Authors
- Borja G. Leon*
- Francesco Riccio
- Kaushik Subramanian
- Pete Wurman
- Peter Stone
* External authors
Date
- 2024
Discovering Creative Behaviors through DUPLEX: Diverse Universal Features for Policy Exploration
Borja G. Leon*
* External authors
2024
Thirty-Eighth Annual Conference on Neural Information Processing Systems (NeurIPS), 2024
†internship project while at Sony AI.
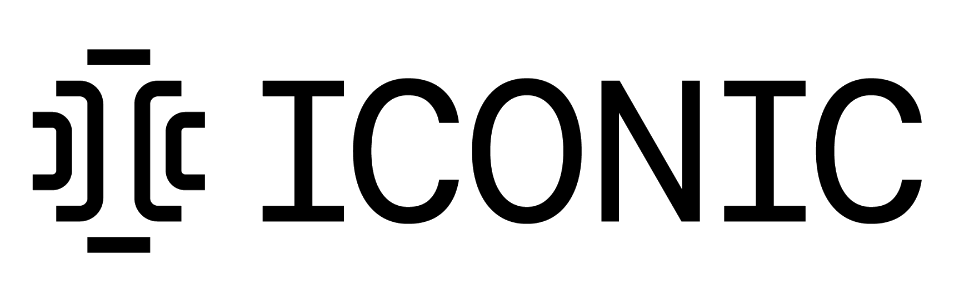
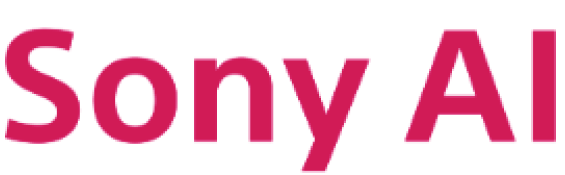
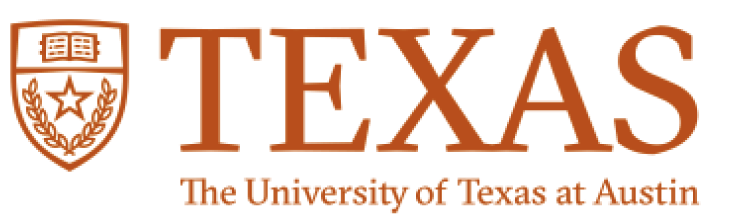
Superhuman and FUN
DUPLEX contributes to diversity learning in RL by improving on previous work to better preserve the diversity vs. near-optimality trade-off in highly-dynamic environments and multi-context settings.
Showing diversity and act differently in the world is fundamental to create engaging experiences for users
Abstract
The ability to approach the same problem from different angles is a cornerstone of human intelligence that leads to robust solutions and effective adaptation to problem variations. In contrast, current RL methodologies tend to lead to policies that settle on a single solution to a given problem, making them brittle to problem variations. Replicating human flexibility in reinforcement learning agents is the challenge that we explore in this work. We tackle this challenge by extending state-of-the-art approaches to introduce DUPLEX, a method that explicitly defines a diversity objective with constraints and makes robust estimates of policies' expected behavior through successor features. The trained agents can (i) learn a diverse set of near-optimal policies in complex highly-dynamic environments and (ii) exhibit competitive and diverse skills in out-of-distribution (OOD) contexts. Empirical results indicate that DUPLEX improves over previous methods and successfully learns competitive driving styles in a hyper-realistic simulator (i.e., GranTurismo™ 7) as well as diverse and effective policies in several multi-context robotics MuJoCo simulations with OOD gravity forces and height limits. To the best of our knowledge, our method is the first to achieve diverse solutions in complex driving simulators and OOD robotic contexts.
