How to Train Your Race Car
Teaching GT Sophy skills and tactics
Gaming
GT Sophy
July 12, 2022
GT SOPHY TECHNICAL SERIES
Starting in 2020, the research and engineering team at Sony AI set out to do something that had never been done before: create an AI agent that could beat the best drivers in the world at the PlayStation® 4 game Gran Turismo™ Sport, the real driving simulator developed by Polyphony Digital. In 2021, we succeeded with Gran Turismo Sophy™ (GT Sophy). This series explores the technical accomplishments that made GT Sophy possible, pointing the way to future AI technologies capable of making continuous real-time decisions and embodying subjective concepts like sportsmanship.
At the 1960 Daytona 500, Junior Johnson entered the race as a long shot. His ’59 Chevy was under-powered, with lap times well behind the top contenders. But during practice, Johnson discovered the “slipstream” effect: by drafting in the air wake of the faster cars, not only could he keep up with them, he could actually use the added momentum to slingshot past them. Johnson rode the air (and a bit of luck) to victory and showed that it wasn’t just speed that wins races—but also the driver’s skills.
As we trained GT Sophy to race against the world’s best esports drivers, the question of skills loomed large. Could GT Sophy learn complex tactics like maneuvering through crowded starts; making inside, outside, and “crossover” passes in the turns; or harnessing the slipstream to make slingshot passes like Junior Johnson? And how would we know if GT Sophy had mastered those skills? To answer these questions, we dedicated part of our research team to building a training regimen to teach GT Sophy the skills and tactics of motorsports.
