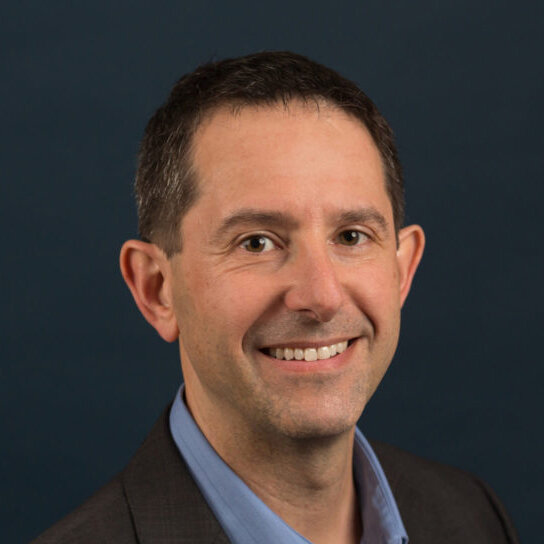
Peter
Stone
Profile
Peter is the Chief Scientist of Sony AI. He is also the founder and director of the Learning Agents Research Group (LARG) within the Artificial Intelligence Laboratory in the Department of Computer Science at The University of Texas at Austin, as well as associate department chair and Director of Texas Robotics. In 2013 he was awarded the University of Texas System Regents' Outstanding Teaching Award and in 2014 he was inducted into the UT Austin Academy of Distinguished Teachers, earning him the title of University Distinguished Teaching Professor. Professor Stone's research interests in Artificial Intelligence include machine learning (especially reinforcement learning), multiagent systems, and robotics.
Professor Stone received his Ph.D. in Computer Science in 1998 from Carnegie Mellon University. From 1999 to 2002 he was a Senior Technical Staff Member in the Artificial Intelligence Principles Research Department at AT&T Labs - Research. He is an Alfred P. Sloan Research Fellow, Guggenheim Fellow, AAAI Fellow, IEEE Fellow, AAAS Fellow, ACM Fellow, Fulbright Scholar, and 2004 ONR Young Investigator. In 2007 he received the prestigious IJCAI Computers and Thought Award, given biannually to the top AI researcher under the age of 35, and in 2016 he was awarded the ACM/SIGAI Autonomous Agents Research Award, and in 2024 he was awarded the ACM/AAAI Allen Newell Award.
Publications
Having explored an environment, intelligent agents should be able to transfer their knowledge to most downstream tasks within that environment. Referred to as ``zero-shot learning," this ability remains elusive for general-purpose reinforcement learning algorithms. While rec…
Scaling up the model size and computation has brought consistent performance improvements in supervised learning. However, this lesson often fails to apply to reinforcement learning (RL) because training the model on non-stationary data easily leads to overfitting and unstab…
Deep reinforcement learning has achieved superhuman racing performance in high-fidelity simulators like Gran Turismo 7 (GT7). It typically utilizes global features that require instrumentation external to a car, such as precise localization of agents and opponents, limiting …
While existing vision and multi-modal foundation models can handle multiple computer vision tasks, they often suffer from significant limitations, including huge demand for data and computational resources during training and inconsistent performance across vision tasks at d…
Recent advances in CV and NLP have been largely driven by scaling up the number of network parameters, despite traditional theories suggesting that larger networks are prone to overfitting. These large networks avoid overfitting by integrating components that induce a simpli…
This work introduces a robotics platform which embeds a conversational AI agent in an embodied system for natural language understanding and intelligent decision-making for service tasks; integrating task planning and human-like conversation. The agent is derived from a larg…
A hallmark of intelligent agents is the ability to learn reusable skills purely from unsupervised interaction with the environment. However, existing unsupervised skill discovery methods often learn entangled skills where one skill variable simultaneously influences many ent…
Unsupervised skill discovery carries the promise that an intelligent agent can learn reusable skills through autonomous, reward-free environment interaction. Existing unsupervised skill discovery methods learn skills by encouraging distinguishable behaviors that cover divers…
Current approaches to learning cooperative multi-agent behaviors assume relatively restrictive settings. In standard fully cooperative multi-agent reinforcement learning, the learning algorithm controls all agents in the scenario, while in ad hoc teamwork, the learning algor…
