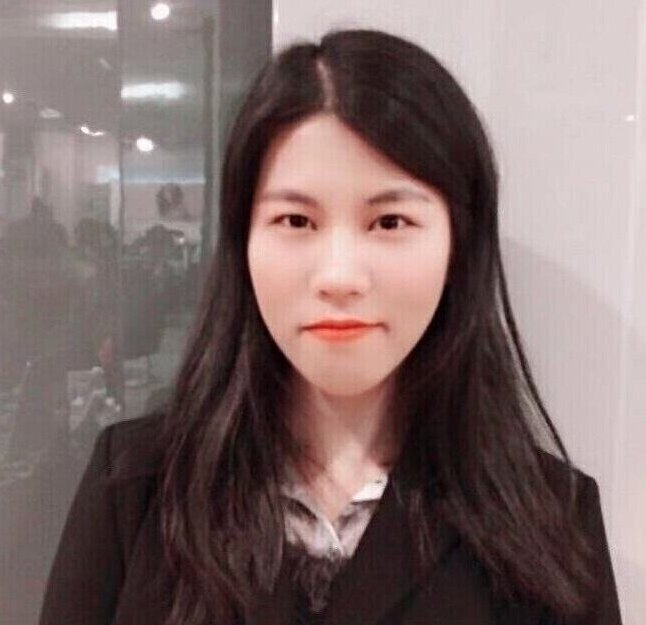
Lingjuan
Lyu
Profile
Lingjuan is the Head of Privacy-Preserving Machine Learning (PPML) team in Sony AI. As a globally recognized expert in privacy and security, she is leading a group of excellent scientists and engineers on privacy and security related initiatives across the company. Prior to joining Sony AI, she spent more than eight years working in academia and at industry organizations. Lingjuan received her Ph.D. from the University of Melbourne. She was a recipient of the prestigious IBM PhD Fellowship Award Worldwide. Lingjuan’s current interest is trustworthy AI, mainly on federated learning, responsible foundation model development, data privacy, model robustness, IP protection, on-device AI, etc. She had published over 100 papers in top conferences and journals, including NeurIPS, ICML, ICLR, Nature, etc. She and her papers had won a long list of awards from top main venues, such as ICML Outstanding Paper Award, ACL Area Chair Award, CIKM Best Paper Runner-up Award (only 1), IEEE Outstanding Leadership Award, and many best paper awards from AAAI, IJCAI, WWW, KDD, etc.
Message
“The Sony AI Privacy-Preserving Machine Learning (PPML) team conducts cutting-edge research on trustworthy AI. Our team aims to integrate more privacy-preserving and robust AI solutions across Sony products. In the long-term, I hope that we can make the industrial AI systems privacy-compliant and robust for social good.”
Publications
Current image anonymization techniques, largely focus on localized pseudonymization, typically modify identifiable features like faces or full bodies and evaluate anonymity through metrics such as detection and re-identification rates. However, this approach often overlooks …
We present COALA, a vision-centric Federated Learning (FL) platform, and a suite of benchmarks for practical FL scenarios, which we categorize as task, data, and model levels. At the task level, COALA extends support from simple classification to 15 computer vision tasks, in…
Latent generative models (e.g., Stable Diffusion) have become more and more popular, but concerns have arisen regarding potential misuse related to images generated by these models. It is, therefore, necessary to analyze the origin of images by inferring if a particular imag…
Federated learning (FL) promotes decentralized training while prioritizing data confidentiality. However, its application on resource-constrained devices is challenging due to the high demand for computation and memory resources for training deep learning models. Neural netw…
Recent text-to-image diffusion models have shown surprising performance in generating high-quality images. However, concerns have arisen regarding the unauthorized data usage during the training or fine-tuning process. One example is when a model trainer collects a set of im…
Federated learning (FL) enhances data privacy with collaborative in-situ training on decentralized clients. Nevertheless, FL encounters challenges due to non-independent and identically distributed (non-i.i.d) data, leading to potential performance degradation and hindered c…
Recent breakthroughs in diffusion models have exhibited exceptional image-generation capabilities. However, studies show that some outputs are merely replications of training data. Such replications present potential legal challenges for model owners, especially when the gen…
The interest in federated learning has surged in recent research due to its unique ability to train a global model using privacy-secured information held locally on each client. This paper pays particular attention to the issue of client-side model heterogeneity, a pervasive…
Hand-crafted image quality metrics, such as PSNR and SSIM, are commonly used to evaluate model privacy risk under reconstruction attacks. Under these metrics, reconstructed images that are determined to resemble the original one generally indicate more privacy leakage. Image…
With growing concerns regarding privacy in machine learning models, regulations have committed to granting individuals the right to be forgotten while mandating companies to develop non-discriminatory machine learning systems, thereby fueling the study of the machine unlearn…
