Revolutionizing Creativity with CTM and SAN: Sony AI's Groundbreaking Advances in Generative AI for Creators
Events
Sony AI
May 10, 2024
In the dynamic world of generative AI, the quest for more efficient, versatile, and high-quality models continues to push forward without any reduction in intensity. At the forefront of this technological evolution are Sony AI's recent contributions: the Consistency Trajectory Model (CTM) and the Slicing Adversarial Network (SAN). Both models have marked their presence with a strong endorsement at ICLR 2024, reflecting their potential to reshape the landscape of AI-driven creativity. This year, we are particularly proud to announce that three of our papers were accepted, each representing major technological breakthroughs in generative AI.
Deep generative models are transforming approaches to not just creativity, but data synthesis, and problem-solving with artificial intelligence as well. This rapidly evolving field of deep learning continues to evolve with new research achieving state-of-the-art results.
Sony AI’s recent discoveries in deep generative modeling are creating a novel family of multi-purpose models that extend beyond traditional score-based models and distillation models and improve on the leading GAN and VAE models in efficiency and stability. Text and image generation pose different and often less complex elements than models that can be useful for tasks in audio, sound and music where considerations of polyphony, other instruments as well as harmony make it a much harder problem to tackle and one that has not received as much attention.
CTM and SAN both achieved state-of-the-art (SOTA) generation (on standard academic datasets CIFAR-10 and ImageNet-64, as well as speech synthesis on LibriTTS.) when tested against similar popular deep generative models. These models stand out as new powerful one-step generation methods. The Sony AI team considered the real-time efficiency and granular control needed for professional creators, finding new approaches are possible and are applicable to a variety of other content types such as audio, video, 3D, and others.
Though CTM and SAN were tested on common image data sets, the team is exploring deep generative models as effective tools for real-time tasks in audio, video and 3D among others.
The research team at Sony AI is led by Yuki Mitsufuji who has been exploring the impact of AI on music and sound for more than 15 years. The team approached deep generative models through the lens of the challenges in developing models for the audio and music field. Deep generative models for music and audio in particular face challenges in maintaining temporal coherence, generating diverse and creative outputs and allowing for real time user control. Developers have struggled to find the optimal trade-off between speed and quality and these models move closer to solving these problems.
Generative AI development is one of the hottest and most competitive areas of research at this time, getting three out of three papers accepted is a nod to the novel and transformative developments in these papers.
Understanding CTM: A Leap in Generative Modeling
The Consistency Trajectory Model (CTM) (Authors: Dongjun Kim, Chieh-Hsin Lai, Wei-Hsiang Liao, Naoki Murata, Yuhta Takida, Toshimitsu Uesaka, Yutong He, Yuki Mitsufuji, Stefano Ermon) represents a significant advancement in the field of deep generative models. Built on the foundation of Consistency Models and score-based diffusion models, CTM introduces a novel approach that accelerates the sampling process while maintaining unparalleled sample quality.
Methodology
CTM operates through a unique framework that utilizes a single neural network to unify diffusion model and diffusion distillation-based method (e.g., Consistency Model).
“To address the challenges in both score-based and distillation samplings, we introduce the Consistency Trajectory Model (CTM), which integrates both decoding strategies to sample from either SDE/ODE solving or direct anytime-to-anytime jump along the PF ODE trajectory,” the paper authors explain.
In other words, this facilitates an efficient and unrestricted traversal along the Probability Flow Ordinary Differential Equation (ODE) in a diffusion process, allowing for "long jumps" along the ODE solution trajectories which consistently improve sample quality as computational budgets increase.
Why It's Groundbreaking
This model achieves remarkable efficiency by combining adversarial training with denoising score-matching loss—a hybrid approach that enhances performance across various benchmarks. CTM has demonstrated its potential by setting new state-of-the-art Frechet Inception Distance (FID) scores on datasets like CIFAR-10 and ImageNet, opening possibilities as a tool for creators seeking speed without sacrificing quality.
CTM can serve as the backbone of a foundational model for music. It can not only deliver fast and high-quality music generation but also be utilized to speed up all music applications including music restoration, music source separation, auto-bridging of music, and more, all in real-time. As the generation is in real-time, creators can instantly interact with the model. In the long term, CTM can significantly support music developers' work and streamline the production pipeline, allowing music producers to focus more on creative activities.
Implications for CTM and Professional Use
From the perspective of professionals, this tool can address some of the data duplication and collapse issues that have plagued current methods. The quest for creating deep generative models that strike the perfect balance between speed and quality has been an ongoing challenge.
CTM represents a monumental leap forward in the realm of deep generative models. It builds upon the foundation laid by Consistency Models (CM) by introducing an approach that not only accelerates the sampling process but also maintains a high level of sample quality.