Many robot manipulation tasks require active or interactive exploration behavior in order to be performed successfully. Such tasks are ubiquitous in embodied domains, where agents must actively search for the information necessary for each stage of a task, e.g., moving the h…
Chain-of-thought (CoT) prompting is a popularin-context learning (ICL) approach for large language models (LLMs), especially when tackling complex reasoning tasks. Traditional ICL approaches construct prompts using examples that contain questions similar to the input questio…
Current approaches to learning cooperative multi-agent behaviors assume relatively restrictive settings. In standard fully cooperative multi-agent reinforcement learning, the learning algorithm controls all agents in the scenario, while in ad hoc teamwork, the learning algor…
The ability to approach the same problem from different angles is a cornerstone of human intelligence that leads to robust solutions and effective adaptation to problem variations. In contrast, current RL methodologies tend to lead to policies that settle on a single solutio…
Racing autonomous cars faster than the best human drivers has been a longstanding grand challenge for the fields of Artificial Intelligence and robotics. Recently, an end-to-end deep reinforcement learning agent met this challenge in a high-fidelity racing simulator, Gran Tu…
Autonomous mobility tasks such as lastmile delivery require reasoning about operator indicated preferences over terrains on which the robot should navigate to ensure both robot safety and mission success. However, coping with out of distribution data from novel terrains or a…
Advances in artificial intelligence (AI) will transform many aspects of our lives and society, bringing immense opportunities but also posing significant risks and challenges. The next several decades may well be a turning point for humanity, comparable to the industrial rev…
We consider algorithms for learning reward functions from human preferences over pairs of trajectory segments, as used in reinforcement learning from human feedback (RLHF). Most recent work assumes that human preferences are generated based only upon the reward accrued withi…
Robustly cooperating with unseen agents and human partners presents significant challenges due to the diverse cooperative conventions these partners may adopt. Existing Ad Hoc Teamwork (AHT) methods address this challenge by training an agent with a population of diverse tea…
Empowering robots to navigate in a socially compliant manner is essential for the acceptance of robots moving in human-inhabited environments. Previously, roboticists have developed geometric navigation systems with decades of empirical validation to achieve safety and effic…
Robotics researchers have been focusing on developing autonomous and human-like intelligent robots that are able to plan, navigate, manipulate objects, and interact with humans in both static and dynamic environments. These capabilities, however, are usually developed for di…
We employ sequences of high-order motion primitives for efficient online trajectory planning, enabling competitive racecar control even when the car deviates from an offline demonstration. Dynamic Movement Primitives (DMPs) utilize a target-driven non-linear differential equ…
Two desiderata of reinforcement learning (RL) algorithms are the ability to learn from relatively little experience and the ability to learn policies that generalize to a range of problem specifications. In factored state spaces, one approach towards achieving both goals is …
Robustly cooperating with unseen agents and human partners presents significant challenges due to the diverse cooperative conventions these partners may adopt. Existing Ad Hoc Teamwork (AHT) methods address this challenge by training an agent with a population of diverse tea…