This paper focuses on addressing the practical yet challenging problem of model heterogeneity in federated learning, where clients possess models with different network structures. To track this problem, we propose a novel framework called pFedHR, which leverages heterogeneo…
Federated learning (FL) is an effective machine learning paradigm where multiple clients can train models based on heterogeneous data in a decentralized manner without accessing their private data. However, existing FL systems undergo performance deterioration due to feature…
Image generation techniques have been gaining increasing attention recently, but concerns have been raised about the potential misuse and intellectual property (IP) infringement associated with image generation models. It is, therefore, necessary to analyze the origin of ima…
Federated learning (FL) is an emerging distributed machine learning method that empowers in-situ model training on decentralized edge devices. However, multiple simultaneous FL tasks could overload resource-constrained devices. In this work, we propose the first FL system to…
Semi-supervised machine learning (SSL) is gaining popularity as it reduces the cost of training ML models. It does so by using very small amounts of (expensive, well-inspected) labeled data and large amounts of (cheap, non-inspected) unlabeled data. SSL has shown comparable …
This paper focuses on an under-explored yet important problem: Federated Class-Continual Learning (FCCL), where new classes are dynamically added in federated learning. Existing FCCL works suffer from various limitations, such as requiring additional datasets or storing the …
AI Generated Content (AIGC) has received tremendous attention within the past few years, with content ranging from image, text, to audio, video, etc. Meanwhile, AIGC has become a double-edged sword and recently received much criticism regarding its responsible usage. In this…
Source-Free domain adaptation transits the source-trained model towards target domain without exposing the source data, trying to dispel these concerns about data privacy and security. However, this paradigm is still at risk of data leakage due to adversarial attacks on the …
Federated learning (FL) is an important technique for learning models from decentralized data in a privacy-preserving way. Existing FL methods usually uniformly sample clients for local model learning in each round. However, different clients may have significantly different…
The EU AI Act proposal addresses, among other applications, AI systems that enable facial classification and emotion recognition. As part of previous work, we have investigated how citizens deliberate about the validity of AI-based facial classifications in the advertisement…
External data sources are increasingly being used to train machine learning (ML) models as the data demand increases. However, the integration of external data into training poses data poisoning risks, where malicious providers manipulate their data to compromise the utility…
Collecting and training over sensitive personal data raise severe privacy concerns in personalized recommendation systems, and federated learning can potentially alleviate the problem by training models over decentralized user data.However, a theoretically private solution i…
Federated learning has exhibited vulnerabilities to Byzantine attacks, where the Byzantine attackers can send arbitrary gradients to the central server to destroy the convergence and performance of the global model. A wealth of defenses have been proposed to defend against B…
Data-free knowledge distillation (KD) helps realistically transfer knowledge from a pre-trained model (known as the teacher model) to a smaller model (known as the student model) without access to the original training data used for training the teacher model. However, the s…
Federated machine unlearning (FMU) aims to remove the influence of a specified subset of training data upon request from a trained federated learning model. Despite achieving remarkable performance, existing FMU techniques suffer from inefficiency due to two sequential opera…
