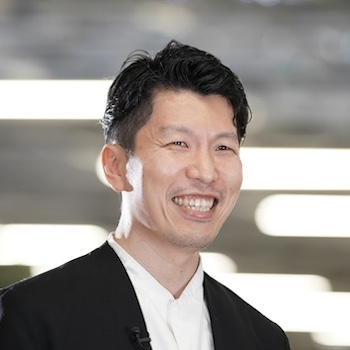
Yuki
Mitsufuji
Publications
Parameter-Efficient Fine-Tuning (PEFT) of text-to-image models has become an increasingly popular technique with many applications. Among the various PEFT methods, Low-Rank Adaptation (LoRA) and its variants have gained significant attention due to their effectiveness, enabl…
We introduce the Robust Audio Watermarking Benchmark (RAW-Bench), a benchmark for evaluating deep learning-based audio watermarking methods with standardized and systematic comparisons. To simulate real-world usage, we introduce a comprehensive audio attack pipeline with var…
Consistency Training (CT) has recently emerged as a promising alternative to diffusion models, achieving competitive performance in image generation tasks. However, non-distillation consistency training often suffers from high variance and instability, and analyzing and impr…
Detecting musical versions (different renditions of the same piece) is a challenging task with important applications. Because of the ground truth nature, existing approaches match musical versions at the track level (e.g., whole song). However, most applications require to …
Diffusion models have demonstrated exceptional performances in various fields of generative modeling, but suffer from slow sampling speed due to their iterative nature. While this issue is being addressed in continuous domains, discrete diffusion models face unique challenge…
We demonstrate the efficacy of using intermediate representations from a single foundation model to enhance various music downstream tasks. We introduce SoniDo, a music foundation model (MFM) designed to extract hierarchical features from target music samples. By leveraging …
By embedding discrete representations into a continuous latent space, we can leverage continuous-space latent diffusion models to handle generative modeling of discrete data. However, despite their initial success, most latent diffusion methods rely on fixed pretrained embed…
In this work, we build a simple but strong baseline for sounding video generation. Given base diffusion models for audio and video, we integrate them with additional modules into a single model and train it to make the model jointly generate audio and video. To enhance align…
This work addresses the lack of multimodal generative models capable of producing high-quality videos with spatially aligned audio. While recent advancements in generative models have been successful in video generation, they often overlook the spatial alignment between audi…
In text-to-motion generation, controllability as well as generation quality and speed has become increasingly critical. The controllability challenges include generating a motion of a length that matches the given textual description and editing the generated motions accordi…
Visual narrative generation transforms textual narratives into sequences of images illustrating the content of the text. However, generating visual narratives that are faithful to the input text and self-consistent across generated images remains an open challenge, due to th…
Deep Generative Models (DGMs), including Energy-Based Models (EBMs) and Score-based Generative Models (SGMs), have advanced high-fidelity data generation and complex continuous distribution approximation. However, their application in Markov Decision Processes (MDPs), partic…
Consistency Training (CT) has recently emerged as a promising alternative to diffusion models, achieving competitive performance in image generation tasks. However, non-distillation consistency training often suffers from high variance and instability, and analyzing and impr…
Diffusion models are prone to exactly reproduce images from the training data. This exact reproduction of the training data is concerning as it can lead to copyright infringement and/or leakage of privacy-sensitive information. In this paper, we present a novel way to unders…
We propose to synthesize high-quality and synchronized audio, given video and optional text conditions, using a novel multimodal joint training framework MMAudio. In contrast to single-modality training conditioned on (limited) video data only, MMAudio is jointly trained wit…
Recent state-of-the-art neural audio compression models have progressively adopted residual vector quantization (RVQ). Despite this success, these models employ a fixed number of codebooks per frame, which can be suboptimal in terms of rate-distortion tradeoff, particularly …
Music timbre transfer is a challenging task that involves modifying the timbral characteristics of an audio signal while preserving its melodic structure. In this paper, we propose a novel method based on dual diffusion bridges, trained using the CocoChorales Dataset, which …
Source separation (SS) of acoustic signals is a research field that emerged in the mid-1990s and has flourished ever since. On the occasion of ICASSP's 50th anniversary, we review the major contributions and advancements in the past three decades in the speech, audio, and mu…
