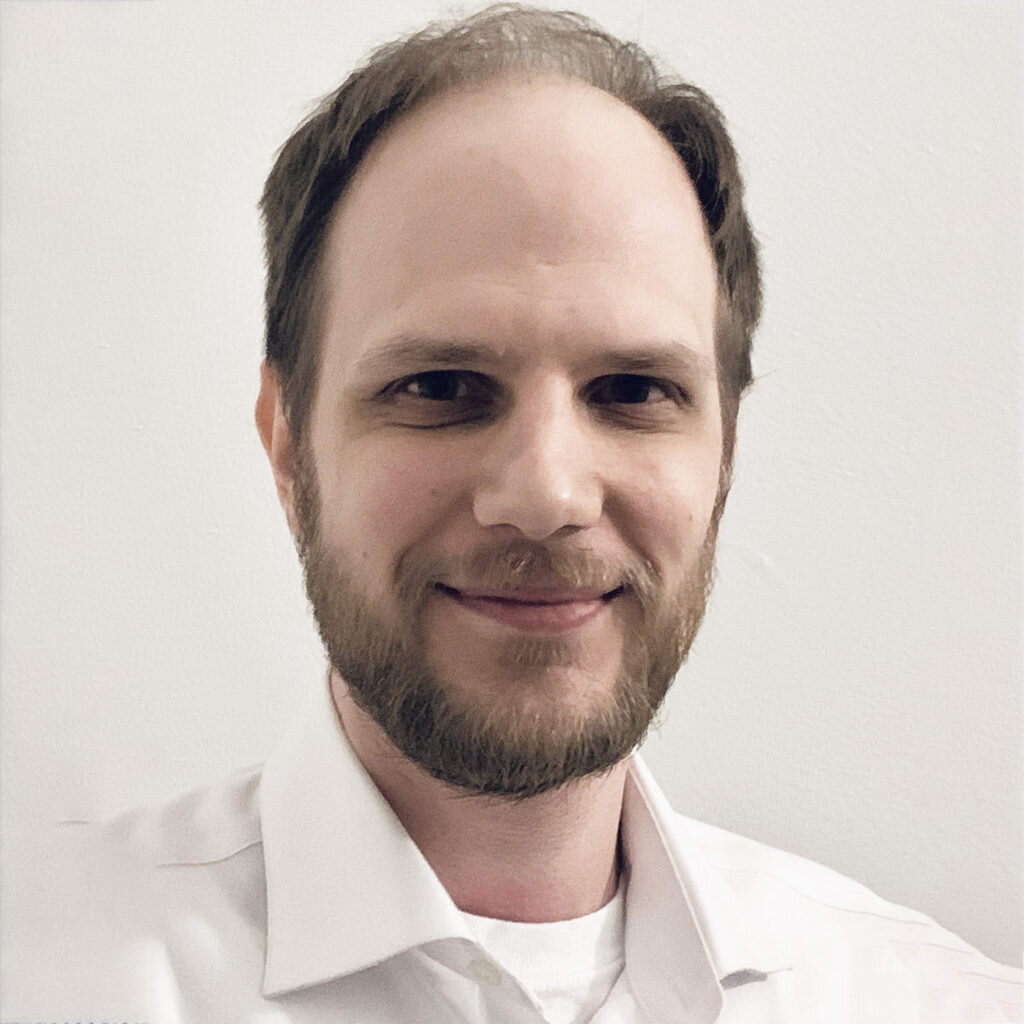
James
MacGlashan
Profile
James is a senior research scientist at Sony AI. He received his PhD in computer science from the University of Maryland, Baltimore County. He went on to do postdoctoral research at Brown University from 2013-2016. He researches autonomous decision-making agents, focusing on Reinforcement Learning (RL), RL that involves interaction with people, and RL for robotics. He was the creator of the Brown-UMBC Reinforcement Learning and Planning (BURLAP) Java library, which was one of the largest early efforts to make an open-source RL library.
Message
“My core goal at Sony AI is to apply reinforcement learning (RL) to real applications like games and robotics, and to research methods that make RL a more reliable tool that can be adopted by non-experts. As a consequence, I am interested in fundamental algorithmic innovations to improve the reliability of RL, methods that provide better insights into the outcomes of RL, and ways people can shape learning. Whenever possible, I like to ground my investigations into real-world applications to focus my research on the core issues with which RL methods currently struggle.”