Deep neural networks (DNNs) have been found to be vulnerable to backdoor attacks, raising security concerns about their deployment in mission-critical applications. While existing defense methods have demonstrated promising results, it is still not clear how to effectively r…
Large language models (LLMs) have demonstrated powerful capabilities in both text understanding and generation. Companies have begun to offer Embedding as a Service (EaaS) based on these LLMs, which can benefit various natural language processing (NLP) tasks for customers. H…
Recent studies show that models trained by continual learning can achieve the comparable performances as the standard supervised learning and the learning flexibility of continual learning models enables their wide applications in the real world. Deep learning models, howeve…
Existing collaborative self-supervised learning (SSL) schemes are not suitable for cross-client applications because of their expensive computation and large local data requirements. To address these issues, we propose MocoSFL, a collaborative SSL framework based on Split Fe…
Knowledge Distillation (KD) is a typical method for training a lightweight student model with the help of a well-trained teacher model. However, most KD methods require access to either the teacher's training data or model parameter, which is unrealistic. To tackle this prob…
In real-world applications, deep learning models often run in non-stationary environments where the target data distribution continually shifts over time. There have been numerous domain adaptation (DA) methods in both online and offline modes to improve cross-domain adaptat…
Continual Test-time Adaptation (CTA) is a promising art to secure accuracy gains in continually-changing environments. The state-of-the-art adaptations improve out-of-distribution model accuracy via computation-efficient online test-time gradient descents but meanwhile cost …
In this paper, we investigate the problem of certifying neural network robustness against universal perturbations (UPs), which have been widely used in universal adversarial attacks and backdoor attacks. Existing robustness certification methods aim to provide robustness gua…
With the great popularity of Graph Neural Networks (GNNs), their robustness to adversarial topology attacks has received increasing attention. Although many attack methods have been proposed, they mainly focus on fixed-budget attacks, aiming at finding the most adversarial p…
In Federated Learning (FL), models are as fragile as centrally trained models against adversarial examples. However, the adversarial robustness of federated learning remains largely unexplored. This paper casts light on the challenge of adversarial robustness of federated le…
The frustratingly fragile nature of neural network models make current natural language generation (NLG) systems prone to backdoor attacks and generate malicious sequences that could be sexist or offensive. Unfortunately, little effort has been invested to how backdoor attac…
Existing collaborative self-supervised learning (SSL) schemes are not suitable for cross-client applications because of their expensive computation and large local data requirements. To address these issues, we propose MocoSFL, a collaborative SSL framework based on Split Fe…
As deep learning blooms with growing demand for computation and data resources, outsourcing model training to a powerful cloud server becomes an attractive alternative to training at a low-power and cost-effective end device. Traditional outsourcing requires uploading device…
Recent studies have shown that, like traditional machine learning, federated learning (FL) is also vulnerable to adversarial attacks.To improve the adversarial robustness of FL, few federated adversarial training (FAT) methods have been proposed to apply adversarial training…
One-shot Federated Learning (FL) has recently emerged as a promising approach, which allows the central server to learn a model in a single communication round. Despite the low communication cost, existing one-shot FL methods are mostly impractical or face inherent limitatio…