GT Sophy’s Training Regimen The goal of the GT Sophy project was to train an agent to outrace the best esports drivers in the world on three different tracks in the PlayStation game Gran Turismo Sport. The agent was trained through reinforcement learning, starting from a blank slate and gathering experience by racing against other AI agents. GT Sophy used these interactions to learn a policy, mapping its observations of the world (speed, position, etc.) to the best action (throttle, brake, and steering) for its current state. The ultimate races against top humans would contain many different situations, from crowded starts to one-on-one battles, so we trained GT Sophy using randomly sampled training scenarios covering a wide variety of traffic conditions.
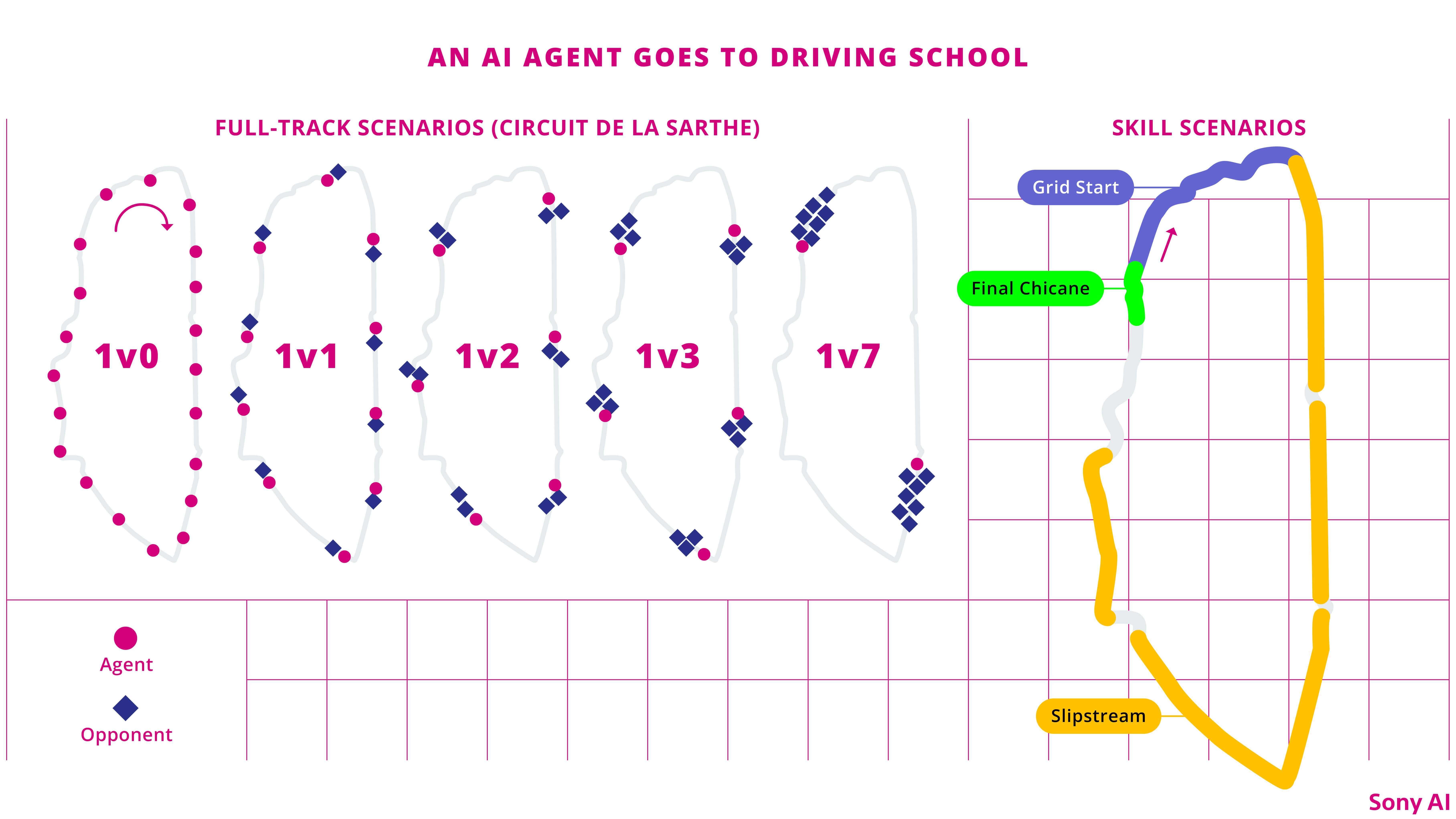
We ensured that GT Sophy gained exposure to a wide range of racing situations—from competing head-to-head with one other car to navigating a crowded track.
The general training scenarios at each track were made up of “1vN” scenarios that trained GT Sophy to drive in traffic with 0,1,2,3, or 7 opponents nearby. Since Gran Turismo Sport, the 2017 iteration of the game we were training the agents on, supports up to 20 cars on the track, we ran parallel versions of a given scenario equally spaced around the course, each with their own randomizations for opponents, starting positions, and speed.
While the 1vN scenarios taught GT Sophy how to drive in packs, they were ineffective for teaching nuanced techniques like slipstream passing or starts. The problem in both cases was one of exposure. Relying on random draws of opponent distances and speeds, it was unlikely for GT Sophy to experience certain scenarios, such as a perfectly spaced grid start. To compensate, we added scenarios covering the following skills at each track.
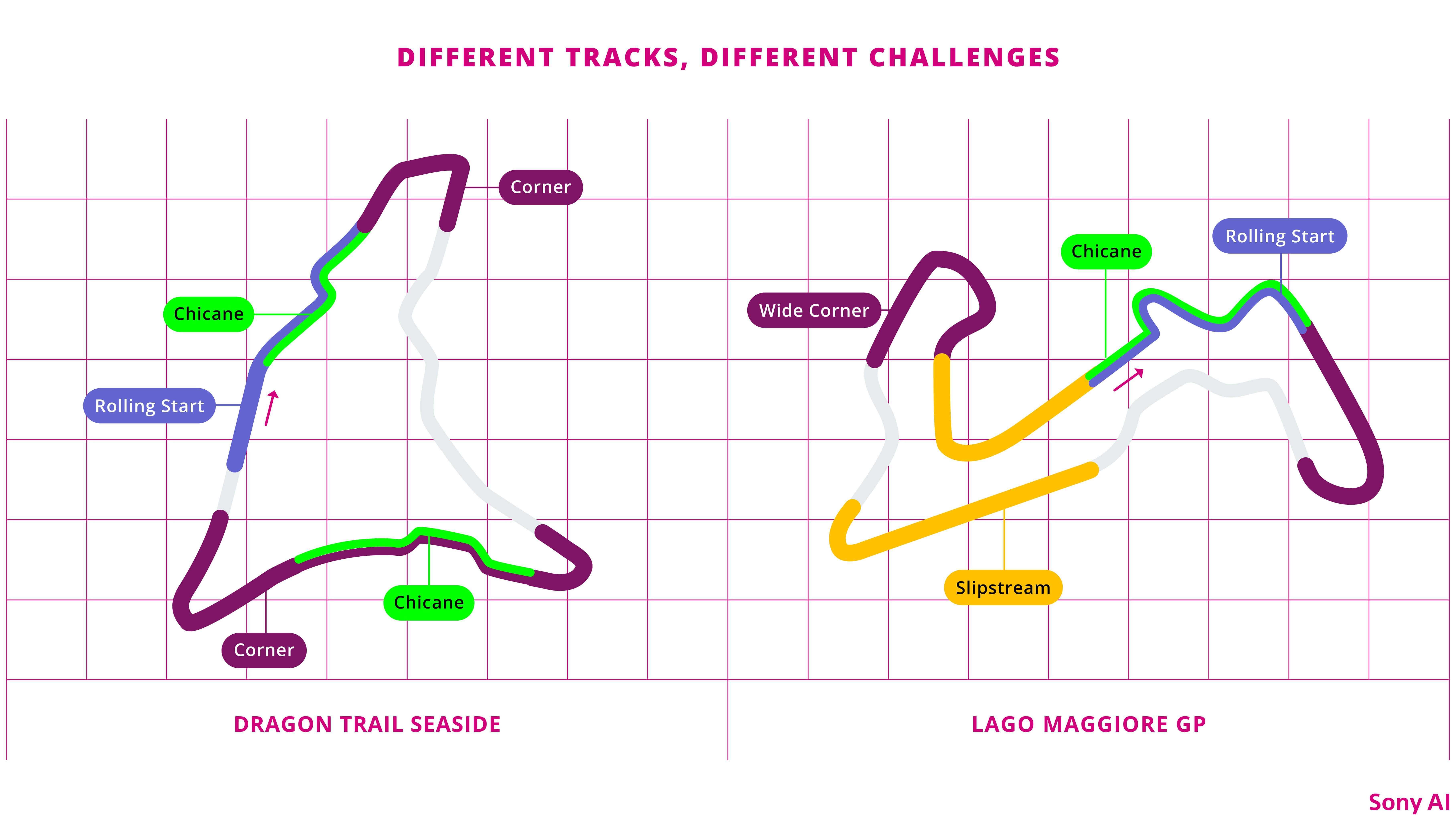