Traditionally, deep generative models have struggled to find the optimal trade-off between speed and quality. While CMs expedite the sampling process, they often compromise on sample quality. Conversely, CTM offers a groundbreaking solution by training a single neural network capable of outputting scores, or gradients of log density, in a single forward pass. This allows for unrestricted traversal between any initial and final time along the Probability Flow Ordinary Differential Equation (ODE) in a diffusion process.
At its essence, CTM learns the anytime-to-anytime transition along the (deterministic) sampling trajectory. CTM is capable of making "infinitesimal jumps" on the trajectory, enabling the utilization of well-established sampling techniques from diffusion models. Additionally, CTM can make long jumps, which led to the introduction of a novel feature called γ-sampling. With a larger γ, users can generate diverse samples with varying semantic meaning, stimulating creativity and providing more options of generations based on user preferences.
Conversely, a smaller γ maintains semantic coherence in the generated samples. This is especially useful for users intending to use CTM for media restoration, such as removing unwanted audio effects from an audio script, while preserving the semantic integrity of the input audio. Therefore, users can adjust γ for their specific use cases. These flexibilities allow creators to employ the CTM for various purposes without needing to choose between diffusion models or distillation-based models.
Moreover, CTM introduces a new family of sampling schemes, both deterministic and stochastic, which involve long jumps along the ODE solution trajectories. Notably, as computational budgets increase, CTM consistently improves sample quality, avoiding the degradation seen in previous models like CM.
Unlike its predecessors, CTM's access to the score function streamlines the adoption of established controllable and conditional generation methods from the diffusion community. This access also enables the computation of likelihood, further expanding its utility and applicability.
The development of CTM marks a revolutionary milestone in deep generative models. Its innovative approach not only addresses the limitations of existing models but also opens up new possibilities for efficient and high-quality sample generation. With its code readily available, the door is wide open for researchers and practitioners to explore the transformative potential of CTM in various domains.
Unpacking SAN: Enhancing GANs
The Slicing Adversarial Network (SAN) is another transformative innovation by Sony AI that refines Generative Adversarial Networks (GANs) by enhancing the discriminator's ability to measure distances between data distributions. (Authors: Yuhta Takida, Masaaki Imaizumi, Takashi Shibuya, Chieh-Hsin Lai, Toshimitsu Uesaka, Naoki Murata, Yuki Mitsufuji) To help us understand this research, “Imagine you want to reach a destination from a starting point. You can use a map app that guides you to your destination. However, some apps (GANs) often fail to guide us correctly because they misrecognize the distance between us and the destination point. On the other hand, improved apps (SANs) help guide us closer to our target point, enabling more accurate and faithful learning," explains Sony AI researcher, Yuhta Takida. This will help ground us as we explore the methodology and transformative implications of this research.
Methodology
The research uncovered an almost universal method to convert existing GANs into the improved models, called SANs. This conversion process, which we refer to as 'SAN-ify,' can be applied to almost any GAN. “SAN-ification” involves only two steps: modifying a last linear layer of a discriminator and adjusting its objective function. These modifications can be implemented essentially as little as ten lines of code.
SAN modifies existing GAN architectures by integrating principles from sliced optimal transport theory, thereby enabling the discriminator to serve effectively as a measure of distance. This not only improves the fidelity of generated images but also ensures that these images are more diverse and realistic.
Transformative Implications
By applying SAN to platforms like StyleGAN-XL, Sony AI has achieved state-of-the-art results in image generation on benchmarks such as CIFAR10 and ImageNet 256×256. The application of SAN suggests a significant step forward in optimizing GANs, making it a crucial development to serve professionals in creative fields like digital art, audio restoration and animation.
Real-World Implications
Both CTM and SAN stand to revolutionize not just how we create digital content but also how we conceptualize the process of creation itself. CTM, for example, can be likened to a conductor who guides an orchestra through complex symphonies with ease and finesse, ensuring each note resonates perfectly without delay. Similarly, SAN can be seen as refining the palette of a painter, allowing for more distinct and vibrant colors that bring a canvas to life with greater authenticity.
Explore Part 2 of Our SAN Series
Dive deeper into the practicalities of Slicing Adversarial Networks (SAN) by exploring Part 2 of our series—"Implementing SAN: A Hands-On Tutorial." After gaining a theoretical grounding from our blog post, this tutorial offers you a step-by-step guide to enhance your GAN models using SAN. Perfect for developers and researchers eager to apply cutting-edge AI in their work, this follow-up piece provides the practical insights needed to transform theory into action. Don't miss out on elevating your AI toolkit—visit our tutorial here.
Celebrating ICLR 2024 Recognition
The inclusion of CTM and SAN in ICLR 2024 underscores their innovative potential and Sony AI's commitment to pushing the boundaries of what's possible in AI research. This recognition is not just a testament to the technical excellence of these models but also to their practical relevance in today's rapidly evolving digital landscape.