We consider algorithms for learning reward functions from human preferences over pairs of trajectory segments---as used in reinforcement learning from human feedback (RLHF)---including those used to fine tune ChatGPT and other contemporary language models. Most recent work o…
This paper explores general multi-robot task and motion planning, where multiple robots in close proximity manipulate objects while satisfying constraints and a given goal. In particular, we formulate the plan refinement problem--which, given a task plan, finds valid assignm…
Automatically varying the number of notes in symbolic music has various applications in assisting music creators to embellish simple tunes or to reduce complex music to its core idea. In this paper, we formulate the problem of varying the number of notes while preserving the…
Lifelong learning offers a promising paradigm of building a generalist agent that learns and adapts over its lifespan. Unlike traditional lifelong learning problems in image and text domains, which primarily involve the transfer of declarative knowledge of entities and conce…
One of the grand enduring goals of AI is to create generalist agents that can learn multiple different tasks from diverse data via multitask learning (MTL). However, gradient descent (GD) on the average loss across all tasks may yield poor multitask performance due to severe…
Tasks with large state space and sparse reward present a longstanding challenge to reinforcement learning. In these tasks, an agent needs to explore the state space efficiently until it finds reward: the hard exploration problem. To deal with this problem, the community has …
Goal-Conditioned RL problems provide sparse rewards where the agent receives a reward signal only when it has achieved the goal, making exploration a difficult problem. Several works augment this sparse reward with a learned dense reward function, but this can lead to subopt…
Exoskeleton robots are capable of safe torque-controlled interactions with a wearer while moving their limbs through pre-defined trajectories. However, affecting and assisting the wearer's movements while incorporating their inputs (effort and movements) effectively during a…
In existing task and motion planning (TAMP) research, it is a common assumption that experts manually specify the state space for task-level planning. A well-developed state space enables the desirable distribution of limited computational resources between task planning and…
In existing task and motion planning (TAMP) research, it is a common assumption that experts manually specify the state space for task-level planning. A welldeveloped state space enables the desirable distribution of limited computational resources between task planning an…
Experience replay (ER) is a crucial component of many deep reinforcement learning (RL) systems. However, uniform sampling from an ER buffer can lead to slow convergence and unstable asymptotic behaviors. This paper introduces Stratified Sampling from Event Tables (SSET), whi…
Modern reinforcement learning systems produce many high-quality policies throughout the learning process. However, to choose which policy to actually deploy in the real world, they must be tested under an intractable number of environmental conditions. We introduce RPOSST, a…
Curriculum learning (CL) has been widely explored to facilitate the learning of hard-exploration tasks in reinforcement learning (RL) by training a sequence of easier tasks, often called a curriculum. While most curricula are built either manually or automatically based on h…
Games continue to drive progress in the development of artificial intelligence.