GT Sophy mastered driving skills on several courses within the Gran Turismo universe.
Starts: The start of the race is often intense and chaotic, making it easy to lose a position or cause an accident. To prepare for a wide variety of rapidly evolving and dynamic situations, GT Sophy trained in each possible position within the track-specific start configurations. The scenario ran for a predetermined length to keep the focus on the start itself. We randomized the opponents, and even some of the grid spots, to make the policy more robust. You can see the resulting skillful start in the video against top drivers below:
After training, GT Sophy took the lead at the start of the Dragon Trail Seaside track in a race against top drivers.
Overtaking and defending in curves: Each track has specific turns that require a lot of practice. These turns might be tight and in rapid succession (a chicane), or present opportunities for inside, outside, or crossover passes where an agent switches lines. At each track we built scenarios to launch GT Sophy among a crowd of randomized opponents entering these specific sections so it could learn the arts of passing and defending.
This skill training paid off. In the following clips against top Gran Turismo drivers, GT Sophy makes the most of very different scenarios. When running solo, the agent takes a traditional out-in-out line through the turn. When that line is blocked by an opponent, GT Sophy pressures them with an outside pass attempt. And in the third clip, GT Sophy “fakes out” its opponent by feigning an outside line and then crossing over for an inside pass when the opponent leaves an opening.
GT Sophy learned to navigate different scenarios, whether driving solo, getting blocked by another car, or seizing an opportunity to fake out an opponent and pull ahead.
