Sights on AI: Peter Stone Talks Reinforcement Learning
Life at Sony AI
May 30, 2023
The Sony AI team is a diverse group of individuals working to accomplish one common goal: accelerate the fundamental research and development of AI and enhance human imagination and creativity, particularly in the realm of entertainment. Each individual brings different experiences, along with a unique view of the technology, to this work. This insightful Q&A series, Sights on AI, will highlight the career journeys of Sony AI’s leaders and offer their perspectives on a number of AI topics.
Peter Stone, PhD, is the Executive Director of Sony AI America and has been a leader in AI for more than two decades. In addition to his role at Sony AI, he is also the founder and director of the Learning Agents Research Group (LARG) in the Department of Computer Science at The University of Texas (UT) at Austin, where he is also a Professor of Computer Science, associate chair of the department, and Director of Texas Robotics. Peter has received numerous accolades throughout his career, including the prestigious IJCAI Computers and Thought Award, given biannually to the top AI researcher under the age of 35.
While Peter can discuss many facets of AI, he is one of the foremost experts in the AI training method of reinforcement learning. In this installment of our Sights on AI Q&A series, we spoke with Peter about how he came to focus on reinforcement learning and its evolution.
How did you first get involved in reinforcement learning?
My first year as a Ph.D. student, I was working on what is known as AI planning, which is about thinking through the steps you would go through to make something happen and change the world from one state to another. This didn’t involve any real robot or agent, and there was no learning or execution happening. It was all about “thinking” through the process.
