From Data Fairness to 3D Image Generation: Sony AI at NeurIPS 2024
Events
December 13, 2024
The 38th Annual Conference on Neural Information Processing Systems is fast approaching. NeurIPS 2024, the largest AI conference in the world, is taking place this year at the Vancouver Convention Center, Vancouver B.C. on Tuesday, December 10 through Sunday, December 15.
This year's conference promises groundbreaking developments across diverse fields, from generative AI to privacy-preserving machine learning. Sony AI is proud to showcase its research contributions to the conversation, spanning music and audio synthesis, skill discovery, federated learning, and beyond. Here’s a glimpse at the research we’re presenting, setting the stage for transformative ideas and innovation in AI.
Don’t miss presentations at our booth and more information about our work: NeurIPS 2024 Schedule
Accepted Research Round-Up
AI Ethics
Data Fairness
Oral presentation
Sony AI’s paper accepted at NeurIPS 2024, ” A Taxonomy of Challenges to Curating Fair Datasets,” highlights the pivotal steps toward achieving fairness in machine learning and is a continuation of our team’s deep explorations into these issues. This research also marks an evolution of the work from empirical inquiry into theory, taking a Human-Computer Interaction (HCI) approach to the issues. Read the full blog post to learn more.
3D Image Generation, The Complexities of Pitch and Timbre in Music Mixing, Generative AI Watermarking and Audio Compression
Research in the areas of generative AI, sound, and audio are moving rapidly, but there are still gaps in its ability to support professional human artistry. Our explorations aim to uncover novel approaches and tools to empower creators, offering them the ability to refine and shape their output in real-time with a better understanding of how AI can support the complexities of pitch and timbre in audio mixing, or reduce memory demands that have inhibited real-time text-to-sound generation.
Our work shows new pathways to deter unauthorized use of audio files and facilitate user tracking in generative models by integrating key-based authentication with watermarking techniques. See more details on our NeurIPS papers and workshops below.
LOCKEY: Securing Generative Models
Authors: Mayank Kumar Singh, Naoya Takahashi, Wei-Hsiang Liao, Yuki Mitsufuji
The Problem
Generative AI models are vulnerable to misuse and deepfake creation, with inadequate existing safeguards.
The Solution
LOCKEY uses key-based authentication, embedding user-specific watermarks for secure, traceable outputs.
The Challenge
Ensuring watermarks are robust, outputs stay high-quality, and scaling to numerous unique keys.
The Result
LOCKEY secures generative models while maintaining audio quality, setting a new benchmark for AI security.
Read the full research paper
Explore the Demo
DisMix: Mastering Music Mixes
Authors: Yin-Jyun Luo, Kin Wai Cheuk, Woosung Choi, Toshimitsu Uesaka, Keisuke Toyama, Koichi Saito, Chieh-Hsin Lai, Yuhta Takida, Wei-Hsiang Liao, Simon Dixon, Yuki Mitsufuji
The Problem
Pitch-timbre disentanglement for multi-instrument mixtures remained unaddressed, limiting creative possibilities.
The Solution
DisMix separates and recombines pitch and timbre using latent diffusion transformers and binarization layers.
The Challenge
Avoiding pitch-timbre overlap and reconstructing complex audio mixtures realistically.
The Result
DisMix delivers precise pitch-timbre manipulation, opening new avenues for creative audio applications.
Read the full research paper
View Samples
SoundCTM: Faster Text-to-Sound Generation
Authors: Koichi Saito, Zhi Zhong, Dongjun Kim, Yuhta Takida, Takashi Shibuya, Chieh-Hsin Lai
The Problem
Slow inference speeds in T2S models hinder real-time applications in creative workflows.
The Solution
SoundCTM combines score-based and consistency models, enabling flexible and efficient T2S generation.
The Challenge
Balancing speed with quality while reducing memory demands and ensuring adaptability.
The Result
SoundCTM accelerates sound generation with flexibility, offering unprecedented creative control.
Read the full research
View Samples
PRISM: Smarter Prompts for Text-to-Image
Authors: Yutong He, Alexander Robey, Naoki Murata, Yiding Jiang, Joshua Williams, George J. Pappas, Hamed Hassani, Yuki Mitsufuji, Ruslan Salakhutdinov, J. Zico Kolter
The Problem
Manual prompt engineering for T2I models is tedious, with limited transferability to closed-source systems.
