, while they may be part of the solution, have a long way to go and may never be able to meet the challenges of being mis-seen on their own.
All these ideas and approaches aim to prevent mis-seeing rather than merely ensuring invisibility to AI systems. Invisibility may seem like a good thing — if not a superpower — but as we have seen, it can mean not being recognized or represented in many important ways. The challenges and paradoxes seem daunting, but facial recognition, computer vision, and AI will likely be an increasing and vital element in our world. The solutions will need to come in many forms and require a lot of thoughtful policy and technology planning and implementation. It's going to be a process, with the essential goal of you having a right to have the ability to have the level of privacy you want while still being represented and accurately and fairly.
Join the Discussion from SXSW!
If this post whetted your appetite to learn more and get directly involved, please listen to the recording of the discussion. We encourage you to discuss this issue and follow us on our blog, X, Instagram, and LinkedIn. Again, here‘s the link to the full paper - Being ‘Seen’ Versus ‘Mis-Seen”; Tensions Between Privacy and Fairness in Computer Vision”, by the Global Head of AI Ethics, Alice Xiang can be read here.
Conclusion
The future of AI and surveillance technologies has not yet been written, but the outcomes may depend on the current steps. The stakes are high for creating a world prioritizing personal privacy choices and fair representation. We hope you stay tuned and get involved in these conversations as we work towards solutions.
More to Explore:
- Blog: Beyond Skin Tone: A Multidimensional Measure of Apparent Skin Color – Sony AI
- Blog: New Dataset Labeling Breakthrough Strips Social Constructs in Image Recognition – Sony AI
- Blog: Exposing Limitations in Fairness Evaluations: Human Pose Estimation – Sony AI
- Research: Beyond Skin Tone: A Multidimensional Measure of Apparent Skin Color – Sony AI
- Research: Feature and Label Embedding Spaces Matter in Addressing Image Classifier Bias – Sony AI
Latest Blog
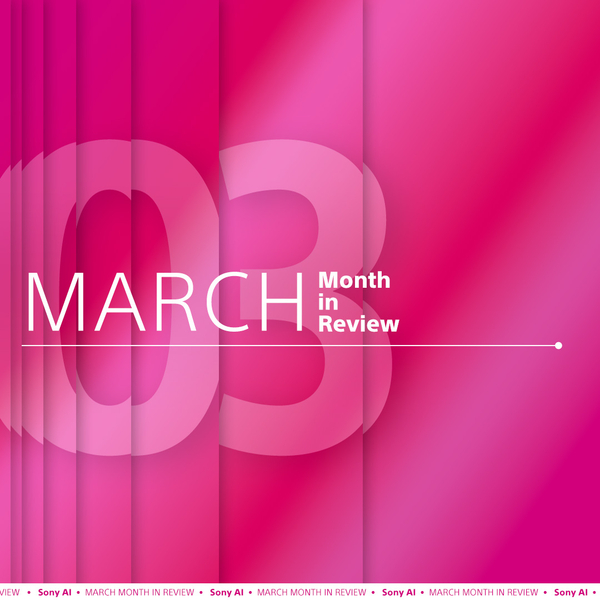
April 1, 2025 | Sony AI
Advancing AI: Highlights from March
March was a month of notable advancements, global discussions, and strategic collaborations, reinforcing our commitment to responsible AI innovation. From pioneering multi-modal AI…
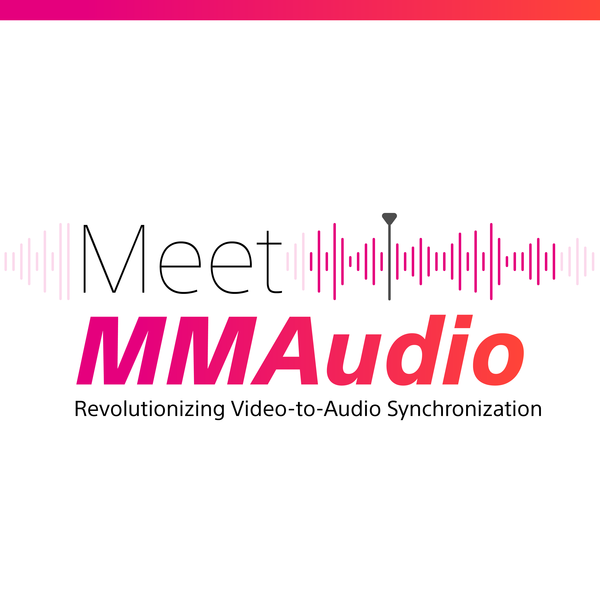
March 18, 2025 | Sony AI
Unlocking the Future of Video-to-Audio Synthesis: Inside the MMAudio Model
In a world increasingly driven by multimedia content, AI researchers have long struggled to generate high-quality, synchronized audio from video. Recently, audio generation models …
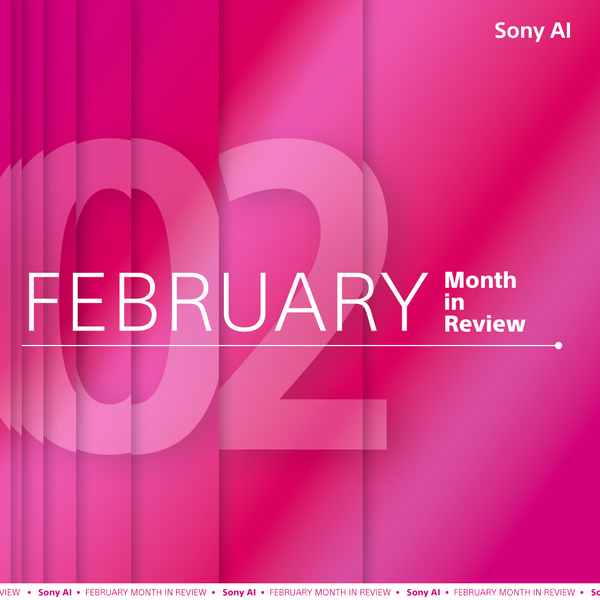
March 1, 2025 | Sony AI
Advancing AI: Highlights from February
Sony AI In Focus: February 2025At Sony AI, every month brings new advancements that push the boundaries of artificial intelligence. February has been a month of innovation, from gr…