In this work, we build a simple but strong baseline for sounding video generation. Given base diffusion models for audio and video, we integrate them with additional modules into a single model and train it to make the model jointly generate audio and video. To enhance align…
Recent state-of-the-art neural audio compression models have progressively adopted residual vector quantization (RVQ). Despite this success, these models employ a fixed number of codebooks per frame, which can be suboptimal in terms of rate-distortion tradeoff, particularly …
Diffusion models have demonstrated exceptional performances in various fields of generative modeling. While they often outperform competitors including VAEs and GANs in sample quality and diversity, they suffer from slow sampling speed due to their iterative nature. Recently…
Prompt engineering is effective for controlling the output of text-to-image (T2I) generative models, but it is also laborious due to the need for manually crafted prompts. This challenge has spurred the development of algorithms for automated prompt generation. However, thes…
Existing work on pitch and timbre disentanglement has been mostly focused on single-instrument music audio, excluding the cases where multiple instruments are presented. To fill the gap, we propose DisMix, a generative framework in which the pitch and timbre representations …
Sound content is an indispensable element for multimedia works such as video games, music, and films. Recent high-quality diffusion-based sound generation models can serve as valuable tools for the creators. However, despite producing high-quality sounds, these models often …
This paper presents a novel approach to deter unauthorized deepfakes and enable user tracking in generative models, even when the user has full access to the model parameters, by integrating key-based model authentication with watermarking techniques. Our method involves pro…
In typical multimodal contrastive learning, such as CLIP, encoders produce onepoint in the latent representation space for each input. However, one-point representation has difficulty in capturing the relationship and the similarity structure of a huge amount of instances in…
Controllable generation through Stable Diffusion (SD) fine-tuning aims to improve fidelity, safety, and alignment with human guidance. Existing reinforcement learning from human feedback methods usually rely on predefined heuristic reward functions or pretrained reward model…
Personalized text-to-image diffusion models have grown popular for their ability to efficiently acquire a new concept from user-defined text descriptions and a few images. However, in the real world, a user may wish to personalize a model on multiple concepts but one at a ti…
Diffusion models have seen notable success in continuous domains, leading to the development of discrete diffusion models (DDMs) for discrete variables. Despite recent advances, DDMs face the challenge of slow sampling speeds. While parallel sampling methods like -leaping ac…
In this study, we aim to construct an audio-video generative model with minimal computational cost by leveraging pre-trained single-modal generative models for audio and video. To achieve this, we propose a novel method that guides single-modal models to cooperatively genera…
Sound content creation, essential for multimedia works such as video games and films, often involves extensive trial-and-error, enabling creators to semantically reflect their artistic ideas and inspirations, which evolve throughout the creation process, into the sound. Rece…
This technical report presents our submission to the cover song identification task for the 2024 edition of the Music Information Retrieval Evaluation eXchange (MIREX). For this submission, we enhanced our Discogs-VINet model by changing the definition of an epoch, incorpora…
To accelerate sampling, diffusion models (DMs) are often distilled into generators that directly map noise to data in a single step. In this approach, the resolution of the generator is fundamentally limited by that of the teacher DM. To overcome this limitation, we propose …
Generating novel views from a single image remains a challenging task due to the complexity of 3D scenes and the limited diversity in the existing multi-view datasets to train a model on. Recent research combining large-scale text-to-image (T2I) models with monocular depth e…
This paper presents the crossing scheme (X-scheme) for improving the performance of deep neural network (DNN)-based music source separation (MSS) with almost no increasing calculation cost. It consists of three components: (i) multi-domain loss (MDL), (ii) bridging operation…
Contrastive cross-modal models such as CLIP and CLAP aid various vision-language (VL) and audio-language (AL) tasks. However, there has been limited investigation of and improvement in their language encoder, which is the central component of encoding natural language descri…
Inferring contextually-relevant and diverse commonsense to understand narratives remains challenging for knowledge models. In this work, we develop a series of knowledge models, DiffuCOMET, that leverage diffusion to learn to reconstruct the implicit semantic connections bet…