Another demonstration of situational skills in a complex turn took place on Gran Turismo Sport’s Lago Maggiore GP track. Here, two GT Sophy agents are racing against two world-class humans, with all four hurtling down the hill into the banked Turn 11. As shown on the diagram below, GT Sophy’s preferred line when driving solo is a classic out-in-out cut through the turn. But because of the traffic, each GT Sophy instance picks a different line, initially giving up positions to the humans, but then rocketing ahead of them through the turn and defending the positions on exit.
Competing against two human drivers on the Lago Maggiore GP track, two GT Sophy agents each carved out different paths—and then burst ahead of the humans.
Slipstream passing: On the Circuit de la Sarthe track, where GT Sophy raced in Formula 1-like cars on long straightaways and tight chicanes, slipstream drafting and slingshot passing were the most crucial skills. To make sure GT Sophy could master those skills, we added training scenarios with a variety of opponents, ensuring GT Sophy learned how to make both left and right slingshot passes. Adding in similar exercises against more defensive opponents gave GT Sophy the skills to make expert slingshot passes against the best players in the world, no matter which side of the track they were on.
GT Sophy learned to draft in the air wake of its opponents—picking up momentum that allowed it to slingshot past them.
Putting All the Skills Together You never know what opportunities will present themselves during a race, so the agent had to be ready to take advantage of any mistake that human competitors would make, anywhere on the track. However, teaching a policy to master all of the skills required more machinery.
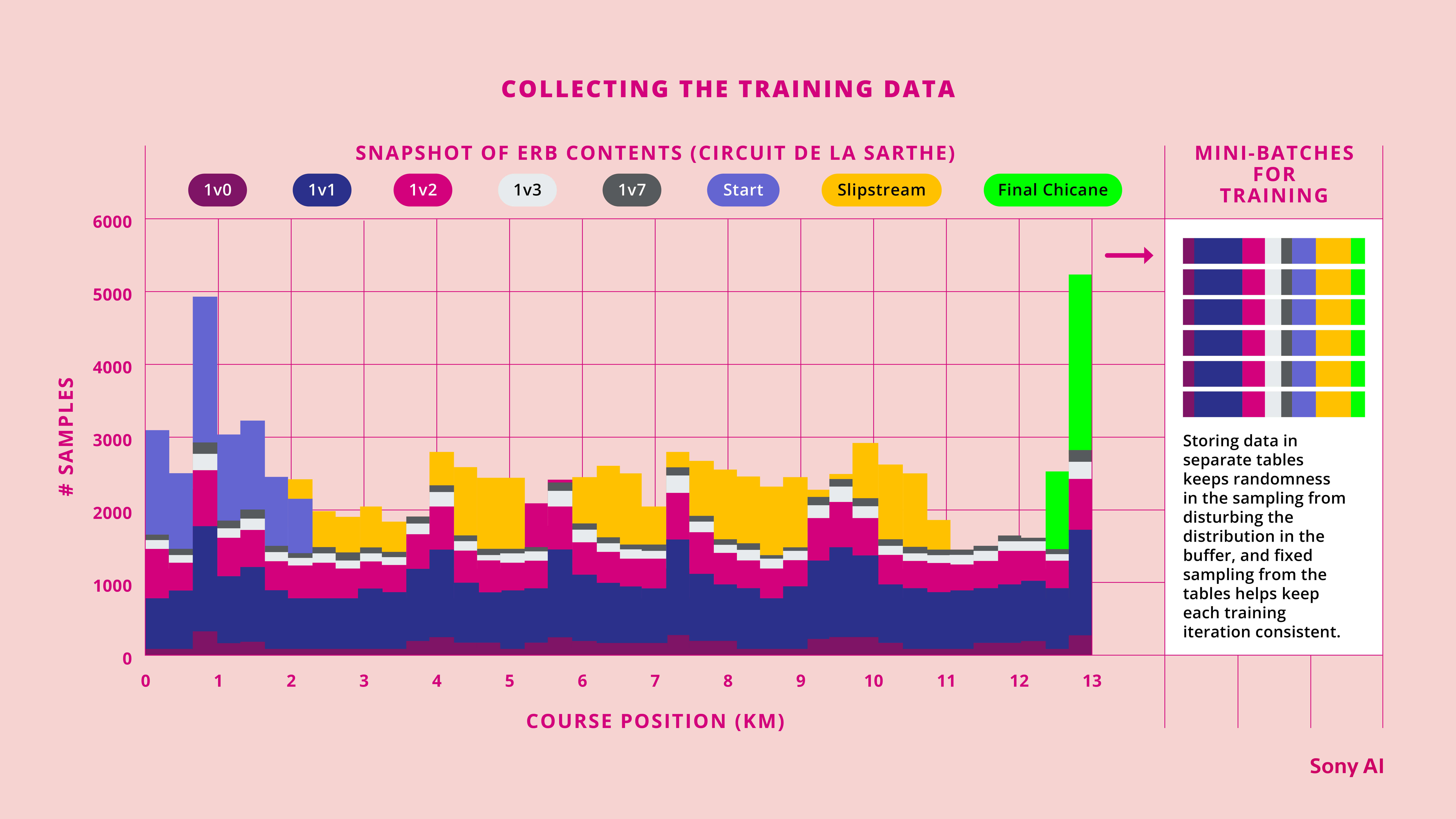
We stored data in an experience replay buffer and used it to build GT Sophy’s skills.