Publications
Designing reinforcement learning (RL) agents is typically a difficult process that requires numerous design iterations. Learning can fail for a multitude of reasons and standard RL methods provide too few tools to provide insight into the exact cause. In this paper, we show …
Many potential applications of artificial intelligence involve making real-time decisions in physical systems while interacting with humans. Automobile racing represents an extreme example of these conditions; drivers must execute complex tactical manoeuvres to pass or block…
It has been recently demonstrated that Temporal Convolution Networks (TCNs) provide state-of-the-art results in many problem domains where the input data is a time-series. TCNs typically incorporate information from a long history of inputs (the receptive field) into a singl…
Blog
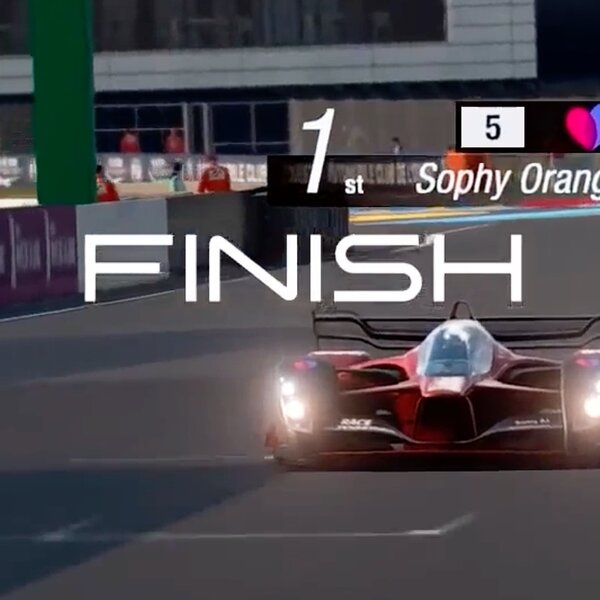
October 4, 2022 | GT Sophy | Game AI
The Race to Turn a World-class AI into a World Champion
GT SOPHY TECHNICAL SERIES Starting in 2020, the research and engineering team at Sony AI set out to do something that had never been done before: create an AI agent that could beat the best drivers in the world at the PlayStation®…
GT SOPHY TECHNICAL SERIES Starting in 2020, the research and engineering team at Sony AI set out to do something that had never be…
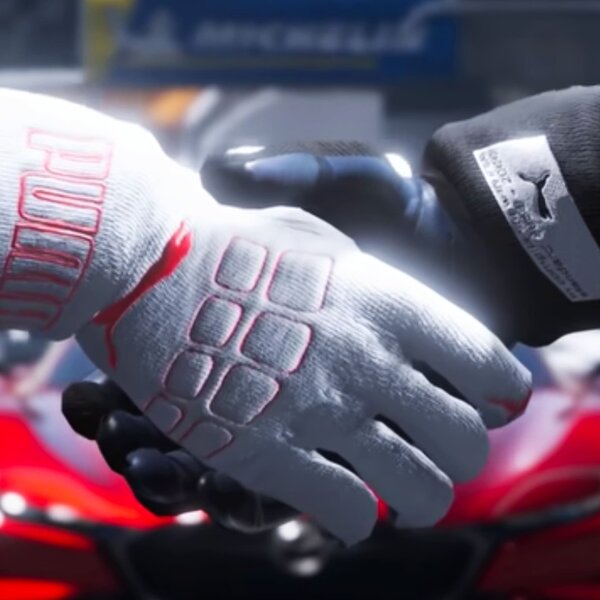
September 15, 2022 | GT Sophy | Game AI
Don’t Cross That Line! How Our AI Agent Learned Sportsmanship
GT SOPHY TECHNICAL SERIES Starting in 2020, the research and engineering team at Sony AI set out to do something that had never been done before: create an AI agent that could beat the best drivers in the world at the PlayStation®…
GT SOPHY TECHNICAL SERIES Starting in 2020, the research and engineering team at Sony AI set out to do something that had never be…
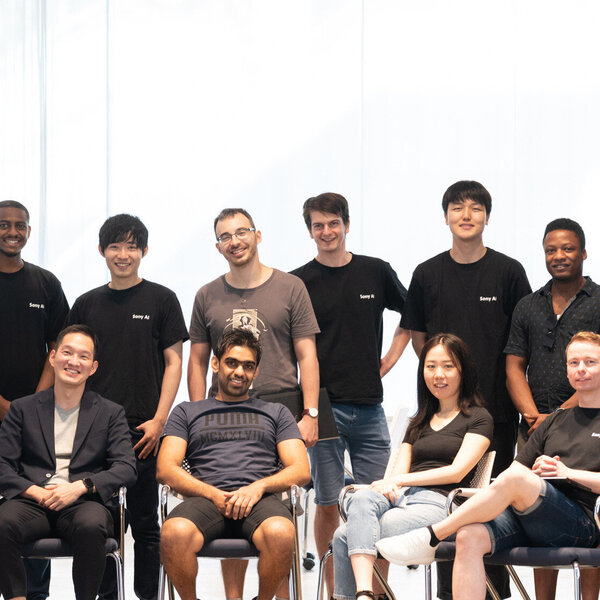
October 12, 2021 | Life at Sony AI
Meet the Team #1: James, Guillem and Raphaela
This is the first post in our new Sony AI Meet the Team series, which will shine a spotlight on the individuals that make up our very talented Sony AI team. Once a month, we will share highlights from our interviews with Sony AI …
This is the first post in our new Sony AI Meet the Team series, which will shine a spotlight on the individuals that make up our …
JOIN US
Shape the Future of AI with Sony AI
We want to hear from those of you who have a strong desire
to shape the future of AI.