The Solution
PRISM refines prompts iteratively using LLMs and feedback from generated and reference images.
The Challenge
Maintaining interpretability, transferability, and effectiveness across diverse T2I models.
The Result
PRISM creates accurate, interpretable prompts, making T2I workflows faster and more accessible.
Di4C: Accelerating Discrete Diffusion
Authors: Satoshi Hayakawa†∗ Yuhta Takida‡ Masaaki Imaizumi§ Hiromi Wakaki† Yuki Mitsufuji†‡
The Problem
Discrete diffusion models are slow, ignoring dimensional correlations that could streamline generation.
The Solution
Di4C distills multi-step processes into fewer steps, leveraging loss functions and mixture modeling.
The Challenge
Preserving accuracy while compressing processes and scaling for high-dimensional data.
The Result
Di4C boosts efficiency, cutting sampling times while maintaining high-quality outputs.
VRVQ: Smarter Audio Compression
Authors: Yunkee Chae1,3∗ Woosung Choi1 Yuhta Takida1 Junghyun Koo1 Yukara Ikemiya1 Zhi Zhong2 Kin Wai Cheuk1 Marco A. Martínez-Ramírez1 Kyogu Lee3,4,5 Wei-Hsiang Liao1 Yuki Mitsufuji1,2
The Problem
Constant bitrate audio compression wastes resources on simple inputs like silence.
The Solution
VRVQ introduces an “importance map” for dynamic bit allocation and efficient training.
The Challenge
Designing robust importance maps and handling masking functions within RVQ architectures.
The Result
VRVQ achieves better rate-distortion tradeoffs, setting a new standard for neural audio codecs.
Discovering Creative Behaviors through Reinforcement Learning (RL)
At NeurIPS 2024, Sony AI highlights the transformative potential of reinforcement learning (RL) through research designed to push beyond traditional limitations. A central focus is on advancing RL’s adaptability in dynamic and complex environments. These efforts explore how RL agents can balance diverse, context-specific strategies with optimal performance, unlocking new possibilities in applications like gaming, robotics, and beyond.
One key contribution, DUPLEX, introduces a diversity objective and successor features, enabling RL agents to discover multiple near-optimal policies rather than converging on single solutions. Demonstrated in Gran Turismo™ 7 and robotics tasks, DUPLEX sets a new benchmark for diversity-focused RL by achieving adaptive and effective behaviors in out-of-distribution scenarios. This work underscores Sony AI’s commitment to advancing RL methodologies for real-world challenges.
DUPLEX
Authors: Borja G. Leon, Francesco Riccio, Kaushik Subramanian, Pete Wurman, Peter Stone
The Problem
Reinforcement learning (RL) agents tend to settle on single solutions, limiting adaptability to diverse and dynamic environments.
The Solution
DUPLEX introduces a diversity objective and successor features, enabling agents to discover multiple near-optimal policies tailored to specific contexts.
The Challenge
Balancing the trade-off between diversity and optimality in highly dynamic or out-of-distribution (OOD) tasks like driving simulators and robotics.
The Result
DUPLEX achieved diverse driving styles in Gran Turismo™ 7 and effective policies in OOD robotics scenarios, setting a new benchmark for diversity-focused RL.
Privacy-Preserving Machine Learning
Sony AI’s research in privacy-preserving machine learning tackles critical challenges at the intersection of data security, utility, and compliance. As privacy regulations tighten and datasets become more sensitive, the need for robust frameworks to protect data while enabling meaningful AI applications has grown. This research introduces innovative solutions that address these complexities, including benchmarks for evaluating privacy-utility trade-offs, frameworks for federated learning across heterogeneous models, and tools for enhancing privacy in recommender systems and medical AI. Together, these advancements not only safeguard sensitive information but also set new standards for scalable, privacy-compliant AI development.
DECO-Bench: Unified Benchmark for Privacy-Preserving Data
Authors: Farzaneh Askari, Lingjuan Lyu, Vivek Sharma
The Problem
Sharing datasets containing sensitive information risks privacy breaches, and current decoupling methods lack a standardized benchmark to evaluate their effectiveness.
The Solution
DECO-Bench introduces a comprehensive framework to benchmark decoupling techniques, integrating synthetic data generation, structured data splits, and evaluation metrics for privacy-utility trade-offs.
