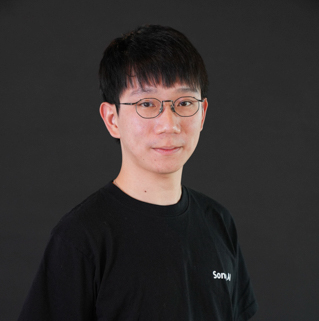
Chieh-Hsin
Lai
Profile
Since 2021, Jesse has worked as a research scientist at Sony AI, focusing on robustness, deep generative models, and theoretical deep learning. Prior to Sony AI, he worked as a Research Assistant at the Institute of Mathematics Academia Sinica. Jesse earned his PhD in Mathematics from the University of Minnesota - Twin Cities.
Message
“I specialize in developing theoretical grounded deep generative models that excel in producing high-fidelity samples, rapid sampling, ease of training, and controllable generation. I expect to unlock the black-box nature of deep generative modeling through the application of advanced mathematical tools. With a focus on innovation and precision, I am dedicated to pushing the boundaries of artificial intelligence and contributing to the advancement of cutting-edge technology.”
Publications
Generative adversarial networks (GANs) learn a target probability distribution by optimizing a generator and a discriminator with minimax objectives. This paper addresses the question of whether such optimization actually provides the generator with gradients that make its d…
Despite the recent advancements, conditional image generation still faces challenges of cost, generalizability, and the need for task-specific training. In this paper, we propose Manifold Preserving Guided Diffusion (MPGD), a training-free conditional generation framework th…
Consistency Models (CM) (Song et al., 2023) accelerate score-based diffusion model sampling at the cost of sample quality but lack a natural way to trade-off quality for speed. To address this limitation, we propose Consistency Trajectory Model (CTM), a generalization encomp…
Restoring degraded music signals is essential to enhance audio quality for downstream music manipulation. Recent diffusion-based music restoration methods have demonstrated impressive performance, and among them, diffusion posterior sampling (DPS) stands out given its intrin…
Pre-trained diffusion models have been successfully used as priors in a variety of linear inverse problems, where the goal is to reconstruct a signal from noisy linear measurements. However, existing approaches require knowledge of the linear operator. In this paper, we prop…
Score-based generative models learn a family of noise-conditional score functions corresponding to the data density perturbed with increasingly large amounts of noise. These perturbed data densities are tied together by the Fokker-Planck equation (FPE), a partial differentia…
Removing reverb from reverberant music is a necessary technique to clean up audio for downstream music manipulations. Reverberation of music contains two categories, natural reverb, and artificial reverb. Artificial reverb has a wider diversity than natural reverb due to its…
One noted issue of vector-quantized variational autoencoder (VQ-VAE) is that the learned discrete representation uses only a fraction of the full capacity of the codebook, also known as codebook collapse. We hypothesize that the training scheme of VQ-VAE, which involves some…
JOIN US
Shape the Future of AI with Sony AI
We want to hear from those of you who have a strong desire
to shape the future of AI.