DUPLEX
Data flow
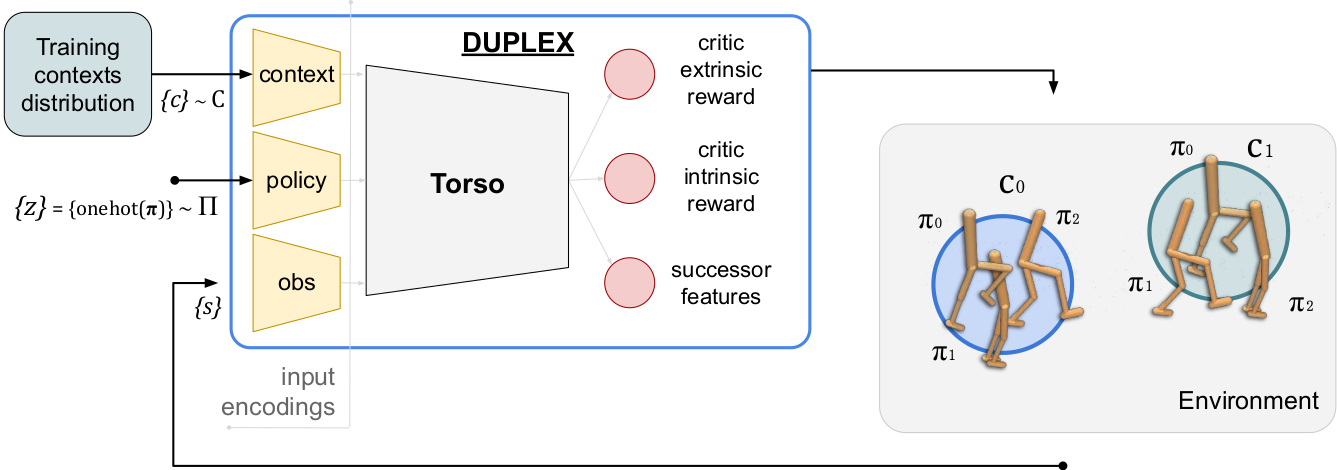
DUPLEX is designed to receive three inputs: (i) a context vector describing task requirements and environment dynamics in the current episode \(c\); (ii) an encoding of the policy used in the episode \(z\); (iii) and the current state of the environment \(s\). The critic network returns estimates for the intrinsic and extrinsic rewards and successor features to drive diverse behavior discovery. Finally, the algorithm samples policies in \(\Pi\) uniformly and rolls them out to collect more experience.
Context-conditioned diversity learning
DUPLEX's diversity definition
Diversity(\(\Pi\)) is a metric of dissimilarity among policies in a set \(\Pi\) with a common goal. Formally, if \(\psi_{\pi_i}\) and \(\psi_{\pi_j}\) are a function of state-occupancy of relevant features of any two policies in \(\Pi\), then their dissimilarity is given by the norm \(\lVert \psi_{\pi_i} - \psi_{\pi_j} \rVert_2\). A non-zero value of this norm indicates dissimilarity, with larger values indicating greater divergence between the policies. Mathematically, diversity is defined as the sum of the minimum L2 dissimilarity norms in:
$$ \operatorname{Diversity}(\Pi) = \frac{1}{2 \cdot \text{size}(\Pi)} \cdot \sum_{\substack{\forall \pi_i,\pi_j \in \Pi,\\ i \neq j}} \min \lVert \psi_{\pi_i} - \psi_{\pi_j} \rVert_2^2 $$
Accordingly, we measure \(\psi\) distances to enforce context-conditioned diversity within \(\Pi\). We aim at training an RL agent that, given a context \(c\), discovers a set of \(n\) near-optimal policies by optimizing our objective function:
$$ \max_{\Pi} \; \operatorname{Diversity}(\Pi) \text{ s.t. } d_{\pi_c} \cdot r_e \geq \rho \hat{v}_e, \quad \forall \pi_c \in \Pi $$
that forces the policies in \(\Pi\) to only explore for diversity within the near-optimal region of the target value.