The Challenge
Ensuring sensitive attributes are anonymized while maintaining non-sensitive data utility, balancing trade-offs in complex real-world datasets.
The Result
DECO-Bench enables systematic evaluation of decoupling methods, releasing datasets, pre-trained models, and metrics to accelerate privacy-preserving research and create a standard for task-agnostic synthetic data benchmarks.
pFedClub: Personalized Federated Learning at Scale
Authors: Jiaqi Wang, Qi Li, Lingjuan Lyu, Fenglong Ma
The Problem
Federated learning often requires uniform model structures across clients, limiting real-world applications where diverse client models and personalized needs are common.
The Solution
pFedClub introduces a novel block substitution and aggregation method, creating personalized models by clustering functional blocks from heterogeneous client models and selecting optimal configurations.
The Challenge
Balancing the creation of personalized models while managing computational costs, maintaining privacy, and ensuring compatibility with varying client resources.
The Result
pFedClub outperformed state-of-the-art methods in accuracy and efficiency, generating size-controllable personalized models suitable for diverse federated learning scenarios without compromising computational or communication resources.
Read the full research paper
The source code can be found at https://github.com/JackqqWang/24club
CURE4Rec: Benchmarking Privacy in Recommender Systems
Authors: Chaochao Chen, Jiaming Zhang, Yizhao Zhang, Li Zhang, Lingjuan Lyu, Yuyuan Li, Biao Gong, Chenggang Yan
The Problem
Current recommendation unlearning methods lack a unified evaluation framework, overlooking key aspects like fairness and efficiency while addressing privacy regulations like GDPR and the Delete Act.
The Solution
CURE4Rec provides a comprehensive benchmark to evaluate unlearning methods across four dimensions: completeness, utility, efficiency, and fairness, using varying data selection strategies such as core, edge, and random data.
The Challenge
Balancing unlearning completeness with computational efficiency, preserving utility for remaining data, and ensuring fairness in recommendations across diverse user groups.
The Result
CURE4Rec establishes a new standard for evaluating privacy-preserving recommender systems, enabling systematic improvement in unlearning methods while ensuring fairness and robustness against diverse data challenges.
Read the full research paper
Our code is available at https://github.com/xiye7lai/CURE4Rec
FEDMEKI: Advancing Privacy-Preserving Medical AI
Authors: Jiaqi Wang, Xiaochen Wang, Lingjuan Lyu, Jinghui Chen, Fenglong Ma
The Problem
Medical foundation models struggle to integrate knowledge from sensitive, decentralized datasets due to privacy regulations like HIPAA.
The Solution
FEDMEKI introduces a federated knowledge injection platform, enabling multi-modal, multi-task learning from distributed medical data without sharing private information.
The Challenge
Adapting decentralized client data into a unified foundation model while preserving privacy and ensuring scalability across diverse medical modalities.
The Result
FEDMEKI achieved state-of-the-art performance in privacy-preserving model scaling, supporting eight diverse medical tasks and demonstrating effective zero-shot generalization.
Read the full research paper
Code is available at https://github.com/psudslab/FEDMEKI
Research Contributions Across Our Leadership and Research Teams
Sony AI’s research achievements reflect the collective expertise of our leadership and researchers, many of whom bridge roles between industry and academia. This synergy enables groundbreaking advancements across fields such as reinforcement learning, skill discovery, and analog AI training. By tackling foundational challenges—like enabling multi-agent collaboration, uncovering meaningful skills in complex environments, and refining energy-efficient AI methods—our teams are driving innovation that addresses both theoretical and practical hurdles in AI. These contributions exemplify the breadth of our efforts to shape a more capable and adaptable future for artificial intelligence and showcase the capabilities of our diverse global team.
Consent in Crisis: The Rapid Decline of the AI Data Commons
The Problem
The internet has long served as the primary "data commons" for AI systems, with vast web datasets fueling advancements in general-purpose AI. However, the mechanisms for signaling data consent, such as robots.txt files and Terms of Service, were not designed with AI in mind. A growing number of web domains are imposing restrictions on data collection for AI training, leading to inconsistencies and limitations in available training data.
The Solution
This research conducted a large-scale, longitudinal audit of 14,000 web domains used in major AI corpora, such as C4, RefinedWeb, and Dolma. It examined the evolution of consent protocols, including robots.txt and Terms of Service, to diagnose the mismatch between traditional data use protocols and AI's reliance on web content.