Developing the next generation of household robot helpers requires combining locomotion and interaction capabilities, which is generally referred to as mobile manipulation (MoMa). MoMa tasks are difficult due to the large action space of the robot and the common multi-objec…
Autonomous service robots in a public setting will generate hundreds of incidental human-robot encounters, yet researchers have only recently addressed this important topic in earnest. In this study, we hypothesized that visual indicators of human control, such as a leash on…
Applying reinforcement learning (RL) to sparse reward domains is notoriously challenging due to insufficient guiding signals. Common RL techniques for addressing such domains include (1) learning from demonstrations and (2) curriculum learning. While these two approaches ha…
Human–exoskeleton interactions have the potential to bring about changes in human behavior for physical rehabilitation or skill augmentation. Despite signifcant advances in the design and control of these robots, their application to human training remains limited. The key o…
While combining imitation learning (IL) and reinforcement learning (RL) is a promising way to address poor sample efficiency in autonomous behavior acquisition, methods that do so typically assume that the requisite behavior demonstrations are provided by an expert that be…
Security vulnerabilities in computer systems raise serious concerns as computers process an unprecedented amount of private and sensitive data today. Cachetiming attacks (CTA) pose an important practical threat as they can effectively breach many protection mechanisms in t…
Deep reinforcement learning (RL) has broughtmany successes for autonomous robot navigation. However,there still exists important limitations that prevent real-worlduse of RL-based navigation systems. For example, most learningapproaches lack safety guarantees; and learned na…
While current systems for autonomous robot navigation can produce safe and efficient motion plans in static environments, they usually generate suboptimal behaviors when multiple robots must navigate together in confined spaces. For example, when two robots meet each other i…
Experience replay (ER) is a crucial component of many deep reinforcement learning (RL) systems. However, uniform sampling from an ER buffer can lead to slow convergence and unstable asymptotic behaviors. This paper introduces Stratified Sampling from Event Tables (SSET), whi…
Despite the advancement of machine learning techniques in recent years, state-of-the-art systems lack robustness to “real world” events, where the input distributions and tasks encountered by the deployed systems will not be limited to the original training context, and syst…
This article considers the problem of diagnosing certain common errors in reward design. Its insights are also applicable to the design of cost functions and performance metrics more generally. To diagnose common errors, we develop 8 simple sanity checks for identifying flaw…
Goal-conditioned reinforcement learning (GCRL) has a wide range of potential real-world applications, including manipulation and navigation problems in robotics. Especially in such robotics tasks, sample efficiency is of the utmost importance for GCRL since, by default, the …
In reinforcement learning (RL), a reward function that aligns exactly with a task's true performance metric is often sparse. For example, a true task metric might encode a reward of 1 upon success and 0 otherwise. These sparse task metrics can be hard to learn from, so in pr…
Robots frequently need to perceive object attributes, such as red, heavy, and empty, using multimodal exploratory behaviors, such as look, lift, and shake. One possible way for robots to do so is to learn a classifier for each perceivable attribute given an exploratory behav…