In particular, training data was collected by randomly sampling the different scenarios above. We then stored the data in an experience replay buffer and randomly sampled it to build the mini-batches of data used to train the policy. All of this randomization led to fluctuations where certain skills might temporarily be lost due to data imbalances. To calm these waves, we applied a form of stratified sampling we call multi-table experience replay that partitioned the replay buffer into different tables, one for each skill or scenario set. When constructing a mini-batch, fixed weights keep the proportion of data for each skill consistent throughout learning. The result was far more consistent performance of the agent throughout the learning process.
GT Sophy learned racing skills through its own experience, making it a contender against the best esports racers in the world.
This process stabilized learning but gave us two sets of hyperparameters to optimize: the weights for sampling tasks and those for sampling from the replay buffer. For task sampling, we normalized the weights based on the number of agents and the length of each task. And for both weight sets, we used parameter sweeps to determine optimal weightings to learn all the skills.
Testing GT Sophy’s Skills With so many training runs and policies to choose from, how could we pick the best policy to race? Our selection process started with policies captured from a set of runs and each evaluated once to test for rough speed and competitiveness. The best of these policies were then put through an N-athlon, our generalization of a decathlon, with “events” designed to test for each skill the agent was supposed to have mastered. As an example, the “slipstream passing” event tested the agent’s capability to make slingshot passes against different opponents all over the track and differentiated the best agents from those that were “close but not good enough.”
We tested agents to find those with the strongest skills—in this case, we sought out those that excelled at slingshot passes.
We scored the N-athlon events based on various criteria and ranked each policy to emphasize the need for mastery of all the skills, not just being good at one particular aspect of racing. From these rankings, Sony AI researchers selected a set of top-performing policies based on the rankings and individual skill tests, trying to find a small set of policies that had the best overall skills and a diversity of training parameters that might differentiate them in further testing. Those policies were then pitted against each other in four-on-four “policy versus policy” races using the same format and scoring system as the upcoming races against human champions. As a final check, the top policies raced against human testers to stress test both the skills and sportsmanship of the trained agents. These final tests with human drivers sometimes highlighted issues that we didn’t catch earlier, such as agents that would timidly move aside if a human car lunged at them from a safe distance, a behavior they never encountered while racing other AIs.