Previous works have validated that text generation APIs can be stolen through imitation attacks, causing IP violations. In order to protect the IP of text generation APIs, a recent work has introduced a watermarking algorithm and utilized the null-hypothesis test as a post-h…
The right to be forgotten calls for efficient machine unlearning techniques that make trained machine learning models forget a cohort of data. The combination of training and unlearning operations in traditional machine unlearning methods often leads to the expensive computa…
Vertical federated learning (VFL) is a privacy-preserving machine learning paradigm that can learn models from features distributed on different platforms in a privacy-preserving way. Since in real-world applications the data may contain bias on fairness-sensitive features (…
The statistical heterogeneity of the non-independent and identically distributed (non-IID) data in local clients significantly limits the performance of federated learning. Previous attempts like FedProx, SCAFFOLD, MOON, FedNova and FedDyn resort to an optimization perspecti…
As data are increasingly being stored in different silos and societies becoming more aware of data privacy issues, the traditional centralized training of artificial intelligence (AI) models are facing efficiency and privacy challenges. Recently, federated learning (FL) has …
Integrating multiple online social networks (OSNs) has important implications for many downstream social mining tasks, such as user preference modelling, recommendation, and link prediction. However, it is unfortunately accompanied by growing privacy concerns about leaking s…
Machine-learning-as-a-service (MLaaS) has attracted millions of users to their splendid large-scale models. Although published as black-box APIs, the valuable models behind these services are still vulnerable to imitation attacks. Recently, a series of works have demonstrate…
Deep Neural Networks (DNNs) are known to be vulnerable to backdoor attacks. In Natural Language Processing (NLP), DNNs are often backdoored during the fine-tuning process of a large-scale Pre-trained Language Model (PLM) with poisoned samples. Although the clean weights of P…
The collection and availability of big data, combined with advances in pre-trained models (e.g. BERT), have revolutionized the predictive performance of natural language processing tasks. This allows corporations to provide machine learning as a service (MLaaS) by encapsulat…
To prevent unintentional data leakage, research community has resorted to data generators that can produce differentially private data for model training. However, for the sake of the data privacy, existing solutions suffer from either expensive training cost or poor general…
The federated learning (FL) framework enables edge clients to collaboratively learn a shared inference model while keeping privacy of training data on clients. Recently, many heuristics efforts have been made to generalize centralized adaptive optimization methods, such as S…
Graph neural network (GNN) is effective in modeling high-order interactions and has been widely used in various personalized applications such as recommendation. However, mainstream personalization methods rely on centralized GNN learning on global graphs, which have conside…
In recent years, knowledge graph~(KG) has obtained many achievements in both research and industrial fields. However, most KG algorithms consider node embedding with only structure and node features, but not relation features. In this paper, we propose a novel Heterogeneous …
Since training a large-scale backdoored model from scratch requires a large training dataset, several recent attacks have considered to inject backdoors into a trained clean model without altering model behaviors on the clean data. Previous work finds that backdoors can be i…