Current approaches to multi-agent cooperation rely heavily on centralized mechanisms or explicit communication protocols to ensure convergence. This paper studies the problem of distributed multi-agent learning without resorting to centralized components or explicit communic…
Bilevel optimization (BO) is useful for solving a variety of important machine learning problems including but not limited to hyperparameter optimization, meta-learning, continual learning, and reinforcement learning. Conventional BO methods need to differentiate through the…
Designing reinforcement learning (RL) agents is typically a difficult process that requires numerous design iterations. Learning can fail for a multitude of reasons and standard RL methods provide too few tools to provide insight into the exact cause. In this paper, we show …
While human-robot interaction studies are becoming more common, quantification of the effects of repeated interaction with an exoskeleton remains unexplored. We draw upon existing literature in human skill assessment and present extrinsic and intrinsic performance metrics t…
Deep reinforcement learning (DRL) agents are trained through trial-and-error interactions with the environment. This leads to a long training time for dense neural networks to achieve good performance. Hence, prohibitive computation and memory resources are consumed. Recentl…
Many potential applications of artificial intelligence involve making real-time decisions in physical systems while interacting with humans. Automobile racing represents an extreme example of these conditions; drivers must execute complex tactical manoeuvres to pass or block…
In this work, we present methods for using human-robot dialog to improve language understanding for a mobile robot agent. The agent parses natural language to underlying semantic meanings and uses robotic sensors to create multi-modal models of perceptual concepts like red a…
The year 2020 saw the covid-19 virus lead to one of the worst global pandemics in history. As a result, governments around the world have been faced with the challenge of protecting public health while keeping the economy running to the greatest extent possible. Epidemiologi…
It has been recently demonstrated that Temporal Convolution Networks (TCNs) provide state-of-the-art results in many problem domains where the input data is a time-series. TCNs typically incorporate information from a long history of inputs (the receptive field) into a singl…
This paper addresses an epidemiologic inference problem where, given realtime observation of test results, presence of symptoms,and physical contacts, the most likely infected individuals need to be inferred. The inference problem is modeled as a hidden Markovmodel where inf…
A desirable goal for autonomous agents is to be able to coordinate on the fly with previously unknown teammates. Known as "ad hoc teamwork", enabling such a capability has been receiving increasing attention in the research community. One of the central challenges in ad hoc …
In continuing tasks, average-reward reinforcement learning may be a more appropriate problem formulation than the more common discounted reward formulation. As usual, learning an optimal policy in this setting typically requires a large amount of training experiences. Reward…
Human-robot shared autonomy techniques for vehicle navigation hold promise for reducing a human driver's workload, ensuring safety, and improving navigation efficiency. However, because typical techniques achieve these improvements by effectively removing human control at cr…
In ad hoc teamwork, multiple agents need to collaborate without having knowledge about their teammates or their plans a priori. A common assumption in this research area is that the agents cannot communicate. However, just as two random people may speak the same language, au…