Duplex stabilizes training and achieves diverse competitive policies by introducing novel components to modulate the contribution of the intrinsics reward:
$$ r_I = \lambda \cdot \chi \cdot r_d $$
Dynamic intrinsic reward factor:
$$ \chi_t = \alpha_{\chi} \chi_t^\prime + (1-\alpha_{\chi})\chi_{(t-1)} $$
$$ \chi^\prime = \left| \frac{v_{e_{\text{avg}}}}{v_{d_{\text{avg}}}} \right| (1-\rho) $$
\(\chi\) scales intrinsic rewards proportionally to the sum of extrinsic values of policies in \(\Pi\).
Soft-lower bound:
$$ \lambda = \left\{\sigma_k\left(\frac{v_{e_{\text{avg}}}^{i}-\beta \hat{v}_{e_{\text{avg}}}}{|\hat{v}_{e_{\text{avg}}}+l|}\right)\right\}_{i=1}^n $$
\(\lambda\) bounds the near-optimal subspace for each policy, where \(\sigma_k\) is a sigmoid function and \(\beta \in [0, 1]\) indicates the reward region we are interested in exploring.
Adding entropy to the SFs estimation:
$$ \psi^{\gamma,i}(s_t, a_t, c)= \phi_t + \mathbb{E}_{\pi_c}\sum_{k=t+1}^{\infty}\gamma^{k-t} \big[\phi_k + \alpha_H H\big(\pi_c^i(\cdot|s,c)\big)\big] $$