Recent advances in generative models that iteratively synthesize audio clips sparked great success to text-to-audio synthesis (TTA), but with the cost of slow synthesis speed and heavy computation. Although there have been attempts to accelerate the iterative procedure, high…
Recent advancements in music generation are raising multiple concerns about the implications of AI in creative music processes, current business models and impacts related to intellectual property management. A relevant challenge is the potential replication and plagiarism o…
In the realm of audio watermarking, it is challenging to simultaneously encode imperceptible messages while enhancing the message capacity and robustness. Although recent advancements in deep learning-based methods bolster the message capacity and robustness over traditional…
Music mixing is compositional -- experts combine multiple audio processors to achieve a cohesive mix from dry source tracks. We propose a method to reverse engineer this process from the input and output audio. First, we create a mixing console that applies all available pro…
Generative adversarial network (GAN)-based vocoders have been intensively studied because they can synthesize high-fidelity audio waveforms faster than real-time. However, it has been reported that most GANs fail to obtain the optimal projection for discriminating between re…
Vector quantization (VQ) is a technique to deterministically learn features with discrete codebook representations. It is commonly performed with a variational autoencoding model, VQ-VAE, which can be further extended to hierarchical structures for making high-fidelity recon…
Generative adversarial network (GAN)-based vocoders have been intensively studied because they can synthesize high-fidelity audio waveforms faster than real-time. However, it has been reported that most GANs fail to obtain the optimal projection for discriminating between re…
Diffusion-based speech enhancement (SE) has been investigated recently, but its decoding is very time-consuming. One solution is to initialize the decoding process with the enhanced feature estimated by a predictive SE system. However, this two-stage method ignores the compl…
Restoring degraded music signals is essential to enhance audio quality for downstream music manipulation. Recent diffusion-based music restoration methods have demonstrated impressive performance, and among them, diffusion posterior sampling (DPS) stands out given its intrin…
Sound event localization and detection (SELD) systems estimate direction-of-arrival (DOA) and temporal activation for sets of target classes. Neural network (NN)-based SELD systems have performed well in various sets of target classes, but they only output the DOA and tempor…
In recent years, research on music transcription has focused mainly on architecture design and instrument-specific data acquisition. With the lack of availability of diverse datasets, progress is often limited to solo-instrument tasks such as piano transcription. Several wor…
Generative adversarial networks (GANs) learn a target probability distribution by optimizing a generator and a discriminator with minimax objectives. This paper addresses the question of whether such optimization actually provides the generator with gradients that make its d…
Despite the recent advancements, conditional image generation still faces challenges of cost, generalizability, and the need for task-specific training. In this paper, we propose Manifold Preserving Guided Diffusion (MPGD), a training-free conditional generation framework th…
Consistency Models (CM) (Song et al., 2023) accelerate score-based diffusion model sampling at the cost of sample quality but lack a natural way to trade-off quality for speed. To address this limitation, we propose Consistency Trajectory Model (CTM), a generalization encomp…
Semantic communication is poised to play a pivotal role in shaping the landscape of future AI-driven communication systems. Its challenge of extracting semantic information from the original complex content and regenerating semantically consistent data at the receiver, possi…
Generative adversarial network (GAN)-based vocoders have been intensively studied because they can synthesize high-fidelity audio waveforms faster than real-time. However, it has been reported that most GANs fail to obtain the optimal projection for discriminating between re…
Diffusion-based speech enhancement (SE) has been investigated recently, but its decoding is very time-consuming. One solution is to initialize the decoding process with the enhanced feature estimated by a predictive SE system. However, this two-stage method ignores the compl…
Restoring degraded music signals is essential to enhance audio quality for downstream music manipulation. Recent diffusion-based music restoration methods have demonstrated impressive performance, and among them, diffusion posterior sampling (DPS) stands out given its intrin…
Sound event localization and detection (SELD) systems estimate direction-of-arrival (DOA) and temporal activation for sets of target classes. Neural network (NN)-based SELD systems have performed well in various sets of target classes, but they only output the DOA and tempor…