A pivotal moment in my AI career happened the summer after that first year when I attended AAAI 1994 in Seattle. During that event, I was exposed to the concept of robot soccer and the notion of creating autonomous agents that can make decisions in the world. Reinforcement learning is designed for that — it is about how you use machine learning to improve an agent’s decision-making.
I quickly started reading the literature on it, but at the time, reinforcement learning was really only a side area of AI. While it was not very popular, it appealed to me because of the idea of learning from experience as well as trial and error, and getting an agent that is actually acting and moving in the world. Years later, the culmination of my Ph.D. thesis was (partly) about applying reinforcement learning in the robot soccer space.
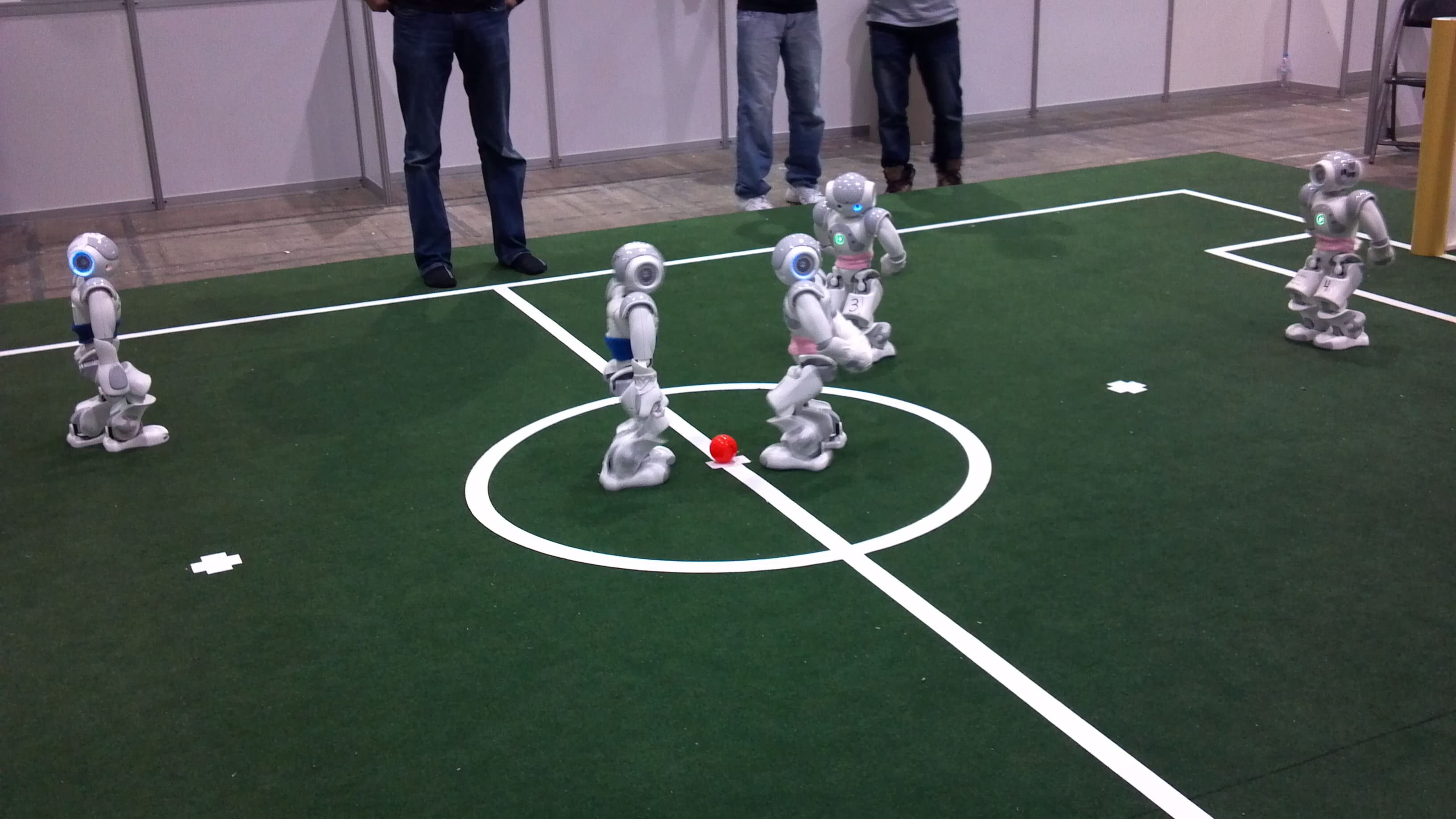
How has reinforcement learning evolved over the years?
The birth of modern reinforcement learning was in the 1980s before I became involved in it. There was an important Ph.D. thesis from Chris Watkins, who proved a fundamental result in this area. This piece of research caused a small bump in popularity among the machine learning community, but at the main machine learning conferences there were still few papers on reinforcement learning algorithms and a handful of people that were working in the space.
Reinforcement grew gradually from the 1980s and gained some notoriety as people like me started to use it for robot learning. I was particularly interested in applying reinforcement learning to multi-agent learning for activities like robot soccer. I also applied it to teaching robots how to walk, which I believe helped cause an uptick in interest for reinforcement learning.