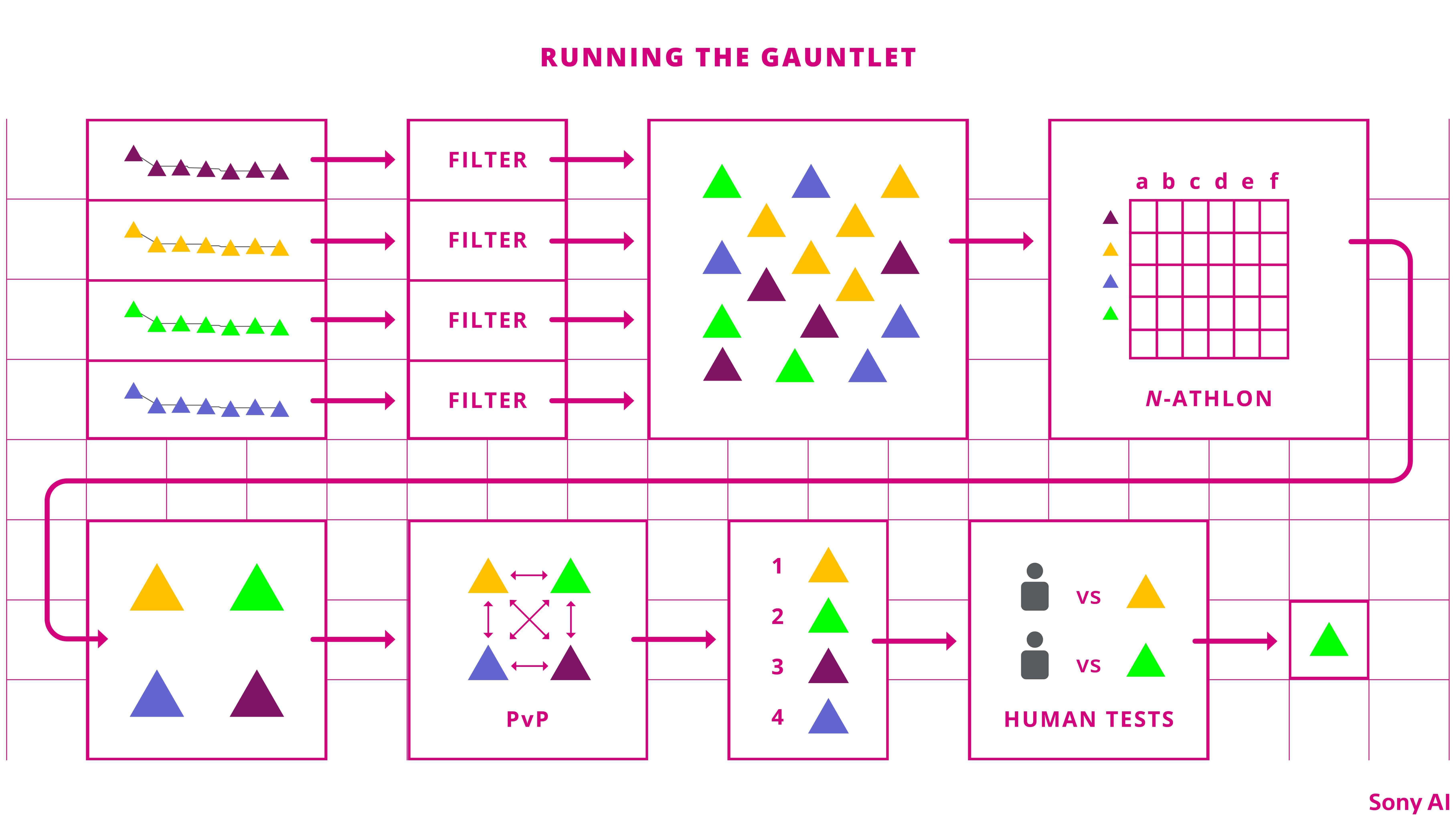
We tested GT Sophy’s performance through a complex challenge modeled after a decathlon, with various “events” for each skill it had to learn.
Learning to Do It All Teaching GT Sophy racing tactics was a new challenge for our team. Unlike traditional AI where skills are chained or composed, GT Sophy needed to “do it all”—to embody many different skills at once. We took on this challenge using diversified training scenarios, multi-table experience replay, and the N-athlon to train and identify the most skillful and well-balanced policies. Looking back at early iterations of GT Sophy before it was trained with these approaches, it certainly would have been a long shot for it to win against top human drivers. But like Junior Johnson at Daytona, GT Sophy learned racing skills through its own experience, making it a contender against the best esports racers in the world.