In recent years, research on music transcription has focused mainly on architecture design and instrument-specific data acquisition. With the lack of availability of diverse datasets, progress is often limited to solo-instrument tasks such as piano transcription. Several wor…
While direction of arrival (DOA) of sound events is generally estimated from multichannel audio data recorded in a microphone array, sound events usually derive from visually perceptible source objects, e.g., sounds of footsteps come from the feet of a walker. This paper pro…
Taking long-term spectral and temporal dependencies into account is essential for automatic piano transcription. This is especially helpful when determining the precise onset and offset for each note in the polyphonic piano content. In this case, we may rely on the capabilit…
Audio classification and restoration are among major downstream tasks in audio signal processing. However, restoration derives less of a benefit from pretrained models compared to the overwhelming success of pretrained models in classification tasks. Due to such unbalanced b…
This paper presents the crossing scheme (X-scheme) for improving the performance of deep neural network (DNN)-based music source separation (MSS) without increasing calculation cost. It consists of three components: (i) multi-domain loss (MDL), (ii) bridging operation, which…
Although deep neural network (DNN)-based speech enhancement (SE) methods outperform the previous non-DNN-based ones, they often degrade the perceptual quality of generated outputs. To tackle this problem, we introduce a DNN-based generative refiner, Diffiner, aiming to impro…
Pre-trained diffusion models have been successfully used as priors in a variety of linear inverse problems, where the goal is to reconstruct a signal from noisy linear measurements. However, existing approaches require knowledge of the linear operator. In this paper, we prop…
Score-based generative models learn a family of noise-conditional score functions corresponding to the data density perturbed with increasingly large amounts of noise. These perturbed data densities are tied together by the Fokker-Planck equation (FPE), a partial differentia…
We propose an end-to-end music mixing style transfer system that converts the mixing style of an input multitrack to that of a reference song. This is achieved with an encoder pre-trained with a contrastive objective to extract only audio effects related information from a r…
In this paper we propose a novel generative approach, DiffRoll, to tackle automatic music transcription (AMT).Instead of treating AMT as a discriminative task in which the model is trained to convert spectrograms into piano rolls, we think of it as a conditional generative t…
Recent progress in deep generative models has improved the quality of neural vocoders in speech domain. However, generating a high-quality singing voice remains challenging due to a wider variety of musical expressions in pitch, loudness, and pronunciations. In this work, we…
Removing reverb from reverberant music is a necessary technique to clean up audio for downstream music manipulations. Reverberation of music contains two categories, natural reverb, and artificial reverb. Artificial reverb has a wider diversity than natural reverb due to its…
Recent years have seen progress beyond domain-specific sound separation for speech or music towards universal sound separation for arbitrary sounds. Prior work on universal sound separation has investigated separating a target sound out of an audio mixture given a text query…
One noted issue of vector-quantized variational autoencoder (VQ-VAE) is that the learned discrete representation uses only a fraction of the full capacity of the codebook, also known as codebook collapse. We hypothesize that the training scheme of VQ-VAE, which involves some…
Blog
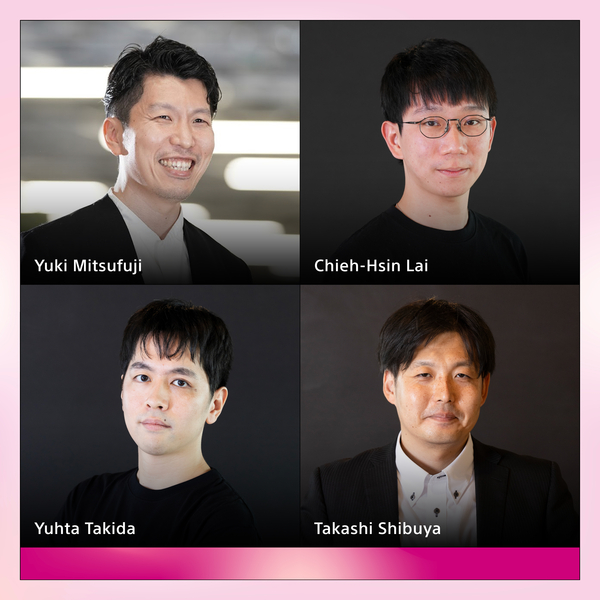
May 10, 2024 | Events | Sony AI
Revolutionizing Creativity with CTM and SAN: Sony AI's Groundbreaking Advances in Generative AI for Creators
In the dynamic world of generative AI, the quest for more efficient, versatile, and high-quality models continues to push forward without any reduction in intensity. At the forefront of this technological evolution are Sony AI's r…
In the dynamic world of generative AI, the quest for more efficient, versatile, and high-quality models continues to push forward …
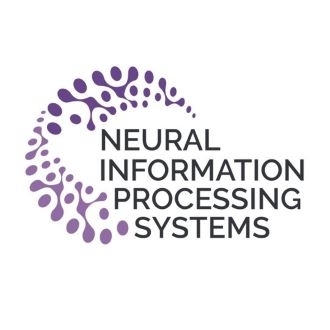
December 13, 2023 | Events
Sony AI Reveals New Research Contributions at NeurIPS 2023
Sony Group Corporation and Sony AI have been active participants in the annual NeurIPS Conference for years, contributing pivotal research that has helped to propel the fields of artificial intelligence and machine learning forwar…
Sony Group Corporation and Sony AI have been active participants in the annual NeurIPS Conference for years, contributing pivotal …
JOIN US
Shape the Future of AI with Sony AI
We want to hear from those of you who have a strong desire
to shape the future of AI.