The Challenge
Balancing the need for open data in AI development with emerging legal and ethical concerns. Restrictions are increasingly skewing data diversity, representativity, and freshness, jeopardizing scaling laws and the quality of AI training corpora.
The Result
The study found a significant rise in restrictive practices. In just one year, ~5% of tokens in major datasets became restricted by robots.txt, and nearly 45% carried restrictions in Terms of Service. These restrictions disproportionately impact high-quality, actively maintained web sources, threatening data availability for both commercial and non-commercial AI initiatives.
Read the full research paper
Additional Details
POAM: Dynamic Multi-Agent Collaboration
Authors: Caroline Wang, Arrasy Rahman, Ishan Durugkar, Elad Liebman, Peter Stone
The Problem
Existing MARL methods assume either full control or single-agent adaptation, failing to address scenarios with mixed controlled and uncontrolled agents.
The Solution
POAM uses an encoder-decoder network to model teammate behavior and dynamically adapt agent policies based on learned embeddings.
The Challenge
Balancing generalization to unseen teammates, managing diverse team compositions, and ensuring efficient learning in partially observable environments.
The Result
POAM outperformed baselines in adaptability and sample efficiency, excelling in tasks like StarCraft and predator-prey scenarios while generalizing to new teammates.
Read the full research paper
Code available here
SkiLD: Smarter Skill Discovery
Authors: Zizhao Wang, Jiaheng Hu, Caleb Chuck, Stephen Chen, Roberto Martin, Amy Zhang, Scott Niekum, Peter Stone
The Problem
Unsupervised skill discovery methods fail in complex environments, often producing overly simplistic skills that struggle with tasks requiring interactions between state factors.
The Solution
SkiLD introduces state-specific dependency graphs to guide skill discovery, encouraging diverse interactions between factors for meaningful downstream applications.
The Challenge
Balancing the complexity of learning interaction-driven skills with ensuring efficient exploration in vast state spaces.
The Result
SkiLD outperformed existing methods in complex environments, producing semantically rich skills that excelled in long-horizon tasks like robotic manipulation and household activities.
Read the full research paper
Code and visualizations
DUSDi: Disentangling Skills for Smarter Reinforcement Learning
Authors: Jiaheng Hu, Zizhao Wang, Roberto Martín-Martín, Peter Stone
The Problem
Existing skill discovery methods produce entangled skills, making it difficult for agents to solve complex tasks involving independent state factors.
The Solution
DUSDi introduces disentangled skills by mapping skill components to independent state factors, leveraging mutual information objectives to ensure minimal overlap between skill effects.
The Challenge
Balancing efficient learning of disentangled skills while addressing the complexity of environments with multiple state factors and ensuring effective downstream task performance.
The Result
DUSDi outperformed state-of-the-art methods in complex tasks, enabling agents to tackle multi-factor challenges efficiently through disentangled, reusable skills for hierarchical reinforcement learning.
Read the full research paper
Code and skills visualization
Tiki-Taka: Refining Analog AI Training
Authors: Zhaoxian Wu, Malte J. Rasch, Tayfun Gokmen, Tianyi Chen
The Problem
Analog in-memory computing accelerators reduce energy costs but face challenges with noise and asymmetric updates during gradient-based training, leading to suboptimal performance.
The Solution
Tiki-Taka introduces an auxiliary device to correct noise and asymmetric updates, ensuring convergence to critical points with improved accuracy.
The Challenge
Addressing the asymmetry in weight updates while managing noise effectively across analog accelerators without increasing computational costs.
The Result
Tiki-Taka eliminates asymptotic errors, outperforming Analog SGD with faster convergence and accuracy comparable to digital training, making analog AI training more viable.
The digital algorithm, including SGD, and dataset used in this paper, including MNIST and CIFAR10, are provided by PyTorch, which has a BSD license; see https://github.com/pytorch/pytorch
Simulate the behaviors of Analog SGD; see github.com/IBM/aihwkit
Read the full research paper
Conclusion
NeurIPS 2024 highlights the rapid pace of AI advancements and the challenges that come with them. Sony AI's contributions this year are a testament to our commitment to pushing boundaries while addressing pressing concerns like security, efficiency, and creativity. Whether it’s securing generative models, accelerating diffusion processes, or pioneering smarter multi-agent systems, we aim to inspire and drive progress across the AI landscape. Join us at NeurIPS to explore these ideas further and help shape the future of artificial intelligence.
