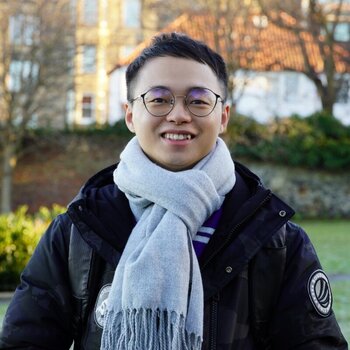
Weiming
Zhuang
Profile
Weiming is a research scientist in Privacy-Preserving Machine Learning (PPML) at Sony AI. His research interests and expertise span federated learning, AI privacy and security, computer vision, and machine learning systems. Before joining Sony AI, Weiming was a Ph.D. researcher under SenseTime-NTU Talent Programme and received his Ph.D. from Nanyang Technological University. He spent two years in software engineering building large-scale distributed systems and completed his Bachelor's from the National University of Singapore, School of Computing. Weiming has published papers in top-tier conferences and journals, including ICLR, ICCV, etc., and his papers have been selected as oral presentations at top conferences.
Message
My role at Sony AI is to empower Sony with privacy-preserving and robust AI solutions and to contribute to the global discourse with cutting-edge AI research.
Publications
While existing vision and multi-modal foundation models can handle multiple computer vision tasks, they often suffer from significant limitations, including huge demand for data and computational resources during training and inconsistent performance across vision tasks at d…
In computer vision, it is well-known that a lack of data diversity will impair model performance. In this study, we address the challenges of enhancing the dataset diversity problem in order to benefit various downstream tasks such as object detection and instance segmentati…
We present COALA, a vision-centric Federated Learning (FL) platform, and a suite of benchmarks for practical FL scenarios, which we categorize as task, data, and model levels. At the task level, COALA extends support from simple classification to 15 computer vision tasks, in…
Federated learning (FL) promotes decentralized training while prioritizing data confidentiality. However, its application on resource-constrained devices is challenging due to the high demand for computation and memory resources for training deep learning models. Neural netw…
Federated learning has become a popular method to learn from decentralized heterogeneous data. Federated semi-supervised learning (FSSL) emerges to train models from a small fraction of labeled data due to label scarcity on decentralized clients. Existing FSSL methods assume…
Federated learning (FL) enhances data privacy with collaborative in-situ training on decentralized clients. Nevertheless, FL encounters challenges due to non-independent and identically distributed (non-i.i.d) data, leading to potential performance degradation and hindered c…
Federated learning (FL) is an effective machine learning paradigm where multiple clients can train models based on heterogeneous data in a decentralized manner without accessing their private data. However, existing FL systems undergo performance deterioration due to feature…
Federated learning (FL) is an emerging distributed machine learning method that empowers in-situ model training on decentralized edge devices. However, multiple simultaneous FL tasks could overload resource-constrained devices. In this work, we propose the first FL system to…
This paper focuses on an under-explored yet important problem: Federated Class-Continual Learning (FCCL), where new classes are dynamically added in federated learning. Existing FCCL works suffer from various limitations, such as requiring additional datasets or storing the …
Blog
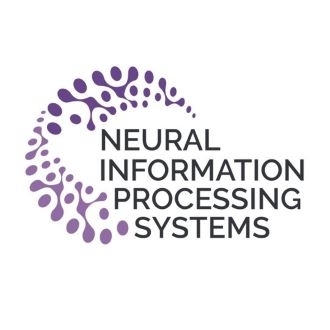
December 13, 2023 | Events
Sony AI Reveals New Research Contributions at NeurIPS 2023
Sony Group Corporation and Sony AI have been active participants in the annual NeurIPS Conference for years, contributing pivotal research that has helped to propel the fields of artificial intelligence and machine learning forwar…
Sony Group Corporation and Sony AI have been active participants in the annual NeurIPS Conference for years, contributing pivotal …
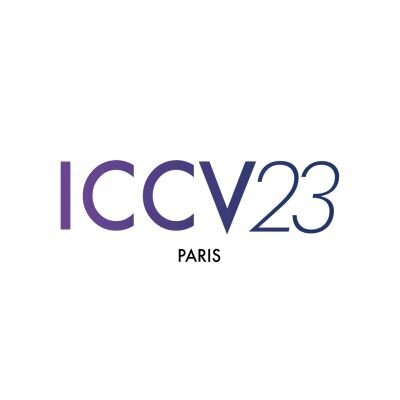
October 6, 2023 | PPML
Advancements in Federating Learning Highlighted in Papers Presented at ICCV 2023
As the field of machine learning continues to evolve, Sony AI researchers are constantly exploring innovative solutions to address the pressing issues faced by the industry. Two research papers, both accepted at the premier intern…
As the field of machine learning continues to evolve, Sony AI researchers are constantly exploring innovative solutions to address…
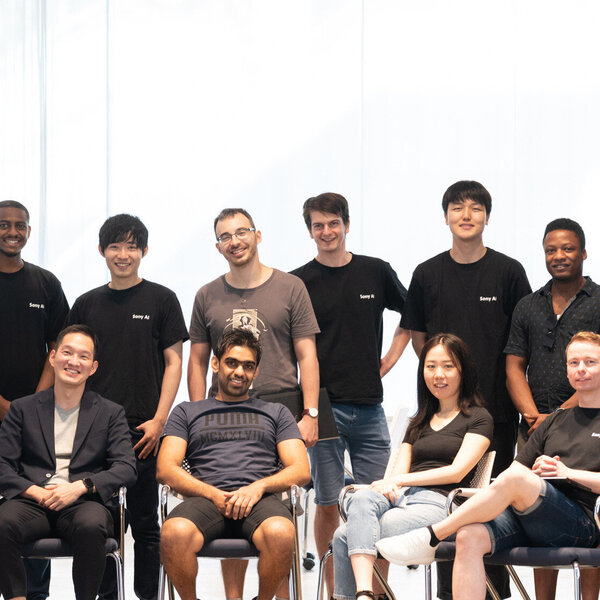
July 13, 2023 | Life at Sony AI
Meet the Team #8: Weiming Zhuang, Nidham Gazagnadou, Chen Chen
At Sony AI, the Privacy-Preserving Machine Learning (PPML) team focuses on fundamental and applied research in computer vision privacy. Their innovative research aims to apply these novel ideas to real-world AI applications. In th…
At Sony AI, the Privacy-Preserving Machine Learning (PPML) team focuses on fundamental and applied research in computer vision pri…
JOIN US
Shape the Future of AI with Sony AI
We want to hear from those of you who have a strong desire
to shape the future of AI.