Graph Neural Network (GNN) has achieved remarkable progresses in various real-world tasks on graph data. High-performance GNN models always depend on both rich features and complete edge information in graph. However, such information could possibly be isolated by different …
Graph neural networks (GNNs) have been widely used in modeling graph structured data, owing to its impressive performance in a wide range of practical applications. Recently, knowledge distillation (KD) for GNNs has enabled remarkable progress in graph model compression and …
Adversarial training (AT) is a typical method to learn adversarially robust deep neural networks via training on the adversarial variants generated by their natural examples. However, as training progresses, the training data becomes less attackable, which may undermine the …
Federated learning is a privacy-preserving machine learning technique to train intelligent models from decentralized data, which enables exploiting private data by communicating local model updates in each iteration of model learning rather than the raw data. However, model …
Existing federated learning (FL) designs have been shown to exhibit vulnerabilities which can be exploited by adversaries to compromise data privacy. However, most current works conduct attacks by leveraging gradients calculated on a small batch of data. This setting is not …
Accurate traffic anomaly prediction offers an opportunity to save the wounded at the right location in time. However, the complex process of traffic anomaly is affected by both various static factors and dynamic interactions. The recent evolving representation learning provi…
Native ad is a popular type of online advertisement which has similar forms with the native content displayed on websites. Native ad CTR prediction is useful for improving user experience and platform revenue. However, it is challenging due to the lack of explicit user inten…
The fast growth of pre-trained models (PTMs) has brought natural language processing to a new era, which becomes a dominant technique for various natural language processing (NLP) applications. Every user can download weights of PTMs, then fine-tune the weights on a task on …
Federated learning (FL) provides a privacy-aware learning framework by enabling a multitude of participants to jointly construct models without collecting their private training data. However, federated learning has exhibited vulnerabilities to Byzantine attacks. Many existi…
Previous studies have shown that federated learning (FL) is vulnerable to well-crafted adversarial examples. Some recent efforts tried to combine adversarial training with FL, i.e., federated adversarial training (FAT), in order to achieve adversarial robustness in FL. Howev…
Cross Domain Recommendation (CDR) has been popularly studied to alleviate the cold-start and data sparsity problem commonly existed in recommender systems. CDR models can improve the recommendation performance of a target domain by leveraging the data of other source domains…
Visual surveillance technology is an indispensable functional component of advanced traffic management systems. It has been applied to perform traffic supervision tasks, such as object detection, tracking and recognition. However, adverse weather conditions, e.g., fog, haze …
Nowadays, due to the breakthrough in natural language generation (NLG), including machine translation, document summarization, image captioning, etc NLG models have been encapsulated in cloud APIs to serve over half a billion people worldwide and process over one hundred bil…
Social recommendation has shown promising improvements over traditional systems since it leverages social correlation data as an additional input. Most existing works assume that all data are available to the recommendation platform. However, in practice, user-item interacti…
Backdoor attack has emerged as a major security threat to deep neural networks(DNNs). While existing defense methods have demonstrated promising results on detecting and erasing backdoor triggers, it is still not clear if measures can be taken to avoid the triggers from bein…