Latest Blog
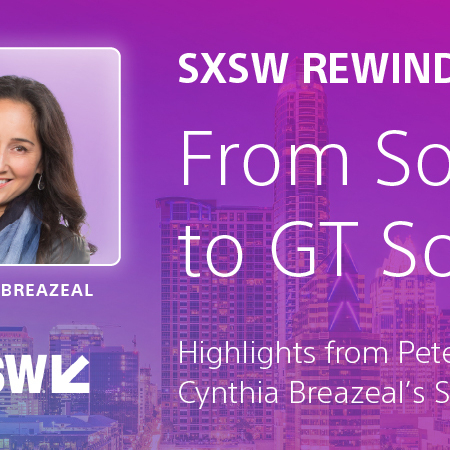
June 17, 2025 | Events, Sony AI
SXSW Rewind: From GT Sophy to Social Robots—Highlights from Peter Stone and Cynt…
While SXSW 2025 may now be in the rearview mirror, the conversations it ignited continue to resonate. On March 10, 2025, Peter Stone, Chief Scientist at Sony AI and Professor at Th…
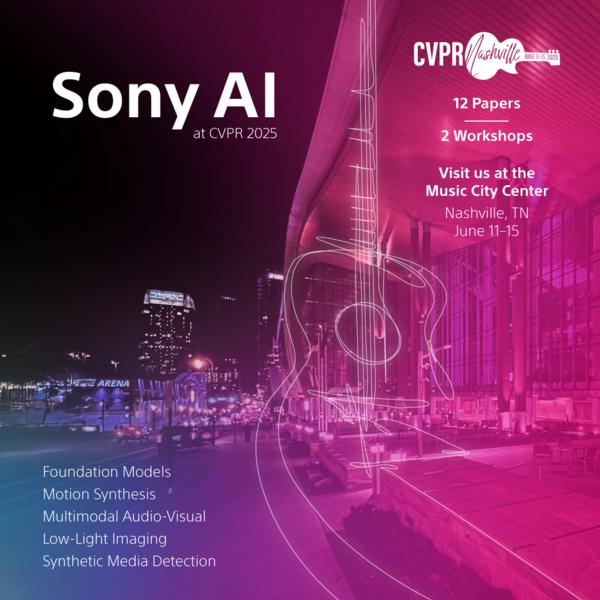
June 12, 2025 | Events, Sony AI
Research That Scales, Adapts, and Creates: Spotlighting Sony AI at CVPR 2025
At this year’s IEEE / CVF Computer Vision and Pattern Recognition Conference (CVPR) in Nashville, TN Sony AI is proud to present 12 accepted papers spanning the main conference and…
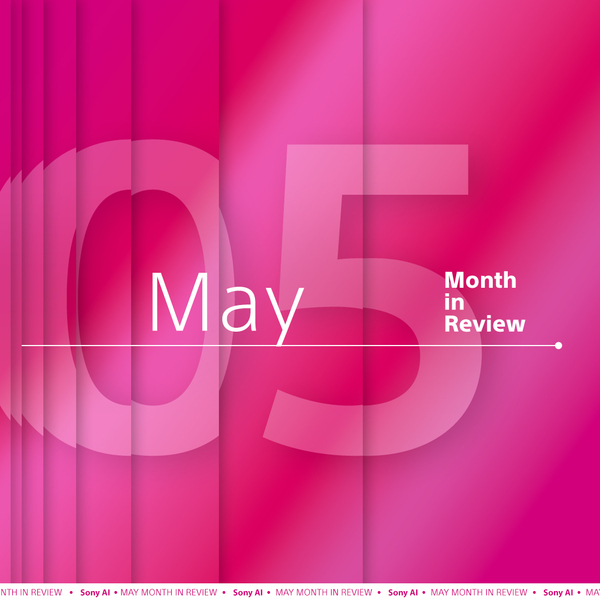
June 3, 2025 | Sony AI
Advancing AI: Highlights from May
From research milestones to conference prep, May was a steady month of progress across Sony AI. Our team's advanced work in vision-based reinforcement learning, continued building …