However, one of the biggest landmarks in reinforcement learning came when it started being deployed to games such as Atari and AlphaGo — which defeated the world go champion. More recently, the unveiling of the research for our superhuman autonomous AI agent, Gran Turismo SophyTM (“GT Sophy”), was seen as as major breakthrough on account of its application of deep reinforcement learning for driving control, racing tactics, and racing etiquette within the highly realistic PlayStation®4 racing simulation game, Gran TurismoTM (GT) Sport.
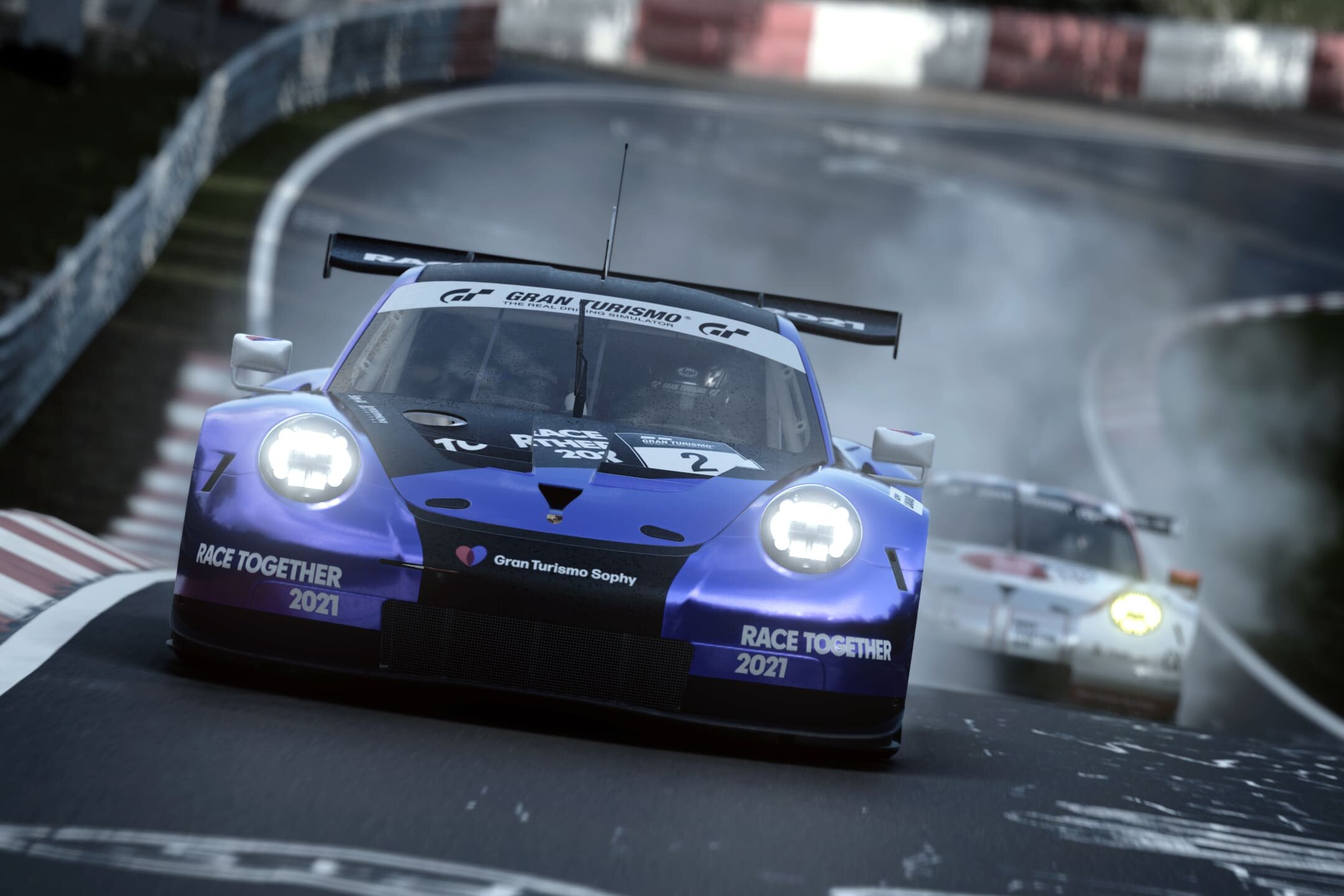
Gran Turismo 7: © 2023 Sony Interactive Entertainment Inc. Developed by Polyphony Digital Inc.
There has also been a more recent surge in interest around reinforcement learning because of generative AI. Many of today’s most recent AI projects have leveraged reinforcement learning from human feedback. While this type of reinforcement learning may seem like a new concept, it actually isn’t. One of my former students at UT Austin, Brad Knox, was arguably one of the first to pioneer in the area. He first published a paper on it back in 2008, and there have been other papers published since that time detailing the use of human feedback in reinforcement learning.
What does the future of reinforcement learning look like from your perspective?
I expect to see a big boom in reinforcement learning in the coming years. I believe this will be largely due to its growing deployment in video games.
Video games provide the ideal application for reinforcement learning. Our work at Sony AI with GT Sophy was a perfect example of this. It took only one year to go from our research featured on the cover of Nature in February 2022 to introducing GT Sophy as a commercialized product within Gran Turismo 7.
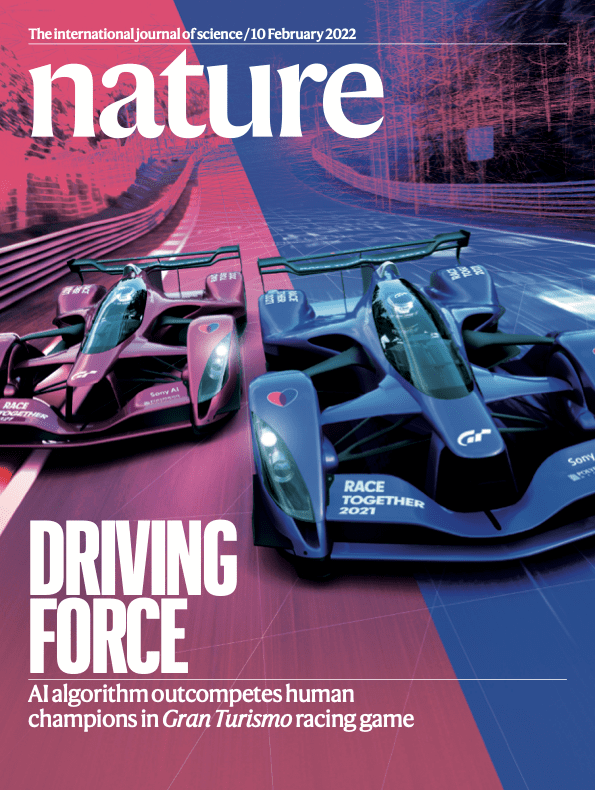
For industry projects that leverage reinforcement learning, much like GT Sophy, I anticipate we will continue to see timelines shorten between research and commercialization. More organizations are beginning to see the true power of reinforcement learning, which is driving the need to deploy it in a real-world context by harnessing the power of their internal R&D groups.