In multiagent reinforcement learning scenarios, it is often the case that independent agents must jointly learn to perform a cooperative task. This paper focuses on such a scenario in which agents have individual preferences regarding how to accomplish the shared task. We co…
We propose firefly neural architecture descent, a general framework for progressively and dynamically growing neural networks to jointly optimize the networks' parameters and architectures. Our method works in a steepest descent fashion, which iteratively finds the best netw…
We examine the problem of transferring a policy learned in a source environment to a target environment with different dynamics, particularly in the case where it is critical to reduce the amount of interaction with the target environment during learning. This problem is par…
The year 2020 has seen the COVID-19 virus lead to one of the worst global pandemics in history. As a result, governments around the world are faced with the challenge of protecting public health, while keeping the economy running to the greatest extent possible. Epidemiologi…
Blog
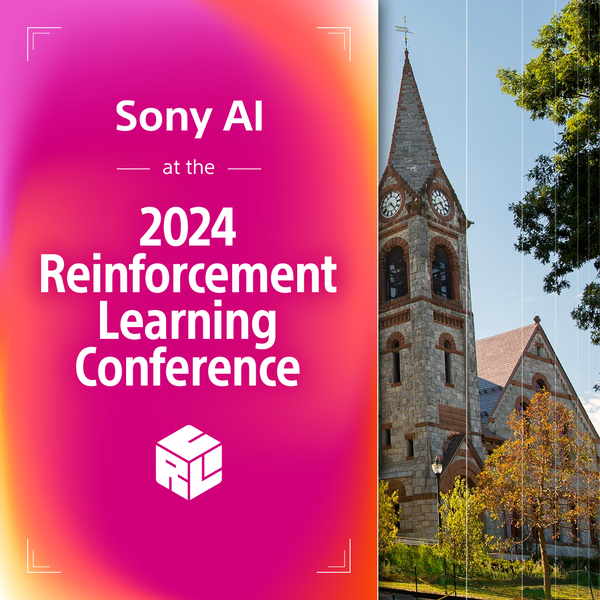
August 10, 2024 | Game AI
Sony AI at the Reinforcement Learning Conference 2024
Sony AI will be participating in the Reinforcement Learning (RL) Conference in Amherst, Massachusetts, from August 9 to 12, 2024 where we will be joining some of the brightest minds in the field—and we are honored to be a part of …
Sony AI will be participating in the Reinforcement Learning (RL) Conference in Amherst, Massachusetts, from August 9 to 12, 2024 w…
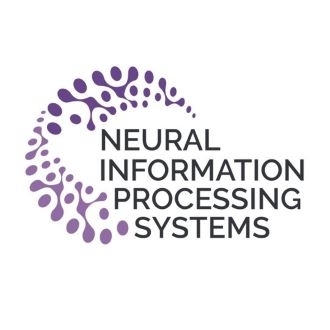
December 13, 2023 | Events
Sony AI Reveals New Research Contributions at NeurIPS 2023
Sony Group Corporation and Sony AI have been active participants in the annual NeurIPS Conference for years, contributing pivotal research that has helped to propel the fields of artificial intelligence and machine learning forwar…
Sony Group Corporation and Sony AI have been active participants in the annual NeurIPS Conference for years, contributing pivotal …
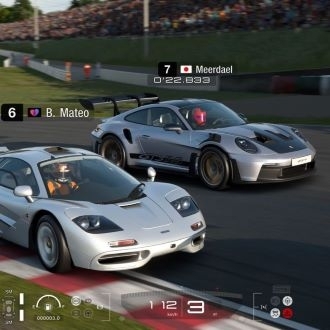
December 5, 2023 | Game AI
RPOSST: Testing an AI Agent for Deployment in the Real World
Bleary-eyed engineers know the anxiety that comes with a deployment, and the importance of testing every aspect of a product before it goes to the “real world.” Will the response time be fast enough? Is there a combination of user…
Bleary-eyed engineers know the anxiety that comes with a deployment, and the importance of testing every aspect of a product befor…
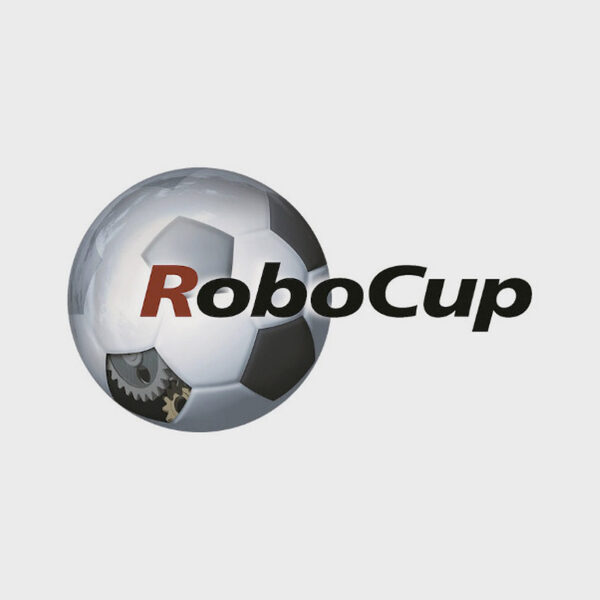
June 17, 2021 | Robotics
RoboCup and Its Role in the History and Future of AI
As I write this blog post, we're a few days away from the opening of the 2021 RoboCup Competitions and Symposium. Running from June 22nd-28th, this event brings together AI and robotics researchers and learners from around the wo…
As I write this blog post, we're a few days away from the opening of the 2021 RoboCup Competitions and Symposium. Running from Jun…
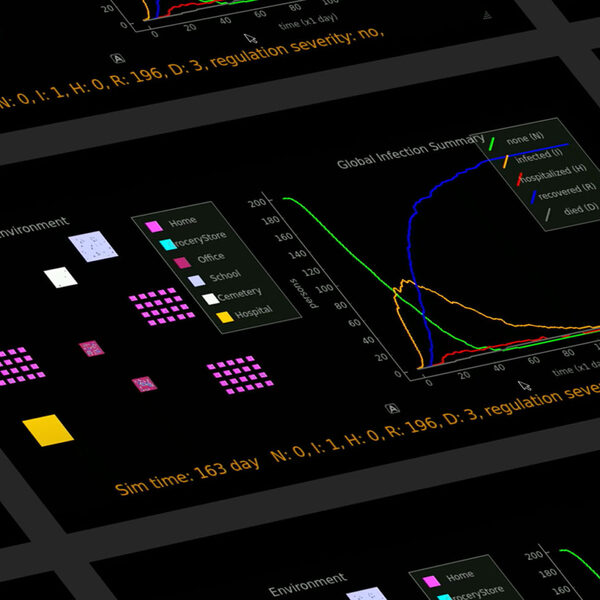
March 3, 2021 | Life at Sony AI
The Challenge to Create a Pandemic Simulator
The thing I like most about working at Sony AI is the quality of the projects we're working on, both for their scientific challenges and for their potential for improving the world. What could be more exciting than magnifying hu…
The thing I like most about working at Sony AI is the quality of the projects we're working on, both for their scientific challen…
News
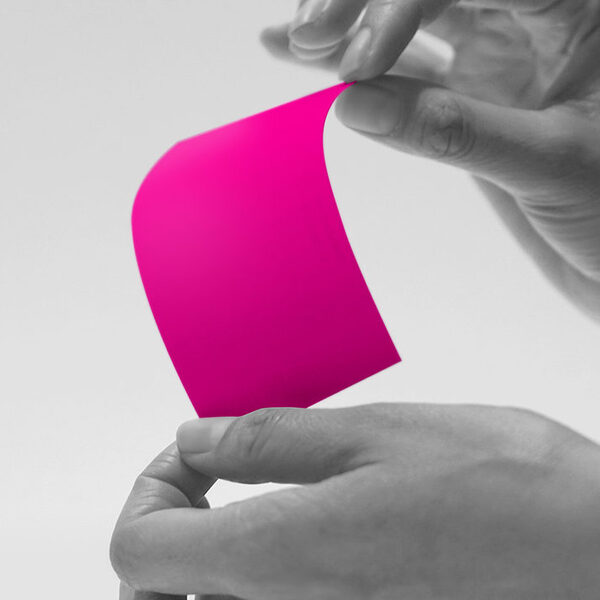
February 4, 2021 | Press Release
Sony AI’s Dr. Peter Stone Named Fellow by the Association for Computing Machiner…
Tokyo, Japan – February 9, 2021 -- Dr. Peter Stone, Executive Director, Sony AI America Inc., has been named a Fellow by the Association for Computing Machinery (ACM), the premier …
JOIN US
Shape the Future of AI with Sony AI
We want to hear from those of you who have a strong desire
to shape the future of AI.