Latest Blog
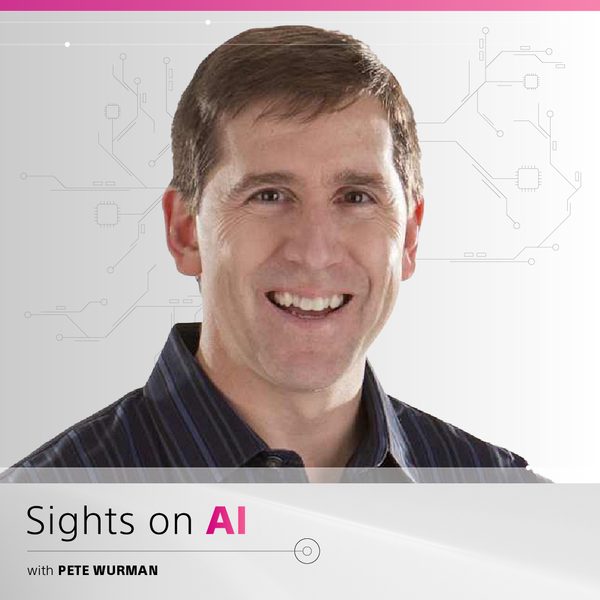
February 5, 2025 | Sony AI
Sights on AI: Pete Wurman Discusses Career in AI and How the Technology is Evolv…
The Sony AI team is a diverse group of individuals working to accomplish one common goal: accelerate the fundamental research and development of AI and enhance human imagination an…
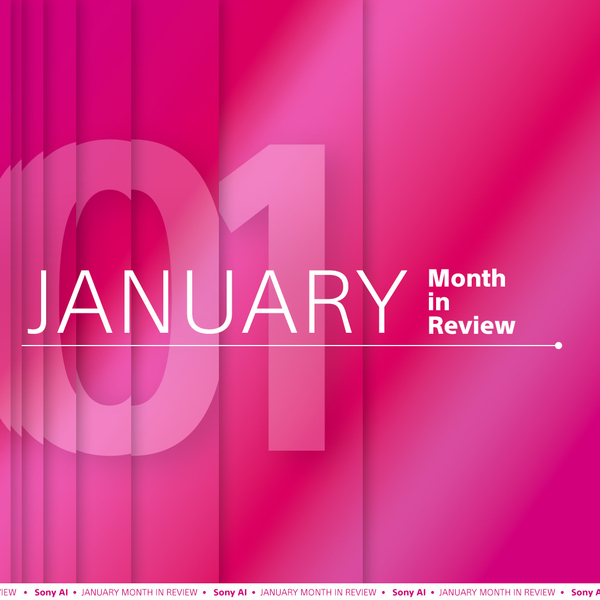
January 31, 2025 | Sony AI
Advancing AI: Highlights from January
At Sony AI, every month is an opportunity to innovate, connect, and share our progress with you. Welcome to Advancing AI, our brand-new monthly round-up series where we highlight t…
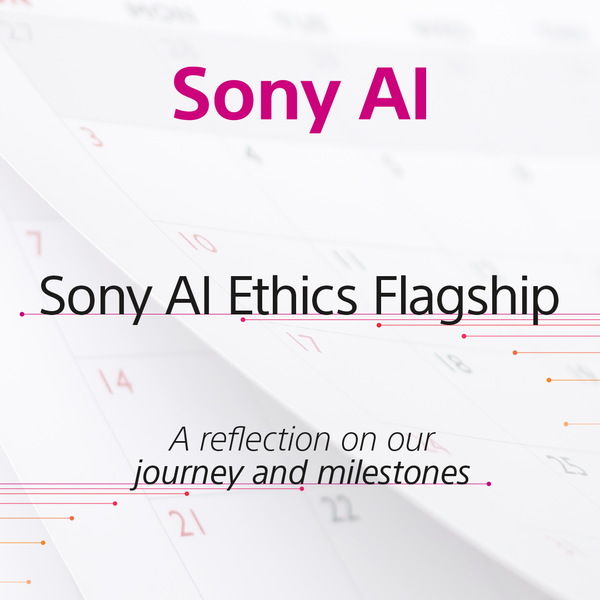
January 14, 2025 | AI Ethics
Sony AI Ethics Flagship: Reflecting on Our Progress and Purpose
Since Sony AI’s inception, ethics has been at the heart of everything we do. The establishment of our AI Ethics Research Flagship at the beginning of our journey laid the foundatio…