to support critic estimates when policy is uncertain.
Averaging critic networks:
$$ y(\phi,s', c, z) =\phi(t)+\gamma\left(\underset{j=1,2}{\operatorname{avg}}\tilde{\psi}_{\theta_{\text{targ},j}}(s',\tilde{a}'_z, c)-\alpha\log\pi^z_{\omega}(\tilde{a}'_z|s',c)\right) $$
Results
Here we show that DUPLEX is the first successful method learning diverse driving styles in highly-dynamic physics simulators; yields a better performance vs. diversity trade-off in canonical physics simulators; exhibits diverse and effective behaviors in OOD dynamics and tasks
GranTurismo™ 7
DUPLEX trains diverse competitive policies in hyper-realistic driving simulators
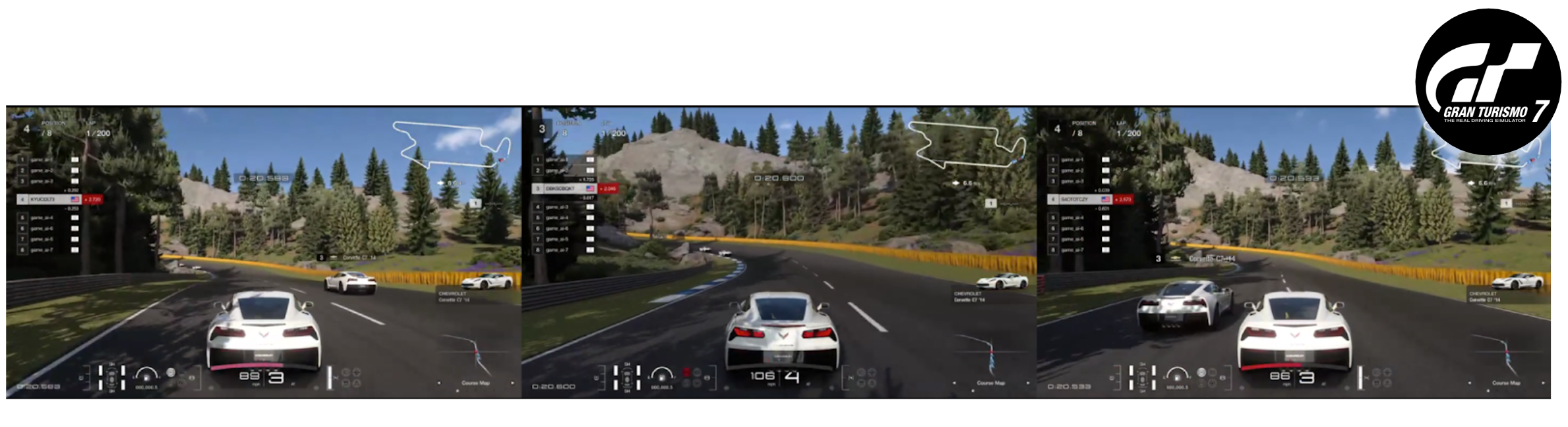
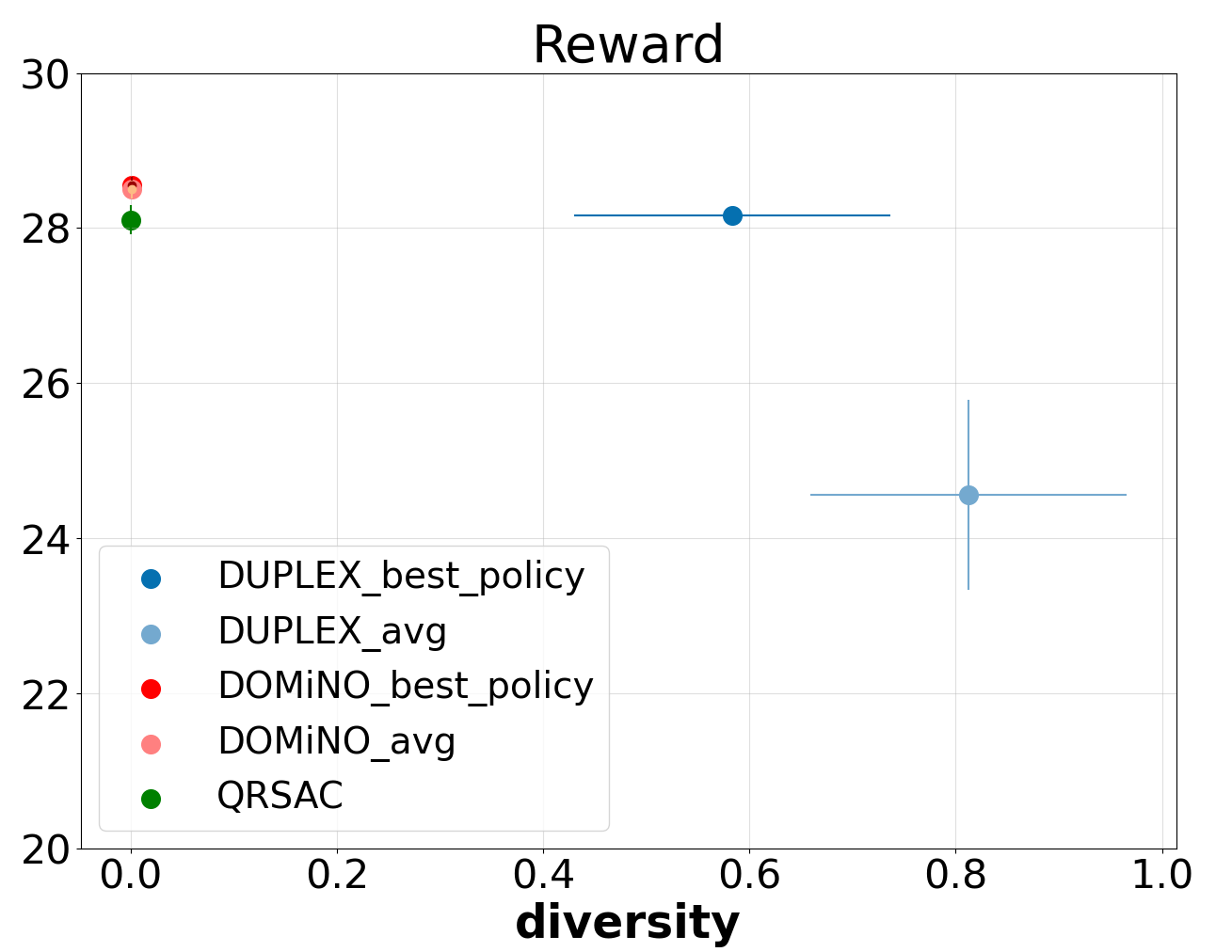
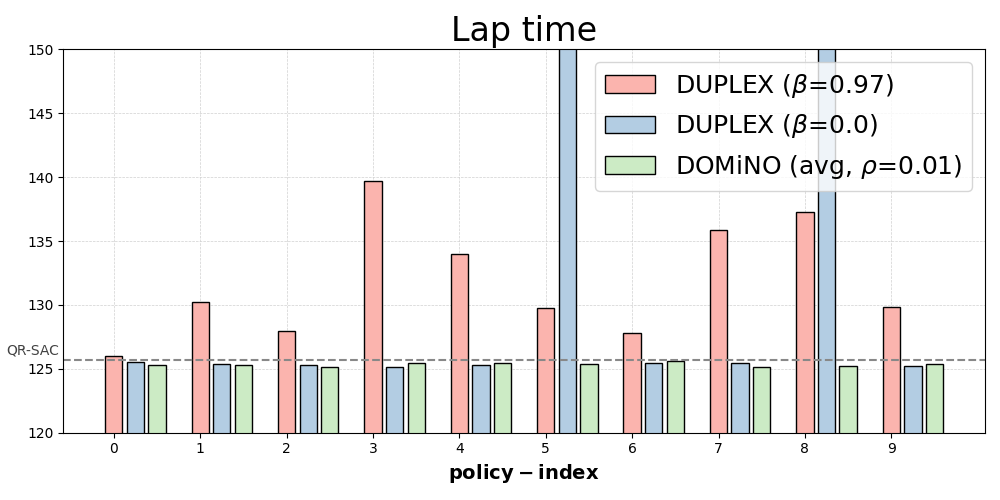
Near-optimal diversity
In GranTurismo7 (GT), we observe that policies manage to score similar lap-times while being
different. In particular,
the DUPLEX agent converges to diverse skill in using the brake and overtaking to guarantee
diversity. To highlight some
examples, at mark 00:10 Policy-2 showcases a different driving line while overtaking the pack of
cars in front.
Moreover, at mark 01:34 we observe that Policy-0 and Policy-1 also employ different strategies while
facing similar
situations. In fact, Policy-1 performs simple overtaks exploiting the slipstream, while Policy-0
executes a slingshot
pass.
Relaxing the optimality constraint to foster diversity
Once the optimality constraint becomes more forgiving, we observer that the DUPLEX agent explores different driving styles that also affect the cars lap-times. For example, we observe different driving lines and different braking areas when approaching a turn. Moreover, driving style become more creative since the agent is allowed to compromise optimality, and thus, we see Policy-4 (bottom-right) discovering a drifting behavior to complete turns (mark 1:00 onwards).