Conclusion
Sony AI's breakthroughs with CTM and SAN are more than just academic achievements; they are beacons of progress in the quest to harness AI's full potential in creative industries. By continuously refining these models, Sony AI enhances the toolkit available to professional creators and broadens the horizons of artistic expression powered by AI.
Latest Blog
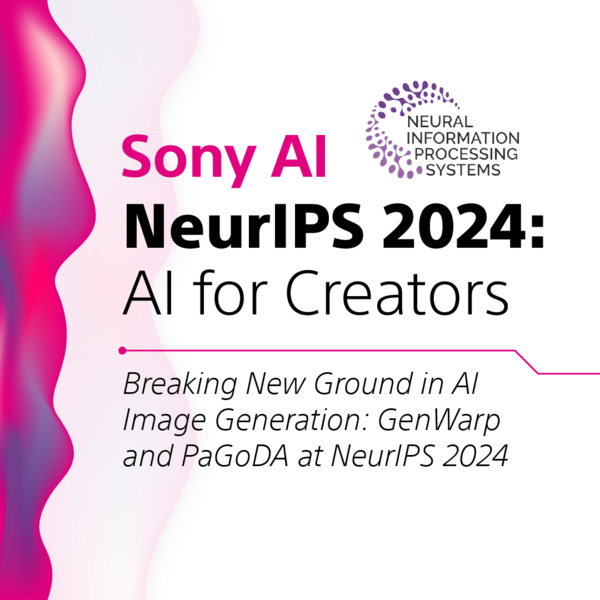
November 15, 2024 | Sony AI
Breaking New Ground in AI Image Generation Research: GenWarp and PaGoDA at NeurI…
At NeurIPS 2024, Sony AI is set to showcase two new research explorations into methods for image generation: GenWarp and PaGoDA. These two research papers highlight advancements in…
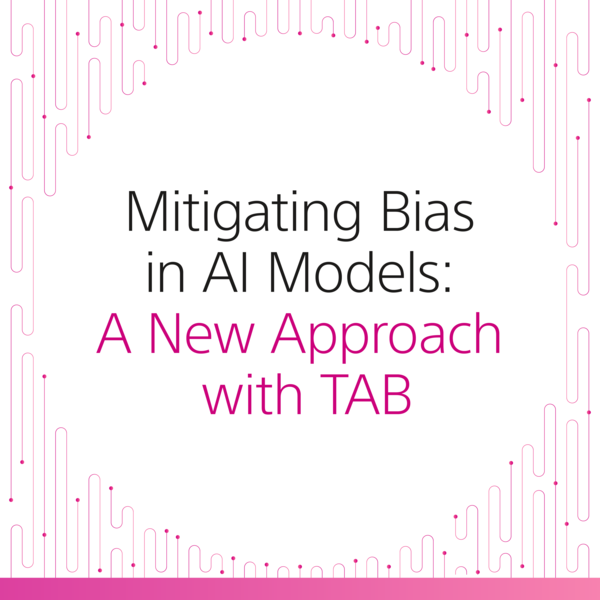
October 4, 2024 | AI Ethics
Mitigating Bias in AI Models: A New Approach with TAB
Artificial intelligence models, especially deep neural networks (DNNs), have proven to be powerful tools in tasks like image recognition and natural language processing. However, t…
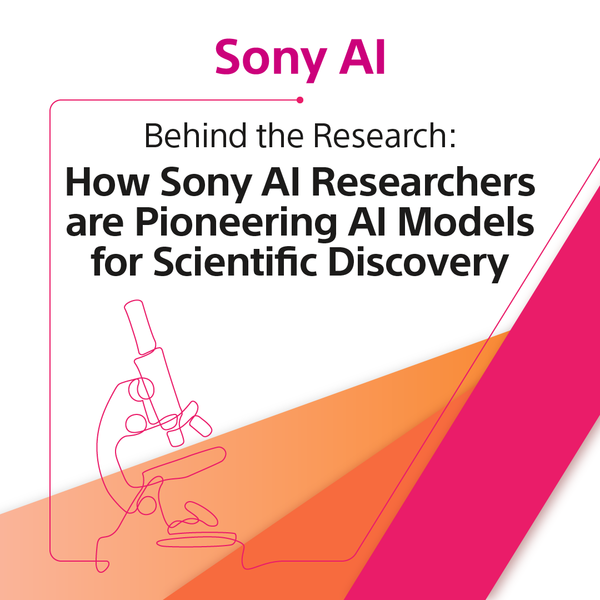
September 14, 2024 | Scientific Discovery
Behind the Research: How Sony AI Researchers are Pioneering AI Models for Scient…
The pace of scientific research is accelerating, with an exponential increase in published research articles each year. For instance, in 1980, approximately 500,000 scientific arti…