More broadly, I expect reinforcement learning to also play a smaller role in other types of systems like autonomous driving, robotics, and content delivery. Even if not for end-to-end systems, it may be used for subsystems such as route planning, navigation, and content moderation. I also anticipate that we will see additional new algorithms to make certain well-defined tasks more efficient and effective in various industries.
I think we will also see a surge in research and Ph.D. theses on reinforcement learning, which may focus on how to improve the methods and causal modeling, among other things. This research conducted at the academic level will center around the problems and topics that have a relatively longer time horizon between innovation and being ready to incorporate in a product. Projects can include the fundamental theoretical analyses and novel algorithms for efficient exploration, off-policy learning, and bridging the gap between simulation and the real world.
Latest Blog
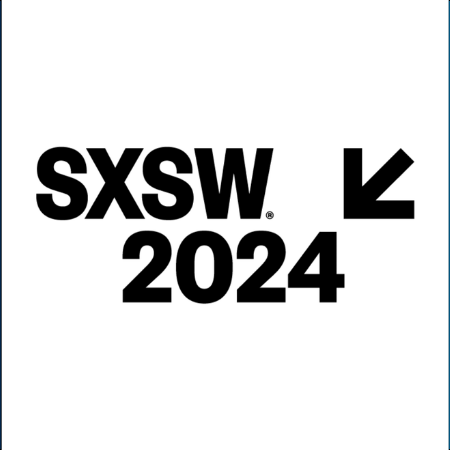
April 3, 2024 | Events
That’s A Wrap: Sony AI Ends Fiscal Year 2023 with SXSW, Two New Published Articl…
The past year marked a period of remarkable creativity, innovation, and excitement for Sony AI as it advanced its mission to research and develop AI that augments, and works in har…
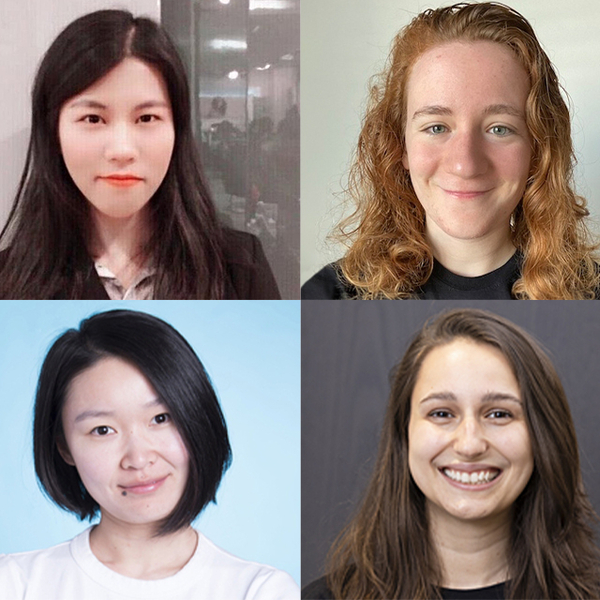
March 29, 2024 | Life at Sony AI
Celebrating the Women of Sony AI: Sharing Insights, Inspiration, and Advice
In March, the world commemorates the accomplishments of women throughout history and celebrates those of today. The United States observes March as Women’s History Month, while man…
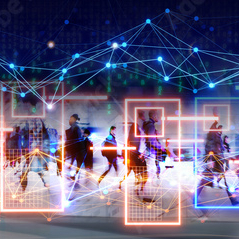
March 26, 2024 | AI Ethics
When Privacy and Fairness Collide: Reconciling the Tensions Between Privacy and …
Invisibility is sometimes thought of as a superpower. People often equate online privacy with selective invisibility, which sounds desirable because it potentially puts them in con…