Collaborative machine learning provides a promising framework for different agents to pool their resources (e.g., data) for a common learning task. In realistic settings where agents are self-interested and not altruistic, they may be unwilling to share data or model without…
Federated machine learning which enables resource-constrained node devices (e.g., Internet of Things (IoT) devices, smartphones) to establish a knowledge-shared model while keeping the raw data local, could provide privacy preservation and economic benefit by designing an ef…
Recently, large volumes of false or unverified information (e.g., fake news and rumors) appear frequently in emerging social media, which are often discussed on a large scale and widely disseminated, causing bad consequences. Many studies on rumor detection indicate that the…
Federated learning (FL) emerged as a promising learning paradigm to enable a multitude of partici- pants to construct a joint ML model without expos- ing their private training data. Existing FL designs have been shown to exhibit vulnerabilities which can be exploited by adv…
Blog
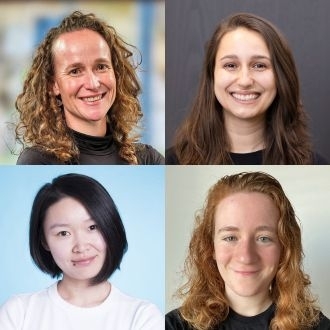
March 29, 2024 | Life at Sony AI
Celebrating the Women of Sony AI: Sharing Insights, Inspiration, and Advice
In March, the world commemorates the accomplishments of women throughout history and celebrates those of today. The United States observes March as Women’s History Month, while many countries around the globe observe International…
In March, the world commemorates the accomplishments of women throughout history and celebrates those of today. The United States …
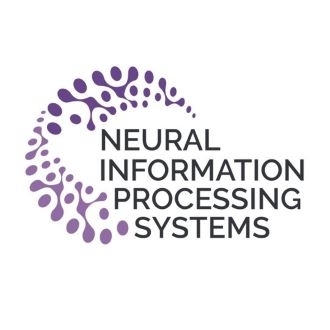
December 13, 2023 | Events
Sony AI Reveals New Research Contributions at NeurIPS 2023
Sony Group Corporation and Sony AI have been active participants in the annual NeurIPS Conference for years, contributing pivotal research that has helped to propel the fields of artificial intelligence and machine learning forwar…
Sony Group Corporation and Sony AI have been active participants in the annual NeurIPS Conference for years, contributing pivotal …
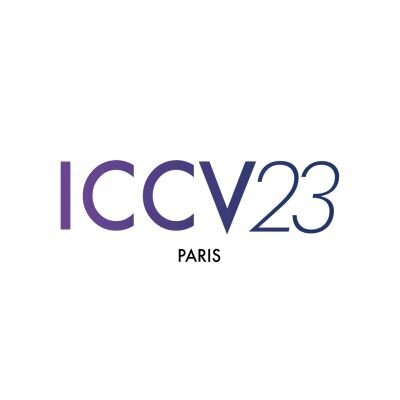
October 6, 2023 | PPML
Advancements in Federating Learning Highlighted in Papers Presented at ICCV 2023
As the field of machine learning continues to evolve, Sony AI researchers are constantly exploring innovative solutions to address the pressing issues faced by the industry. Two research papers, both accepted at the premier intern…
As the field of machine learning continues to evolve, Sony AI researchers are constantly exploring innovative solutions to address…
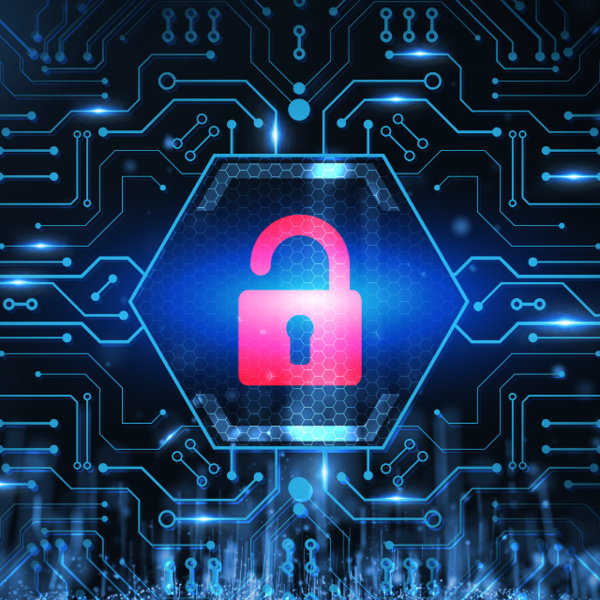
August 7, 2023 | Machine Learning
Privacy-Preserving Machine Learning Blog Series: Practicing Privacy by Design
Privacy-Preserving Machine Learning Blog SeriesAt Sony AI, the Privacy-Preserving Machine Learning (PPML) team focuses on fundamental and applied research in computer vision privacy. Their innovative research aims to apply these n…
Privacy-Preserving Machine Learning Blog SeriesAt Sony AI, the Privacy-Preserving Machine Learning (PPML) team focuses on fundamen…
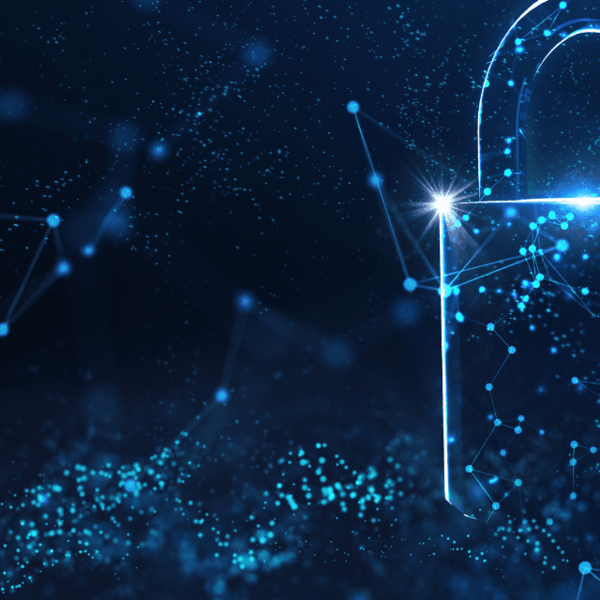
June 8, 2023 | Machine Learning
Recent Breakthroughs Tackle Challenges in Federated Learning
Privacy-Preserving Machine Learning Blog SeriesAt Sony AI, the Privacy-Preserving Machine Learning (PPML) team focuses on fundamental and applied research in computer vision privacy and security. Their innovative research aims to …
Privacy-Preserving Machine Learning Blog SeriesAt Sony AI, the Privacy-Preserving Machine Learning (PPML) team focuses on fundamen…
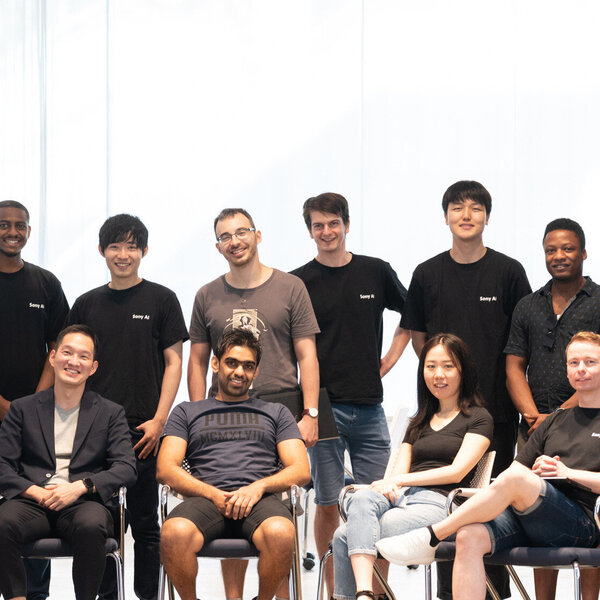
November 29, 2021 | Life at Sony AI
Meet the Team #2: Lingjuan, Jerone and Roberto
What do privacy, pattern recognition, and percussion all have in common? They are concepts and creative endeavors that have inspired Sony AI team members Lingjuan, Jerone and Roberto. Read on to learn more about these three Sony…
What do privacy, pattern recognition, and percussion all have in common? They are concepts and creative endeavors that have insp…
JOIN US
Shape the Future of AI with Sony AI
We want to hear from those of you who have a strong desire
to shape the future of AI.