Discovering diversity on specific successor features: Aggressiveness
Here, we report an interesting result that showcases how DUPLEX can be used to target diversity on particular Successor Features, and thus, to make strategic behavior (that might be difficult implement in a reward function) emerge. For example, in order to provide diversity on the aggressiveness levels of the agent, we can simply use the observation on how often cars are being hit by the agent. This translates in various policy strategies that range from very timid and cautions agents to very aggressive ones that intentionally hit other cars (if the collision is not expected to result in significant speed loss)
MuJoCo Walker2d and Ant
DUPLEX improves the diversity vs near-optimality trade-off both within- and out-of- distribution settings
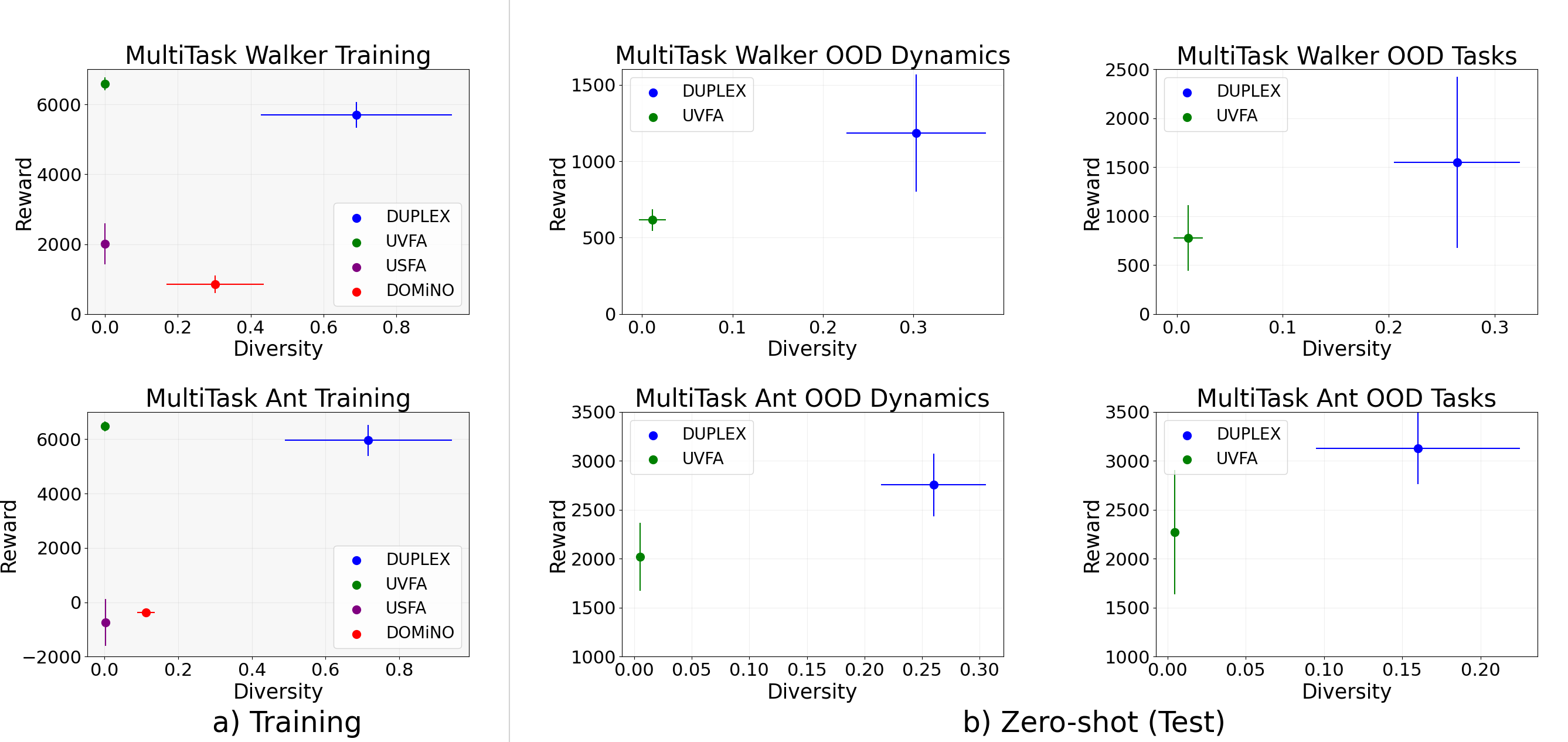
Experiment Ant: Jump with low gravity
We evaluate Duplex in an out-of-distribution (OOD) multi-context MuJoCo.Ant scenario. Here the agent is tasked to jump forward while operating under a gravitational force 46% lower than the minimum gravity included in the in training scenarios. In such context, Policy-0 uses three legs while jumping – resulting in the slowest policy completing the task. Differently, Policy-1 executes rotating jumps, while Policy-2 converged to the the most effective behavior by using the front two legs to propel in the air.
Experiment Ant: Walk forward with high gravity
In this experiment, we evaluate a DUPLEX agent in another OOD multi-context MuJoCo.Ant scenario. Akin to the previous case, the agent is tasked to move forward as fast as possible but now it is forced to move under a gravitational force that is 40% higher than the maximum gravity considered at training time. We observe that agent diversifies the usage of legs influencing the walking behavior of the policies. In particular, two policies exploit two out of four legs: Policy-0 uses legs 2 and 4 while Policy-1 uses legs 1 and 3 respectively. Policy-2 instead, learns to use legs 1, 2 and 3 while limping on leg 4 occasionally.
Experiment Walker: Jump while moving forward with low gravity
Similarly to the Ant scenario, we evaluate Duplex while operating OOD with a different agent while operating under a gravitational force 46% lower than the minimum gravity in the training set. In this scenario, all policies can successfully move forward and jump without falling while diversifying the legs openings and postures in the air. In particular, we observe that from left to right videoclip, the policies adopt diverse strategies widening more and more their legs angle while raising the knees incrementally higher.
Experiment Walker: Walk with stronger gravity and with a spatial constraint
In this experiment we evaluate DUPLEX while operating in OOD dynamics while being constrained to walk below an “invisible” horizontal. Specifically, the agent operates with a gravitational force that is 46% higher that the ones in the training set and with a wall height that is 20% lower than the minimum height experienced at training time. That invisible wall forces all policies to bend the head. This is the most challenging task limits exploration for diversity since the agent has to guarantee task execution with all the policies. Nevertheless, we observe that both Policy-0 and Policy-1 periodically use little jumps to keep moving forward and that Policy-1 widens the agent legs more than Policy-0. Policy-2 instead manages to make actual jumps and still move forward.
BibTeX
@InProceedings{NEURIPS24-duplex,
author="Borja G. Le\'on and Francesco Riccio and Kaushik Subramanian and Peter R. Wurman and Peter Stone",
title="Discovering Creative Behaviors through DUPLEX: Diverse Universal Features for Policy Exploration",
booktitle="NeurIPS 2024, the Thirty-Eighth Annual Conference on Neural Information Processing Systems",
month="December",
year="2024",
location="Vancouver, Canada",
abstract={The ability to approach the same problem from different angles is a cornerstone of human intelligence that leads to robust solutions and effective adaptation to problem variations. In contrast, current RL methodologies tend to lead to policies that settle on a single solution to a given problem, making them brittle to problem variations. Replicating human flexibility in reinforcement learning agents is the challenge that we explore in this work. We tackle this challenge by extending state-of-the-art approaches to introduce \duplex, a method that explicitly defines a diversity objective with constraints and makes robust estimates of policies' expected behavior through successor features. The trained agents can (i) learn a diverse set of near-optimal policies in complex highly-dynamic environments and (ii) exhibit competitive and diverse skills in out-of-distribution (OOD) contexts. Empirical results indicate that \duplex~improves over previous methods and successfully learns competitive driving styles in a hyper-realistic simulator (i.e., GranTurismo\textsuperscript
{\texttrademark} 7) as well as diverse and effective policies in several multi-context robotics MuJoCo simulations with OOD gravity forces and height limits. To the best of our knowledge, our method is the first to achieve diverse solutions in complex driving simulators and OOD robotic contexts.
}
},
Related Publications
Current approaches to learning cooperative multi-agent behaviors assume relatively restrictive settings. In standard fully cooperative multi-agent reinforcement learning, the learning algorithm controls all agents in the scenario, while in ad hoc teamwork, the learning algor…
Racing autonomous cars faster than the best human drivers has been a longstanding grand challenge for the fields of Artificial Intelligence and robotics. Recently, an end-to-end deep reinforcement learning agent met this challenge in a high-fidelity racing simulator, Gran Tu…
Autonomous mobility tasks such as lastmile delivery require reasoning about operator indicated preferences over terrains on which the robot should navigate to ensure both robot safety and mission success. However, coping with out of distribution data from novel terrains or a…
JOIN US
Shape the Future of AI with Sony AI
We want to hear from those of you who have a strong desire
to shape the future of AI.